Understanding the quantum supremacy debate in the context of AI
Understanding the quantum supremacy debate in the context of AI is crucial for grasping the future of artificial intelligence. Quantum computing, with its potential to solve problems intractable for even the most powerful classical computers, promises a revolution in AI. But the path is fraught with challenges: building stable and scalable quantum computers, developing efficient quantum algorithms, and even defining what constitutes “quantum supremacy” are all areas of intense debate.
This exploration delves into these complexities, examining the current state of the field, the controversies surrounding its progress, and the profound implications for various sectors.
From drug discovery to financial modeling, the potential applications of quantum AI are vast. However, significant hurdles remain before we see widespread adoption. This exploration aims to clarify the key issues, present the ongoing discussion in an accessible way, and provide a balanced perspective on the opportunities and risks associated with this transformative technology.
Defining Quantum Supremacy and its Relevance to AI
Quantum supremacy, in simple terms, is the point where a quantum computer can solve a problem that’s practically impossible for even the most powerful classical computers. It’s not about being “better” at everything, but about demonstrating a clear advantage in specific, computationally intensive tasks. This has significant implications for artificial intelligence, potentially unlocking new possibilities in areas currently limited by classical computing power.Quantum computers leverage the bizarre laws of quantum mechanics to perform calculations in fundamentally different ways than classical computers.
Instead of bits representing 0 or 1, they use qubits, which can exist in a superposition – representing both 0 and 1 simultaneously. This, along with other quantum phenomena like entanglement, allows for vastly more complex calculations to be performed, potentially leading to exponential speedups for certain types of problems.
Potential Advantages of Quantum Computers for AI Tasks
The potential advantages of quantum computers for AI are numerous. Quantum algorithms can dramatically accelerate machine learning processes, leading to faster training times and the ability to handle significantly larger datasets. This could revolutionize fields like drug discovery, materials science, and financial modeling, where complex AI models are crucial. For example, quantum machine learning could lead to the development of more accurate and efficient algorithms for image recognition, natural language processing, and anomaly detection.
Faster training also means that more complex and sophisticated AI models can be developed and deployed.
Comparison of Classical and Quantum Algorithms for Machine Learning
Classical machine learning algorithms rely on iterative processes to find optimal solutions. While powerful, they often struggle with high-dimensional data and complex relationships. Quantum algorithms, on the other hand, can exploit quantum phenomena like superposition and entanglement to explore the solution space more efficiently. For instance, quantum algorithms like Quantum Approximate Optimization Algorithm (QAOA) and Variational Quantum Eigensolver (VQE) are designed to tackle optimization problems that are intractable for classical computers.
These algorithms could lead to significant improvements in training neural networks and developing more accurate predictive models. The difference isn’t simply a matter of speed; some problems might be solvable only using quantum algorithms.
Examples of AI Problems Where Quantum Computing Could Offer Significant Speedups
Several AI problems stand to benefit significantly from quantum speedups. One key area is drug discovery. Simulating molecular interactions is incredibly computationally intensive, but quantum computers could drastically reduce the time and resources needed to design new drugs and therapies. Another example is materials science, where quantum simulations could accelerate the discovery of new materials with specific properties.
Financial modeling, particularly in areas like portfolio optimization and risk management, could also benefit from the speed and power of quantum computers to analyze massive datasets and predict market trends more accurately. Furthermore, advancements in quantum machine learning could enable the development of more robust and efficient AI systems for tasks like fraud detection and cybersecurity, leading to more effective solutions for complex problems.
Current State of Quantum Computing and its Impact on AI Development
Quantum computing is still in its nascent stages, but its potential to revolutionize AI is undeniable. While not yet ready to replace classical computers for most AI tasks, the field is making rapid progress, and understanding its current limitations and potential is crucial. This section will explore the current state of quantum computing, highlighting its impact on AI development and the challenges that remain.Current limitations significantly restrict the application of quantum computers to real-world AI problems.
The most prominent constraint is the limited number of qubits available in current quantum computers. These are prone to errors, and maintaining coherence (the delicate quantum state) is extremely challenging. This leads to a high error rate, limiting the complexity of problems that can be solved effectively. Furthermore, the development and maintenance of quantum computers are incredibly expensive, restricting access and hindering widespread adoption.
As a result, current applications are primarily focused on research and proof-of-concept demonstrations, rather than large-scale deployment in practical AI systems.
Limitations of Quantum Computers and Their Impact on Real-World AI Applications
The fragility of quantum states and the high error rates are major obstacles. Current quantum computers are far from fault-tolerant, meaning that even small errors can cascade and render results unreliable. This necessitates the development of advanced error correction techniques, which are currently computationally expensive and limit the effective number of qubits available for computation. The lack of readily available, large-scale quantum computers also restricts the scope of AI applications.
Existing algorithms, while showing promise, are often limited in their applicability due to these constraints. For example, while quantum machine learning algorithms can theoretically outperform classical algorithms for certain tasks, the practical implementation is hindered by the limited qubit count and coherence times.
Technological Hurdles in Quantum Computing for AI
Several significant technological hurdles must be overcome for quantum computing to significantly impact AI. Improving qubit coherence times is paramount. Longer coherence times allow for more complex computations before errors accumulate. Developing robust error correction codes is also critical to mitigate the effects of noise and errors in quantum computations. Scaling up the number of qubits while maintaining coherence is another major challenge.
Current quantum computers have a relatively small number of qubits, limiting their computational power. Finally, the development of more efficient and user-friendly quantum programming languages and software tools is essential to make quantum computing accessible to a wider range of AI researchers and developers. Addressing these challenges requires significant advances in materials science, engineering, and computer science.
Quantum Computing Architectures and Their Suitability for AI Tasks
Different quantum computing architectures exist, each with its strengths and weaknesses concerning AI applications. Superconducting qubit-based systems are currently the most advanced, offering relatively high coherence times and scalability, but they require extremely low temperatures for operation. Trapped ion systems are another promising approach, offering good coherence times and scalability potential, but they are more complex to control.
Photonic quantum computers utilize photons as qubits and offer the potential for high connectivity and scalability, but achieving high fidelity operations remains a challenge. The choice of architecture depends on the specific AI task and the trade-offs between coherence times, scalability, and complexity. For instance, tasks requiring a large number of interconnected qubits might benefit from photonic systems, while tasks requiring high precision might be better suited to superconducting or trapped ion systems.
Examples of Quantum Algorithms in AI Research
Several quantum algorithms are being explored for AI applications, demonstrating potential advantages over classical algorithms in specific areas.
Algorithm Name | Classical vs Quantum Performance | Application Area | Limitations |
---|---|---|---|
Quantum Approximate Optimization Algorithm (QAOA) | Potentially faster for certain optimization problems than classical algorithms, but performance depends on problem structure and hardware limitations. | Optimization problems, machine learning model training | Susceptible to noise and limited scalability on current hardware. |
Variational Quantum Eigensolver (VQE) | Can potentially find ground states of molecules more efficiently than classical methods for certain molecules. | Quantum chemistry, materials science, drug discovery | Requires significant classical computation for optimization and is sensitive to noise. |
Quantum Support Vector Machines (QSVM) | Theoretical speedup compared to classical SVM, but practical advantage remains limited by hardware constraints. | Classification tasks, pattern recognition | Requires significant classical preprocessing and post-processing. Limited by qubit availability and error rates. |
The Debate Surrounding Quantum Supremacy Claims: Understanding The Quantum Supremacy Debate In The Context Of AI
The claim of achieving “quantum supremacy”—a quantum computer outperforming the best classical computers on a specific task—has sparked considerable debate. This stems from the difficulty in definitively proving supremacy and the inherent complexities of both quantum and classical computing architectures. The arguments for and against hinge on the criteria used, the specific problem chosen, and the methods of verification employed.
Arguments For and Against Quantum Supremacy Claims
The debate around quantum supremacy is multifaceted. Proponents point to successful experiments demonstrating a quantum computer’s speed advantage on a specific, albeit possibly contrived, problem. They argue that these experiments represent a significant milestone in quantum computing development, paving the way for future advancements with broader practical applications. Conversely, critics question the practical relevance of the chosen benchmark problems and the scalability of the achieved quantum advantage.
They highlight the limitations of current quantum computers, emphasizing the considerable resources required and the error rates that currently plague them. Furthermore, they point to the possibility of future classical algorithms that could potentially match or surpass the performance of current quantum computers on these specific tasks.
Criteria Used to Define and Verify Quantum Supremacy
Establishing quantum supremacy requires rigorous criteria. A key criterion is the demonstrable speed advantage of a quantum computer over the best known classical algorithms for a specific computational task. This advantage must be substantial and verifiable, meaning the results obtained by the quantum computer must be independently confirmed and shown to be unattainable by classical computers within a reasonable timeframe.
The chosen computational task needs to be practically relevant, even if limited in scope, to demonstrate the potential of quantum computing. Verification often involves sophisticated statistical methods to rule out the possibility of classical simulation or error.
Comparison of Approaches to Demonstrating Quantum Advantage, Understanding the quantum supremacy debate in the context of AI
Different research groups have employed various approaches to demonstrating quantum advantage. Some focus on specific sampling problems, such as generating random numbers from a complex probability distribution, a task that is notoriously difficult for classical computers. Others explore applications in quantum chemistry or materials science, aiming to solve problems intractable for classical methods. Each approach has its own strengths and weaknesses.
Sampling problems offer a relatively clean benchmark, but their practical applications might be limited. Simulations of quantum systems, while potentially more impactful, are more complex to verify and require more sophisticated error mitigation techniques.
Key Arguments Summarized
- Pro-Supremacy Arguments:
- Successful experiments demonstrate a clear speed advantage in specific tasks.
- Represents a significant milestone in quantum computing development.
- Paves the way for future advancements with broader applications.
- Anti-Supremacy Arguments:
- Benchmark problems may lack practical relevance and scalability.
- Current quantum computers have significant limitations (error rates, resource requirements).
- Future classical algorithms might surpass current quantum computer performance on these specific tasks.
- The claim of “supremacy” is misleading, as it doesn’t necessarily translate to real-world applications.
Potential Applications of Quantum AI in Various Fields
The convergence of quantum computing and artificial intelligence promises to revolutionize numerous sectors, offering solutions to currently intractable problems. Quantum AI leverages the unique properties of quantum mechanics – superposition and entanglement – to develop algorithms capable of exponentially faster computation and more sophisticated data analysis than classical AI. This unlocks possibilities across various fields, leading to significant advancements in scientific discovery, technological innovation, and societal impact.
Quantum AI in Drug Discovery
Quantum algorithms hold immense potential for accelerating drug discovery, a process traditionally hampered by the vast chemical space and complex biological interactions. A hypothetical scenario involves designing a new drug targeting a specific protein implicated in a disease. Classical methods rely on computationally expensive simulations and high-throughput screening, often yielding limited results. In contrast, a quantum approach could employ Quantum Approximate Optimization Algorithm (QAOA) to explore the vast conformational space of potential drug molecules, identifying promising candidates with high binding affinity to the target protein.
Furthermore, Variational Quantum Eigensolver (VQE) could be used to accurately simulate the quantum mechanical properties of the drug-protein interaction, predicting its efficacy and potential side effects with unprecedented precision. The expected outcome is a significant reduction in drug development time and cost, leading to faster availability of life-saving medications. For example, imagine using QAOA to optimize the structure of a potential antiviral drug, then using VQE to simulate its interaction with the virus’s protein, predicting its efficacy in inhibiting viral replication with far greater accuracy than classical methods.
Quantum Machine Learning in Financial Modeling and Risk Assessment
Quantum machine learning (QML) algorithms offer the potential to revolutionize financial modeling and risk assessment by handling significantly larger datasets and identifying complex patterns imperceptible to classical methods. This could lead to more accurate predictions of market trends, improved portfolio optimization, and more robust risk management strategies.
Quantum machine learning has the potential to unlock new insights from complex financial data, leading to more accurate predictions and better decision-making.
However, the implementation of QML in finance faces challenges.
The development of robust and scalable quantum algorithms for financial applications, along with the need for specialized quantum hardware, present significant hurdles.
For instance, a quantum support vector machine (Q-SVM) could analyze vast amounts of financial market data to identify subtle correlations and predict market movements with higher accuracy than classical SVMs. This could significantly improve trading strategies and risk management.
Research Project: Quantum AI in Materials Science
This research project aims to investigate the application of quantum machine learning algorithms, specifically Quantum Neural Networks (QNNs), for the discovery of novel materials with enhanced properties. The project will focus on developing and training QNNs to predict the electronic and structural properties of materials based on their chemical composition and crystal structure. The goal is to identify materials with superior strength, conductivity, or catalytic activity for various applications, such as energy storage, electronics, and catalysis.
The expected outcomes include the identification of novel materials with superior properties, accelerated materials discovery, and a deeper understanding of material behavior at the quantum level. This research will utilize both simulation and experimental validation to ensure the accuracy and reliability of the QNN models.
Quantum AI in Climate Change Modeling and Prediction
Climate change modeling involves complex systems with numerous interacting variables. Quantum AI offers the potential to significantly improve the accuracy and efficiency of these models. Quantum computers could handle the vast datasets and intricate interactions involved in climate modeling, leading to more precise predictions of future climate scenarios. Quantum algorithms could simulate atmospheric and oceanic processes with greater accuracy than classical methods, improving our understanding of climate change and aiding in the development of effective mitigation strategies.
For example, quantum algorithms could more accurately model the complex interactions between atmospheric gases, ocean currents, and ice sheets, leading to more precise predictions of sea-level rise and extreme weather events. This improved accuracy could significantly enhance our ability to plan for and adapt to the effects of climate change.
Ethical and Societal Implications of Quantum AI
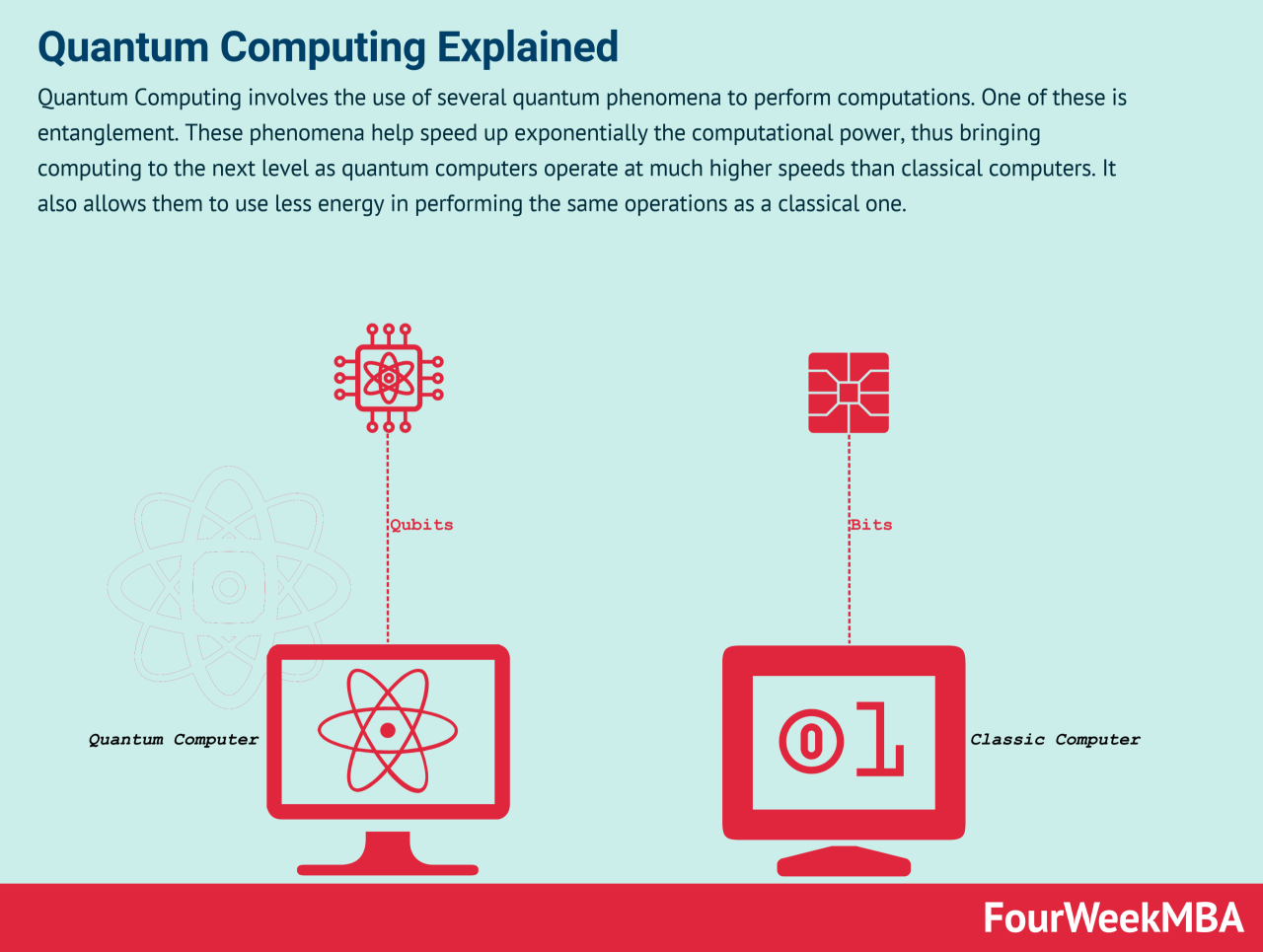
Source: fourweekmba.com
The advent of quantum AI presents a double-edged sword, promising unprecedented advancements while simultaneously raising profound ethical and societal concerns. Its potential to revolutionize various sectors necessitates a careful consideration of its impact on individuals, communities, and the global landscape. Failing to address these implications proactively could lead to unforeseen and potentially harmful consequences.
The transformative power of quantum AI stems from its ability to solve complex problems currently intractable for classical computers. This capability will undoubtedly reshape numerous industries, from medicine and materials science to finance and cybersecurity. However, this transformative potential is intertwined with significant risks that demand careful consideration and proactive mitigation strategies.
Societal Impact of Widespread Quantum AI Adoption
The widespread adoption of quantum AI will likely lead to significant societal shifts. Increased automation driven by quantum algorithms could lead to job displacement in various sectors, requiring proactive retraining and workforce adaptation initiatives. Furthermore, the concentration of quantum computing power in the hands of a few powerful entities could exacerbate existing inequalities, creating a digital divide and potentially reinforcing existing power structures.
On the positive side, quantum AI could revolutionize healthcare through faster drug discovery and personalized medicine, leading to improved health outcomes and increased longevity for many. The societal impact will be a complex interplay of these positive and negative forces.
Ethical Concerns Related to Quantum AI Development and Deployment
Several ethical concerns surround the development and deployment of quantum AI systems. Bias in training data could lead to discriminatory outcomes, perpetuating and even amplifying existing societal biases. The potential for misuse in areas like autonomous weapons systems or sophisticated surveillance technologies poses significant ethical challenges. Furthermore, the opacity of some quantum algorithms can make it difficult to understand their decision-making processes, raising concerns about accountability and transparency.
The lack of clear guidelines and regulations for the development and deployment of quantum AI necessitates a proactive approach to establishing ethical frameworks.
Examples of Potential Misuse and Unintended Consequences
The potential for misuse of quantum AI is substantial. Sophisticated quantum algorithms could be used to break current encryption methods, jeopardizing sensitive data and national security. The development of autonomous weapons systems powered by quantum AI raises serious concerns about accountability and the potential for unintended escalation of conflicts. Furthermore, the use of quantum AI in surveillance could lead to unprecedented levels of mass surveillance, eroding privacy and potentially suppressing dissent.
These examples highlight the urgency of developing robust safeguards and ethical guidelines to mitigate these risks.
Suggestions for Responsible Development and Governance of Quantum AI
Responsible development and governance of quantum AI require a multi-faceted approach. This includes investing in research on explainable AI (XAI) to improve the transparency and understandability of quantum algorithms. Developing robust ethical guidelines and regulations, incorporating input from diverse stakeholders, is crucial. International collaboration is essential to establish common standards and prevent a global “quantum arms race.” Furthermore, promoting education and public awareness about the potential benefits and risks of quantum AI is critical for fostering informed public discourse and responsible innovation.
A proactive and collaborative approach is essential to harness the potential of quantum AI while mitigating its risks.
Epilogue
The quantum supremacy debate is far from settled, but the potential impact of quantum computing on AI is undeniable. While significant technological challenges remain, the ongoing research and development in this field are paving the way for transformative advancements across diverse sectors. The ethical considerations surrounding quantum AI’s development and deployment must be addressed proactively to ensure its responsible and beneficial integration into society.
Ultimately, the journey towards realizing the full potential of quantum AI will require continued collaboration between researchers, policymakers, and the wider community.
FAQ
What is the difference between a quantum bit (qubit) and a classical bit?
A classical bit represents either a 0 or a 1. A qubit, however, can represent a 0, a 1, or a superposition of both simultaneously, allowing for vastly increased computational power.
What are some examples of real-world applications of quantum computing beyond AI?
Quantum computing has potential applications in materials science (designing new materials), cryptography (breaking current encryption methods and creating new, quantum-resistant ones), and optimization problems (logistics, supply chain management).
How long until quantum computers replace classical computers for all tasks?
It’s unlikely that quantum computers will entirely replace classical computers. Quantum computers excel at specific types of problems, while classical computers remain superior for many other tasks. A more likely scenario is a hybrid approach, leveraging the strengths of both.
What are the biggest obstacles to widespread quantum computing adoption?
Major obstacles include maintaining qubit coherence (stability), scaling up the number of qubits while preserving their functionality, and developing error correction techniques to improve the reliability of quantum computations.