Quantum AIs Role in Personalized Healthcare
The role of quantum AI in personalized medicine and healthcare is poised to revolutionize how we diagnose, treat, and prevent diseases. Imagine a future where treatments are precisely tailored to your unique genetic makeup, lifestyle, and environment, leading to faster recovery times and fewer side effects. This is the promise of quantum AI, a powerful technology leveraging the principles of quantum mechanics to solve complex problems far beyond the capabilities of classical computers.
This exploration delves into the potential of quantum AI to transform healthcare, from drug discovery to personalized treatment plans, while acknowledging the challenges and ethical considerations that lie ahead.
Quantum AI’s unique ability to process vast amounts of data and perform complex simulations opens doors to breakthroughs in various areas. For instance, it can significantly accelerate drug discovery by simulating molecular interactions with unprecedented accuracy, leading to faster development of new therapies. In diagnostics, quantum algorithms can analyze medical images with greater speed and precision than traditional methods, potentially enabling earlier and more accurate disease detection.
Furthermore, quantum AI can personalize treatment plans by considering individual patient characteristics, optimizing therapies for maximum effectiveness and minimizing adverse reactions.
Introduction to Quantum AI and its Potential in Healthcare
Quantum computing represents a paradigm shift in computational power, leveraging the principles of quantum mechanics to solve problems intractable for even the most powerful classical computers. Unlike classical bits which represent either 0 or 1, quantum bits, or qubits, can exist in a superposition, representing 0 and 1 simultaneously. This, combined with phenomena like quantum entanglement, allows quantum computers to explore vast solution spaces exponentially faster than classical counterparts.
This enhanced computational power has profound implications for various fields, including healthcare.Quantum AI, in the context of healthcare, differs significantly from classical AI by its ability to handle exponentially larger and more complex datasets. Classical AI struggles with the massive, high-dimensional data generated by genomics, proteomics, and medical imaging. Quantum AI algorithms, however, can potentially analyze this data more efficiently, leading to more accurate diagnoses, personalized treatments, and improved drug discovery.
For example, classical AI might struggle to predict protein folding accurately for large molecules, a crucial step in drug development. Quantum AI, with its ability to model complex quantum interactions, offers the potential for more accurate and faster predictions.
Quantum AI’s Benefits in Personalized Medicine
The potential benefits of quantum AI in revolutionizing personalized medicine are substantial. Its superior computational power enables the analysis of individual patient data—genomics, lifestyle, environmental factors—to create highly personalized treatment plans. This is particularly relevant in areas like cancer treatment, where genetic variations significantly impact drug efficacy and response. Quantum AI could analyze a patient’s unique genomic profile to predict the most effective chemotherapy regimen, minimizing side effects and maximizing chances of success.
Furthermore, quantum machine learning algorithms can identify subtle patterns and correlations in large medical datasets that classical methods might miss, leading to earlier and more accurate diagnoses of diseases like Alzheimer’s or Parkinson’s, where early detection is critical for effective intervention. The development of new drugs and therapies is also accelerated; quantum simulations can predict the effectiveness of drug candidates with greater accuracy and speed, reducing the time and cost associated with traditional drug development pipelines.
For example, a quantum computer could simulate the interaction of a drug molecule with a specific protein target far more accurately than current classical methods, potentially leading to the discovery of more effective and safer medications.
Quantum AI for Drug Discovery and Development
Quantum computing holds immense promise for revolutionizing drug discovery and development, a traditionally lengthy and expensive process. By leveraging the unique capabilities of quantum algorithms and machine learning, researchers can significantly accelerate the identification and development of novel therapeutics. This approach promises to reduce costs, shorten timelines, and ultimately improve patient outcomes.Quantum algorithms offer a powerful approach to simulate molecular interactions, a crucial step in drug discovery.
Classical computers struggle to accurately model the complex behavior of molecules, particularly large and intricate ones like proteins. Quantum computers, however, can potentially handle these simulations with far greater accuracy and efficiency, enabling researchers to predict how drug candidates will interact with their target molecules. This predictive power is crucial for identifying promising drug candidates early in the development process, saving time and resources.
Quantum Algorithm Acceleration of Molecular Simulations
Quantum algorithms, such as Quantum Monte Carlo and Variational Quantum Eigensolver (VQE), can be employed to simulate the quantum mechanical behavior of molecules. These algorithms can accurately calculate the energy levels and other properties of molecules, allowing researchers to predict binding affinities, reaction rates, and other crucial factors for drug efficacy. For example, VQE can be used to optimize the geometry of a molecule, finding the lowest energy configuration, which is crucial for predicting its stability and reactivity.
This level of detail is difficult, if not impossible, to achieve with classical computational methods for complex molecules.
Quantum Machine Learning for Drug Candidate Identification
Quantum machine learning (QML) algorithms can analyze vast datasets of molecular properties and biological activity to identify potential drug candidates. QML algorithms can uncover patterns and relationships that are hidden from classical machine learning algorithms, leading to the discovery of novel drug candidates with improved efficacy and reduced side effects. Imagine a QML model trained on a massive dataset of known drug molecules and their associated properties.
This model could then predict the likelihood of a new molecule being a successful drug candidate based on its structural features and other relevant properties. This drastically reduces the need for extensive and costly experimental screening.
A Hypothetical Workflow for Quantum AI in Clinical Trials
The integration of quantum AI into clinical trials could streamline the entire process, from patient selection to data analysis. A hypothetical workflow might look like this:
- Patient Selection and Stratification: QML algorithms could analyze patient data (genomics, proteomics, etc.) to identify subgroups of patients most likely to respond to a specific treatment, thus improving trial efficiency and reducing the risk of adverse events. This personalized approach allows for more targeted clinical trials.
- Dosage Optimization: Quantum simulations could predict optimal drug dosages for individual patients based on their unique characteristics, minimizing side effects and maximizing therapeutic benefits. This personalized approach leads to better treatment outcomes.
- Real-time Monitoring and Analysis: Quantum sensors and AI-powered data analysis could monitor patient responses in real-time, enabling adjustments to treatment plans as needed. This dynamic approach allows for adaptive clinical trials, which can respond to emerging data and improve efficiency.
- Data Analysis and Interpretation: QML algorithms can analyze the vast amounts of data generated during clinical trials, identifying subtle patterns and relationships that might be missed by traditional methods. This enhanced data analysis could lead to a more complete understanding of the drug’s efficacy and safety profile.
Quantum AI in Diagnostics and Disease Prediction
Quantum computing’s immense processing power holds transformative potential for medical diagnostics and disease prediction. By leveraging quantum algorithms, we can analyze complex medical data far more efficiently than classical methods, leading to faster, more accurate diagnoses and improved patient outcomes. This section explores the advantages of quantum AI in this field, comparing its performance to traditional approaches and highlighting specific applications.Quantum AI-powered diagnostic tools offer the potential for significantly increased accuracy and speed compared to traditional methods.
Classical diagnostic techniques often rely on relatively simple algorithms and may be limited by the complexity of the data they can process. In contrast, quantum algorithms can handle vast datasets, identifying subtle patterns and correlations that might be missed by classical approaches. This leads to more precise diagnoses and earlier detection of diseases, enabling timely interventions. The speed advantage stems from the inherent parallelism of quantum computation, allowing for significantly faster processing of complex medical images and genomic data.
Quantum AI’s Enhanced Diagnostic Capabilities
Quantum AI’s superior computational capabilities translate to improved diagnostic accuracy and speed across various medical domains. For instance, in oncology, quantum algorithms could analyze medical images (MRI, CT scans, PET scans) with greater precision to detect cancerous tumors at earlier stages, when treatment is often more effective. Similarly, in cardiology, quantum AI could analyze electrocardiograms (ECGs) and other cardiac data to identify subtle anomalies indicative of heart disease, leading to earlier interventions and improved patient survival rates.
In neurology, quantum AI could assist in diagnosing neurodegenerative diseases like Alzheimer’s disease by analyzing brain imaging data and identifying subtle biomarkers indicative of disease progression. Early detection is crucial in all these cases, and quantum AI offers a powerful tool to achieve it.
Examples of Quantum Algorithms in Medical Image Analysis
Several quantum algorithms are being explored for analyzing medical images and generating detailed reports. These algorithms leverage the unique properties of quantum mechanics to perform complex computations that are beyond the capabilities of classical computers. For example, quantum machine learning algorithms can be trained on large datasets of medical images to identify patterns associated with specific diseases. Quantum annealing algorithms can be used to optimize the parameters of these models, leading to improved accuracy and efficiency.
Quantum tomography algorithms can be used to reconstruct 3D images from sparse data, reducing the need for lengthy scanning procedures.
Algorithm | Disease | Accuracy | Speed |
---|---|---|---|
Quantum Support Vector Machine (QSVM) | Cancer detection (e.g., breast cancer) | Potentially higher than classical SVM, research ongoing | Significantly faster for large datasets |
Quantum Annealing | Alzheimer’s disease diagnosis (based on brain scans) | Improved biomarker identification compared to classical methods, research ongoing | Faster optimization of diagnostic models |
Quantum Principal Component Analysis (QPCA) | Cardiovascular disease diagnosis (ECG analysis) | Improved detection of subtle anomalies, research ongoing | Faster dimensionality reduction of ECG data |
Note: The accuracy and speed improvements listed in the table are based on theoretical analyses and early experimental results. Further research is needed to fully validate these claims in real-world clinical settings. The “research ongoing” notation highlights that these are active areas of development, with full clinical validation still to come.
Quantum AI for Personalized Treatment Planning
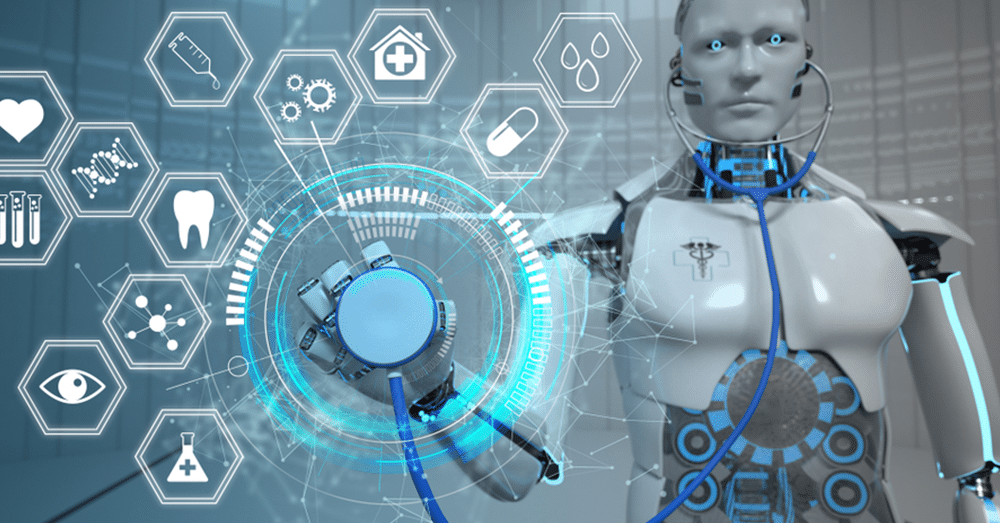
Source: hestabit.com
Quantum AI holds immense potential for revolutionizing treatment planning by leveraging its ability to process vast amounts of complex data and identify intricate patterns invisible to classical methods. This allows for the creation of highly individualized treatment strategies tailored to each patient’s unique characteristics, ultimately leading to improved outcomes and reduced side effects. The ability to analyze genetic predispositions, lifestyle factors, and tumor characteristics simultaneously offers a level of personalization previously unattainable.Quantum algorithms can analyze patient data, including genomic information, medical history, lifestyle factors, and imaging data, to predict treatment response and identify optimal treatment strategies.
This contrasts with traditional methods which often rely on population averages and less comprehensive data analysis. The enhanced computational power of quantum computers allows for the exploration of a far wider range of treatment options and their potential outcomes, leading to more informed decision-making.
Optimizing Radiation Therapy and Chemotherapy Regimens
Quantum AI can significantly improve the efficacy and safety of radiation and chemotherapy by optimizing treatment plans based on individual patient characteristics. For example, quantum algorithms can model the complex interactions between radiation beams and tumor cells, considering factors like tumor location, size, and surrounding healthy tissue. This allows for the precise targeting of cancerous cells while minimizing damage to healthy tissues, leading to reduced side effects and improved treatment outcomes.
Similarly, in chemotherapy, quantum AI can predict individual responses to different drug combinations, optimizing the selection and dosage to maximize efficacy and minimize toxicity. This precision approach minimizes unnecessary side effects and improves the patient’s quality of life during treatment.
A Hypothetical Case Study: Personalized Cancer Treatment, The role of quantum AI in personalized medicine and healthcare
This case study illustrates how quantum AI could personalize cancer treatment. Imagine a patient, Sarah, diagnosed with a particularly aggressive form of breast cancer. Traditional methods might suggest a standard chemotherapy regimen with a high probability of severe side effects. However, using quantum AI, a detailed analysis of Sarah’s genome, tumor biopsy, and lifestyle factors is conducted.The steps involved are:
- Comprehensive Data Acquisition: Gathering Sarah’s genetic information, tumor characteristics from biopsies, lifestyle details (diet, exercise, smoking history), and medical history. This involves sequencing her genome to identify genetic mutations influencing drug response and tumor growth.
- Quantum AI Modeling: Utilizing a quantum algorithm to model the growth and response of Sarah’s specific tumor to various chemotherapy drugs and combinations. This model incorporates her unique genetic profile and other factors.
- Treatment Plan Optimization: The quantum AI identifies the chemotherapy regimen with the highest probability of success and the lowest risk of severe side effects for Sarah. This might involve a novel combination of drugs or a tailored dosage schedule.
- Real-time Monitoring and Adjustment: During treatment, the quantum AI continuously monitors Sarah’s response and adjusts the regimen as needed. This allows for dynamic optimization based on her evolving condition.
The quantum AI approach might identify a specific drug combination that targets Sarah’s unique tumor mutations effectively, while minimizing the risk of common side effects like hair loss, nausea, and fatigue. This personalized approach, unlike traditional methods, is predicted to significantly improve Sarah’s chances of successful treatment and improve her quality of life during the process. The predicted outcome, based on the model’s simulations, is a significantly higher survival rate and reduced severity of side effects compared to a standard treatment approach.
Quantum AI and Data Privacy in Healthcare
The immense potential of quantum AI in revolutionizing healthcare is undeniable, but its implementation necessitates a robust framework for addressing the inherent challenges related to data privacy and security. The sensitivity of patient data, coupled with the power of quantum computing, necessitates a proactive and comprehensive approach to safeguarding information. Failure to do so could lead to serious breaches of trust and potentially catastrophic consequences for individuals and the healthcare system as a whole.The unique capabilities of quantum computers, while offering advancements in diagnostics and treatment, also present new vulnerabilities.
Quantum algorithms could potentially break many currently used encryption methods, rendering traditional security measures inadequate. Therefore, developing new cryptographic techniques resistant to quantum attacks is paramount. Furthermore, the sheer volume and complexity of healthcare data being processed by quantum AI systems amplify the risk of unauthorized access and data breaches. Effective data governance and stringent security protocols are critical to mitigating these risks.
Data Privacy Challenges in Quantum AI Healthcare Applications
The increased computational power of quantum AI systems poses significant threats to the confidentiality, integrity, and availability of patient data. Traditional encryption methods, like RSA, are vulnerable to attacks from sufficiently powerful quantum computers. This vulnerability necessitates a shift towards post-quantum cryptography, which employs algorithms resistant to attacks from both classical and quantum computers. Another challenge lies in the potential for data leakage during the processing of sensitive patient information by quantum AI algorithms.
Robust access control mechanisms are crucial to limit access to authorized personnel only, preventing unauthorized data access and manipulation. Finally, the complexity of quantum algorithms can make it difficult to audit and verify the integrity of the data processing pipeline, increasing the risk of undetected errors or malicious manipulations.
Robust Data Encryption and Access Control
Implementing robust data encryption and access control mechanisms is fundamental to protecting patient information within quantum AI healthcare applications. This involves utilizing post-quantum cryptographic algorithms, such as lattice-based cryptography or code-based cryptography, to encrypt sensitive data at rest and in transit. These algorithms are designed to withstand attacks from even the most powerful quantum computers. Furthermore, implementing strict access control policies, based on the principle of least privilege, is crucial.
This ensures that only authorized personnel with a legitimate need to access specific data have the necessary permissions. Role-based access control (RBAC) and attribute-based access control (ABAC) are examples of mechanisms that can be employed to achieve fine-grained control over data access. Regular security audits and penetration testing are essential to identify and address potential vulnerabilities. These audits should encompass the entire quantum AI system, including the hardware, software, and data storage infrastructure.
Framework for Ethical and Responsible Use of Quantum AI in Healthcare
A comprehensive framework for the ethical and responsible use of quantum AI in healthcare must prioritize data privacy and security. This framework should encompass several key elements. Firstly, a robust data governance policy should be established, outlining clear guidelines for data collection, storage, processing, and disposal. This policy should adhere to all relevant data privacy regulations, such as HIPAA in the United States or GDPR in Europe.
Secondly, the framework should mandate the implementation of rigorous security measures, including post-quantum cryptography, robust access control, and regular security audits. Thirdly, transparency and accountability are crucial. The framework should ensure that data subjects have the right to access, correct, and delete their personal data, and that any use of their data is transparent and justifiable. Finally, the framework should incorporate a mechanism for independent oversight and ethical review of quantum AI applications in healthcare, ensuring responsible innovation and minimizing potential risks.
This could involve the establishment of an ethics committee or regulatory body specifically tasked with reviewing the ethical implications of quantum AI technologies in healthcare.
Challenges and Limitations of Quantum AI in Healthcare: The Role Of Quantum AI In Personalized Medicine And Healthcare
While the potential of quantum AI in revolutionizing healthcare is immense, several significant hurdles currently limit its widespread adoption. These challenges span technological limitations, computational resource constraints, and the need for substantial further research and development. Overcoming these obstacles is crucial for realizing the full transformative potential of this exciting field.Current limitations in quantum computing technology significantly impede the immediate application of quantum AI in healthcare settings.
The technology is still in its nascent stages, facing considerable engineering and design challenges. The fragility of quantum systems, their susceptibility to noise and decoherence, and the difficulty in scaling up the number of qubits needed for complex healthcare problems all pose significant barriers. For example, simulating the intricate interactions of molecules for drug discovery requires a far greater number of stable qubits than are currently available in existing quantum computers.
Qubit Instability and Scalability
Quantum computers rely on qubits, which are incredibly sensitive to their environment. Even minor fluctuations in temperature or electromagnetic fields can cause errors in computation, a phenomenon known as decoherence. This instability necessitates complex error correction techniques, which further increase the computational demands and complexity. Moreover, scaling up the number of qubits to a level required for solving truly impactful healthcare problems remains a major technological hurdle.
Current quantum computers have a limited number of qubits, restricting their capabilities in handling large and complex datasets typical in healthcare applications. Progress in this area is ongoing, with researchers exploring various qubit technologies and error correction strategies, but significant breakthroughs are still needed before quantum computers reach the required level of stability and scalability.
Computational Cost and Resource Requirements
Quantum AI algorithms, while potentially more powerful than classical algorithms for specific tasks, are extremely computationally expensive. Running these algorithms requires specialized and expensive hardware, along with significant computational resources. The energy consumption of quantum computers is also a significant factor, posing both economic and environmental challenges. For instance, simulating the behavior of a complex protein to understand its function and potential drug interactions requires immense computational power, far exceeding the capabilities of current classical computers and posing a significant challenge even for the most advanced quantum computers currently under development.
This high cost limits accessibility and hinders the widespread implementation of quantum AI in healthcare.
Need for Further Research and Development
Overcoming the challenges Artikeld above requires significant investment in further research and development across multiple disciplines. This includes advancements in quantum hardware, the development of more robust and efficient quantum algorithms tailored for healthcare applications, and the creation of appropriate software and infrastructure to support these algorithms. Furthermore, research is needed to address the specific data requirements and challenges posed by integrating quantum AI into existing healthcare workflows and data management systems.
The development of standardized protocols and benchmarks for evaluating the performance and reliability of quantum AI algorithms in healthcare settings is also critical for ensuring responsible and effective implementation. Only through continued collaborative efforts across academia, industry, and government can the full potential of quantum AI in healthcare be unlocked.
The Future of Quantum AI in Personalized Medicine
The next 5-10 years promise a dramatic shift in healthcare, driven by the burgeoning field of quantum artificial intelligence. While still in its nascent stages, quantum AI’s potential to revolutionize personalized medicine is undeniable, offering unprecedented opportunities to improve diagnostic accuracy, accelerate drug discovery, and tailor treatments to individual patient needs. This transformation will not only enhance patient outcomes but also contribute to a more cost-effective healthcare system.The integration of quantum AI into healthcare delivery will likely proceed in a phased manner, with initial applications focusing on areas where its unique capabilities offer the most immediate advantages.
We can anticipate a gradual increase in the sophistication and breadth of quantum AI applications, leading to a fundamental reshaping of the healthcare landscape.
Potential Impact of Quantum AI on Healthcare Delivery in the Next 5-10 Years
Within the next five to ten years, we can expect quantum AI to significantly impact several key areas of healthcare delivery. For instance, more accurate and faster disease diagnostics will be possible due to quantum algorithms’ ability to analyze complex medical images and genomic data far surpassing the capabilities of classical algorithms. This will lead to earlier detection of diseases like cancer, allowing for earlier and more effective interventions.
Furthermore, the accelerated drug discovery process enabled by quantum AI will bring novel therapies to market much faster, potentially addressing currently untreatable diseases. Personalized treatment plans, tailored to individual genetic profiles and other patient-specific factors, will become increasingly common, leading to improved treatment efficacy and reduced side effects.
Transformation of the Healthcare Landscape: Patient Outcomes and Cost-Effectiveness
Quantum AI’s impact on patient outcomes will be multifaceted. Improved diagnostic accuracy will lead to earlier disease detection and intervention, resulting in better treatment outcomes and potentially increased life expectancy. The development of personalized therapies will minimize adverse drug reactions and optimize treatment efficacy for each individual, leading to improved quality of life. The accelerated drug discovery process will make novel treatments available sooner, addressing unmet medical needs and improving survival rates for patients with serious illnesses.The cost-effectiveness of healthcare will also benefit from quantum AI.
While the initial investment in quantum computing infrastructure will be substantial, the long-term cost savings resulting from improved diagnostic accuracy, reduced treatment costs due to personalized medicine, and accelerated drug development will likely outweigh the initial investment. For example, reducing hospital readmissions through better disease prediction and personalized treatment plans can lead to significant cost savings for healthcare systems.
The faster development of effective drugs will also reduce the overall cost of healthcare by shortening the time needed to treat diseases.
Timeline of Key Milestones and Advancements
The advancement of quantum AI in healthcare is expected to follow a trajectory marked by several key milestones.
A projected timeline might look like this:
Year | Milestone | Description |
---|---|---|
2024-2026 | Proof-of-concept studies in specific areas | Small-scale studies demonstrating the feasibility of quantum AI algorithms for tasks such as drug discovery and image analysis. Examples include successful application in identifying promising drug candidates or improving the accuracy of cancer detection in small datasets. |
2027-2029 | Integration into existing healthcare workflows | Pilot programs integrating quantum AI tools into clinical settings. For example, a hospital might implement a quantum AI-powered diagnostic tool for a specific type of cancer, evaluating its performance and integrating it into their routine workflow. |
2030-2035 | Widespread adoption of quantum AI in specific applications | Quantum AI becomes a standard tool in specific healthcare areas, such as drug discovery and personalized oncology. Examples include widespread use of quantum AI-designed drugs and personalized treatment plans for various cancers becoming commonplace. |
Final Conclusion
The integration of quantum AI into personalized medicine and healthcare holds immense potential to reshape the future of medical practice. While challenges remain in terms of technology development and ethical considerations, the advancements already being made are incredibly promising. The ability to tailor treatments to individual patients, accelerate drug discovery, and improve diagnostic accuracy offers a future where healthcare is more effective, efficient, and personalized than ever before.
Continued research and responsible development are crucial to realizing this transformative vision and ensuring equitable access to these groundbreaking technologies.
General Inquiries
What are the main ethical concerns surrounding the use of quantum AI in healthcare?
Major ethical concerns include data privacy and security (protecting sensitive patient information), algorithmic bias (ensuring fairness and avoiding discrimination), and access equity (making these advanced technologies available to all patients, regardless of socioeconomic status).
How long will it take before quantum AI is widely used in clinical settings?
It’s difficult to give a precise timeline. While some applications are closer to reality than others, widespread adoption will likely take several years, depending on technological advancements, regulatory approvals, and the development of necessary infrastructure.
What is the difference between classical AI and quantum AI in healthcare?
Classical AI relies on traditional computing methods, while quantum AI uses quantum phenomena like superposition and entanglement to solve problems that are intractable for classical computers. This allows quantum AI to handle much larger and more complex datasets, leading to potentially more accurate and insightful results in areas like drug discovery and image analysis.
Will quantum AI replace human doctors?
No. Quantum AI is intended to augment, not replace, human expertise. It will serve as a powerful tool to assist healthcare professionals in making better diagnoses, developing more effective treatments, and improving patient care overall. The human element—compassion, empathy, and clinical judgment—remains irreplaceable.