Quantum Computing in Drug Discovery
Quantum computing in drug discovery is poised to revolutionize the pharmaceutical industry. Traditional methods for drug discovery are often slow, expensive, and inefficient. Quantum computers, with their ability to process vast amounts of data and perform complex simulations far beyond the capabilities of classical computers, offer a powerful new tool to accelerate the development of new therapies. This exciting field leverages unique quantum algorithms to tackle challenges in drug target identification, drug design, and optimization, ultimately aiming to deliver safer and more effective medicines faster.
The potential applications are vast, ranging from simulating molecular interactions with unprecedented accuracy to developing advanced machine learning models for identifying promising drug candidates. This exploration delves into the various quantum algorithms being developed, the challenges inherent in this nascent technology, and the promising future collaborations between quantum computing experts and pharmaceutical researchers. Ultimately, the goal is to harness the power of quantum mechanics to improve human health.
Introduction to Quantum Computing in Drug Discovery
Drug discovery is a complex and time-consuming process, traditionally reliant on classical computing methods. These methods, while powerful, face significant limitations when tackling the intricate challenges inherent in understanding molecular interactions and designing novel therapeutics. The sheer scale of the problem, involving vast chemical spaces and complex simulations, often pushes classical computers to their limits, leading to long development times and high costs.Quantum computing offers a potential paradigm shift in drug discovery by leveraging the principles of quantum mechanics to solve problems intractable for classical computers.
Its unique capabilities, such as superposition and entanglement, enable the exploration of significantly larger chemical spaces and the performance of more accurate and efficient simulations. This has the potential to dramatically accelerate the drug development process, leading to faster identification of promising drug candidates and a reduction in overall costs.
Limitations of Classical Computing in Drug Discovery
Classical computers struggle with the computational complexity of accurately modeling molecular interactions. Simulating the behavior of even moderately sized molecules requires enormous computational resources, often exceeding the capabilities of even the most powerful supercomputers. This limitation restricts the ability to explore a comprehensive range of potential drug candidates and accurately predict their efficacy and safety profiles. Furthermore, the computational cost of optimizing drug molecules for desired properties, such as binding affinity and bioavailability, is prohibitively high for classical methods.
This often necessitates the use of simplified models and approximations, which can compromise the accuracy of the results and lead to missed opportunities.
Potential Advantages of Quantum Computing for Accelerating Drug Development
Quantum computers offer several key advantages over their classical counterparts in drug discovery. Their ability to handle vast datasets and perform complex calculations more efficiently could significantly shorten the time required to identify and optimize potential drug candidates. Quantum algorithms can more accurately model molecular interactions, leading to improved predictions of drug efficacy and safety. This can reduce the reliance on expensive and time-consuming experimental validation, ultimately accelerating the entire drug development pipeline.
For instance, quantum simulations could accurately predict the binding affinity of a drug molecule to its target protein, a crucial step in drug design. This allows researchers to focus their efforts on the most promising candidates, optimizing resource allocation and reducing development costs.
Relevant Quantum Computing Algorithms in Drug Discovery
Several quantum algorithms hold significant promise for accelerating drug discovery. Quantum simulation algorithms, such as the Variational Quantum Eigensolver (VQE) and Quantum Approximate Optimization Algorithm (QAOA), can be used to model the electronic structure of molecules with greater accuracy than classical methods. These algorithms can efficiently calculate molecular properties crucial for drug design, such as binding energies and geometries.
Quantum machine learning algorithms can be used to analyze large datasets of molecular properties and identify patterns that could lead to the discovery of novel drug candidates. For example, quantum machine learning could be used to predict the activity of a drug molecule based on its chemical structure, allowing researchers to quickly screen large libraries of compounds. Furthermore, quantum optimization algorithms can be employed to optimize the design of drug molecules for improved efficacy and reduced side effects.
These algorithms can efficiently explore a vast chemical space to identify optimal drug candidates with desired properties.
Quantum Algorithms for Drug Discovery Applications
Quantum computing offers the potential to revolutionize drug discovery by tackling problems intractable for classical computers. Its power lies in efficiently simulating quantum systems, which are at the heart of molecular interactions crucial for understanding drug behavior. This section explores how specific quantum algorithms can be harnessed for various stages of drug development.Quantum algorithms can simulate molecular interactions by leveraging the inherent quantum nature of molecules.
Classical computers struggle to accurately model the complex interactions between electrons and nuclei in molecules, especially for larger systems. Quantum computers, however, can directly represent these quantum systems and use algorithms to simulate their evolution, providing more accurate predictions of molecular properties like binding affinity and reactivity. This opens doors to designing more effective drugs with higher precision.
Simulating Molecular Interactions with Quantum Algorithms
Several quantum algorithms are being developed and explored for molecular dynamics simulations. These algorithms aim to accurately predict the behavior of molecules over time, providing insights into their interactions with other molecules, including potential drug targets. The accuracy and efficiency of these simulations are crucial for understanding drug efficacy and toxicity.
Comparison of Quantum Algorithms for Molecular Dynamics
The Variational Quantum Eigensolver (VQE) and Quantum Approximate Optimization Algorithm (QAOA) are two prominent quantum algorithms used for molecular simulations. VQE is a hybrid quantum-classical algorithm that iteratively refines a wavefunction to find the ground state energy of a molecule. This ground state energy is directly related to the molecule’s stability and reactivity. QAOA, on the other hand, is designed to find approximate solutions to combinatorial optimization problems, which can be applied to finding optimal conformations of molecules.
While both aim to provide information about molecular properties, VQE is generally better suited for calculating ground state energies, while QAOA can be more efficient for finding optimal molecular structures. A direct comparison reveals that VQE offers higher accuracy for energy calculations, while QAOA can be faster for certain optimization tasks, especially in cases where finding the exact solution is not critical.
The choice between these algorithms depends heavily on the specific problem and the desired level of accuracy.
Hypothetical Quantum Algorithm for Predicting Drug Efficacy
A hypothetical quantum algorithm for predicting drug efficacy could combine VQE for calculating binding affinities with a quantum machine learning model to predict the overall efficacy. The algorithm would first use VQE to calculate the binding affinity of a drug candidate to its target protein. This binding affinity would then be fed into a quantum machine learning model trained on a dataset of known drugs and their efficacy.
The quantum machine learning model, possibly using a quantum neural network, could learn complex relationships between molecular properties and drug efficacy, providing a more accurate prediction than classical models. For instance, this could involve incorporating factors like drug metabolism and pharmacokinetic properties into the model for a more holistic prediction. This approach could significantly reduce the time and cost associated with drug development by identifying promising candidates earlier in the process.
Consider a hypothetical scenario involving a novel cancer drug candidate. The algorithm could predict its efficacy based on its binding affinity to a specific cancer protein, alongside its predicted metabolic stability and potential side effects, providing a comprehensive assessment of its therapeutic potential.
Quantum Machine Learning for Identifying Potential Drug Candidates
Quantum machine learning (QML) offers the potential to accelerate the identification of potential drug candidates. Classical machine learning algorithms are already used extensively in drug discovery, but QML algorithms could offer significant advantages in terms of speed and accuracy, especially when dealing with large and complex datasets. QML algorithms can analyze vast amounts of molecular data to identify patterns and relationships that are difficult or impossible to detect using classical methods.
This could involve analyzing large libraries of molecules to identify those with high probability of being effective drugs. For example, a QML algorithm could be trained on a dataset of known drug molecules and their corresponding biological activities, and then used to predict the activity of new molecules. This approach could significantly reduce the number of molecules that need to be synthesized and tested experimentally, saving time and resources.
Imagine a scenario where a pharmaceutical company is screening millions of compounds for a potential treatment for Alzheimer’s disease. A QML algorithm could significantly narrow down the search space, focusing on compounds with the highest likelihood of success based on learned patterns from previous successful drugs.
Quantum Computing for Drug Target Identification
Quantum computing offers a transformative potential in drug discovery, particularly in the challenging area of identifying novel drug targets. Traditional methods often struggle with the complexity of biological systems, leading to lengthy and expensive research processes. Quantum algorithms, however, possess the power to analyze vast datasets and model intricate molecular interactions with unprecedented speed and accuracy, potentially revolutionizing target identification.The application of quantum computing in this field focuses on leveraging its unique capabilities to overcome limitations inherent in classical approaches.
By exploiting quantum phenomena like superposition and entanglement, quantum computers can explore a much larger search space of potential drug targets more efficiently than classical computers. This allows for the investigation of complex interactions between proteins, genes, and metabolites, leading to the discovery of previously unknown targets for various diseases.
Quantum Algorithms for Target Identification
Several quantum algorithms show promise in accelerating drug target identification. Quantum machine learning algorithms, for example, can analyze large genomic and proteomic datasets to identify potential targets based on patterns and correlations that classical algorithms might miss. Quantum simulations can model the intricate interactions between drug candidates and target proteins with higher accuracy, enabling more precise prediction of efficacy and toxicity.
This allows researchers to prioritize promising candidates and reduce the need for extensive and costly experimental validation. Furthermore, quantum optimization algorithms can help in designing more efficient drug screening campaigns, streamlining the overall drug discovery process.
Challenges in Applying Quantum Computing to Target Identification, Quantum computing in drug discovery
Despite its potential, applying quantum computing to drug target identification faces several significant challenges. Currently, the availability of fault-tolerant quantum computers is limited. Existing quantum computers are relatively small and prone to errors, restricting the complexity of problems that can be tackled effectively. Developing efficient quantum algorithms specifically tailored for drug target identification requires significant expertise in both quantum computing and biology.
The translation of biological data into a format suitable for quantum algorithms is another hurdle, requiring specialized pre-processing techniques. Finally, the high cost of accessing and maintaining quantum computing resources remains a significant barrier to widespread adoption.
Comparison of Classical and Quantum Approaches
Method | Speed | Accuracy | Cost |
---|---|---|---|
Classical Target Identification (e.g., high-throughput screening, bioinformatics analysis) | Relatively slow; can take years | Moderate; often requires extensive experimental validation | High; involves significant experimental costs and labor |
Quantum Target Identification (e.g., quantum machine learning, quantum simulation) | Potentially much faster; could significantly reduce identification time | Potentially higher; more accurate modeling of complex interactions | Currently very high due to limited access and high cost of quantum computers; expected to decrease with technological advancements |
Quantum Computing for Drug Design and Optimization
Quantum computing offers a powerful new approach to drug design and optimization, surpassing the capabilities of classical computers in handling the complex calculations involved in molecular modeling and simulations. This allows for the exploration of a much larger chemical space and the identification of novel drug candidates with improved properties. By leveraging the principles of quantum mechanics, quantum algorithms can efficiently analyze and predict the behavior of molecules, leading to faster and more accurate drug development processes.Quantum computers can significantly accelerate the design of novel drug molecules by efficiently exploring vast chemical spaces.
Traditional methods often struggle with the computational complexity of simulating molecular interactions, limiting the number of potential drug candidates that can be evaluated. Quantum algorithms, however, can tackle these challenges more effectively. They can predict the binding affinities of drug candidates to target proteins with greater accuracy, leading to the identification of molecules with higher potency and selectivity. This allows researchers to focus on the most promising candidates, reducing the time and cost associated with drug development.
Quantum Algorithms for Drug Optimization
Several quantum algorithms are being explored for drug optimization and structure-activity relationship (SAR) studies. Variational Quantum Eigensolver (VQE) and Quantum Approximate Optimization Algorithm (QAOA) are prominent examples. VQE aims to find the ground state energy of a molecule, providing insights into its stability and reactivity. This information is crucial for optimizing the drug’s properties, such as its solubility, bioavailability, and metabolic stability.
QAOA, on the other hand, is used to find optimal configurations of molecules, assisting in the design of molecules with desired properties. These algorithms can be applied to optimize various aspects of drug molecules, including their shape, size, and chemical composition, leading to improved efficacy and reduced side effects. For example, QAOA could be used to optimize the binding affinity of a drug molecule to its target protein by exploring different conformations of the molecule.
Benefits and Limitations of Quantum Computing in Drug Design
The potential benefits of using quantum computing for drug design are substantial. It promises to accelerate the drug discovery process significantly, reducing the time and cost associated with bringing new drugs to market. This is particularly important for diseases with high unmet medical needs, where rapid development of effective treatments is crucial. Improved accuracy in predicting molecular properties can lead to the design of drugs with enhanced efficacy and reduced side effects, ultimately benefiting patients.
The ability to explore a much larger chemical space can also lead to the discovery of novel drug candidates that would be impossible to identify using classical methods.However, it is important to acknowledge the limitations. Quantum computers are still in their early stages of development, and their capabilities are limited by factors such as qubit coherence time and scalability.
The development of robust and efficient quantum algorithms specifically tailored for drug design is an ongoing process. Furthermore, the interpretation and validation of results obtained from quantum computations require careful consideration and validation using experimental data. The high cost of accessing and operating quantum computers also presents a significant barrier to widespread adoption. Despite these limitations, the potential benefits of quantum computing for drug design are significant, and ongoing research and development efforts are paving the way for its wider application in the pharmaceutical industry.
Quantum Computing for Drug Delivery and Pharmacokinetics
Quantum computing holds immense potential to revolutionize drug delivery and pharmacokinetics, areas currently hampered by the complexity of biological systems and the limitations of classical computational methods. By leveraging the power of quantum mechanics, we can simulate molecular interactions with unprecedented accuracy, leading to the design of more efficient and targeted drug delivery systems and a deeper understanding of how drugs behave within the body.The ability to predict drug pharmacokinetics (PK) and pharmacodynamics (PD) with greater precision is crucial for optimizing drug efficacy and minimizing adverse effects.
Quantum computing holds immense promise for revolutionizing drug discovery by simulating molecular interactions with unprecedented accuracy. However, realizing this potential hinges on advancements in Quantum AI hardware development and its challenges in scalability , specifically building stable and large-scale quantum computers. Overcoming these hardware limitations is crucial for unlocking the full therapeutic power of quantum simulations in drug design.
Quantum simulations can model the intricate processes involved in drug absorption, distribution, metabolism, and excretion (ADME), providing insights that are currently difficult or impossible to obtain using classical approaches. This improved understanding can significantly accelerate the drug development process and improve patient outcomes.
Quantum computing holds immense promise for revolutionizing drug discovery by simulating molecular interactions with unprecedented accuracy. However, realizing this potential requires overcoming significant hurdles, as highlighted in this insightful article: Exploring the limitations and challenges of current quantum AI algorithms. Understanding these limitations is crucial for developing more robust quantum algorithms capable of tackling the complexity of drug design and significantly accelerating the drug development process.
Quantum Simulations for Predicting Drug ADME
A hypothetical quantum simulation of drug ADME could begin by modeling the drug molecule and its interaction with the biological environment at the atomic level. This involves representing the drug molecule and relevant biological components (e.g., proteins, lipids, and water molecules) as quantum mechanical systems. Quantum algorithms, such as variational quantum eigensolver (VQE) or quantum approximate optimization algorithm (QAOA), can then be employed to calculate the energies and properties of these systems, providing information on the drug’s binding affinity to target sites, its solubility, and its permeability across biological membranes.
For example, a VQE algorithm could be used to determine the binding energy of a drug molecule to a transporter protein involved in drug absorption. This calculation would be significantly faster and more accurate than classical methods, especially for large and complex molecules. The simulation would then progress to model the drug’s distribution within the body, considering factors like blood flow, tissue permeability, and binding to plasma proteins.
Quantum algorithms could be used to simulate diffusion processes and predict drug concentrations in different tissues over time. Metabolism, involving the transformation of the drug molecule by enzymes, could be simulated by modeling the interactions between the drug and relevant enzymes. Finally, the simulation would model drug excretion, considering pathways like renal clearance and biliary excretion. The results of this comprehensive simulation would provide a detailed picture of the drug’s ADME profile, informing decisions on dosage, formulation, and route of administration.
This detailed understanding could help researchers predict potential drug-drug interactions or identify individuals who might be at higher risk of adverse effects due to specific genetic factors impacting their metabolism. For instance, simulating the metabolism of a drug by the cytochrome P450 enzyme family could identify potential drug-drug interactions that may not be apparent using traditional methods. This comprehensive approach offers the potential to significantly reduce the time and cost associated with drug development, while simultaneously improving the safety and efficacy of new medications.
Challenges and Future Directions
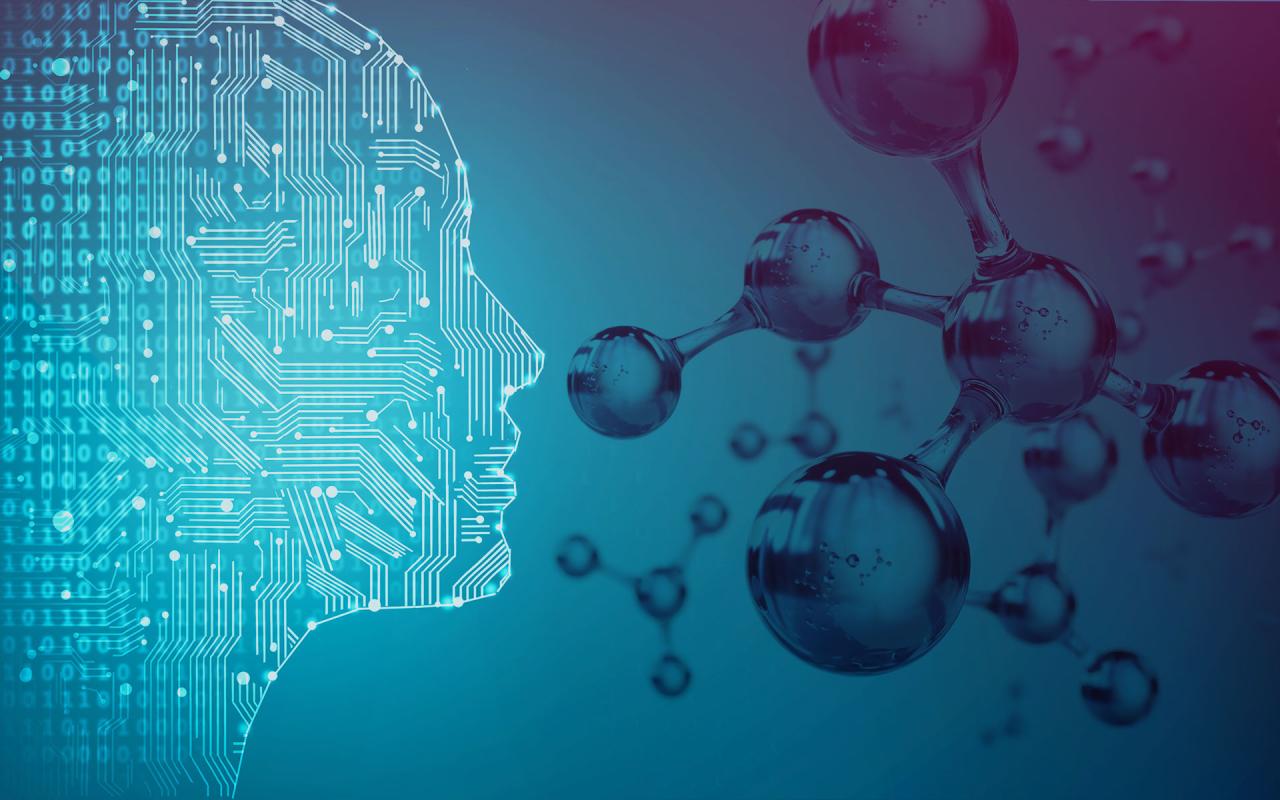
Source: 1qbit.com
Quantum computing holds incredible promise for accelerating drug discovery by simulating molecular interactions far beyond classical computers’ capabilities. This power extends beyond pharmaceuticals; consider the logistical challenges in drug distribution, where optimizing delivery routes is crucial. Learning about Quantum AI’s potential in solving optimization problems in logistics highlights how similar quantum approaches can revolutionize different fields.
Ultimately, these advancements in quantum computation will likely lead to faster development and more efficient delivery of life-saving medicines.
Quantum computing holds immense promise for revolutionizing drug discovery, but significant hurdles remain before it becomes a mainstream tool. Currently, the technology is in its nascent stages, and several key limitations hinder its widespread adoption in the pharmaceutical industry. Overcoming these challenges requires concerted efforts in hardware development, software optimization, and collaborative research between diverse scientific communities.The path to realizing the full potential of quantum computing in drug discovery necessitates addressing several critical bottlenecks.
These include the inherent fragility of quantum states, the limited qubit count and coherence times in current quantum computers, and the lack of readily available, user-friendly quantum algorithms specifically tailored for pharmaceutical applications. Furthermore, the high cost of quantum computing infrastructure and the specialized expertise required to operate and program these machines present significant barriers to entry for many pharmaceutical companies.
Technological Limitations of Quantum Computing in Drug Discovery
Current quantum computers suffer from several limitations that restrict their applicability in drug discovery. The number of qubits available is still relatively small compared to the complexity of many drug discovery problems. Furthermore, maintaining the coherence of quantum states—a crucial requirement for accurate computation—is challenging and significantly limits the length of computations that can be performed reliably. Noise and errors introduced during computation can also compromise the accuracy of results.
The development of error correction techniques is crucial for addressing this issue. Finally, the lack of standardized software and algorithms specifically designed for drug discovery applications hinders the wider adoption of quantum computing in this field. Existing algorithms often need adaptation and optimization for specific pharmaceutical problems.
Advancements in Quantum Hardware and Software
To facilitate widespread adoption of quantum computing in drug discovery, significant advancements are needed in both hardware and software. Hardware improvements should focus on increasing qubit count, extending coherence times, and reducing error rates. This could involve exploring new qubit technologies, such as topological qubits, or developing more sophisticated error correction codes. Simultaneously, software development must concentrate on creating more efficient and user-friendly quantum algorithms specifically designed for drug discovery tasks.
This includes developing algorithms that can handle noisy intermediate-scale quantum (NISQ) devices and translating classical algorithms into their quantum counterparts. The creation of standardized software tools and libraries would also greatly facilitate the adoption of quantum computing by pharmaceutical researchers. Furthermore, the development of quantum-classical hybrid algorithms, which combine the strengths of both classical and quantum computing, could provide a more practical approach in the near term.
Collaboration Between Quantum Computing Experts and Pharmaceutical Researchers
Successful integration of quantum computing into drug discovery requires strong collaborations between experts in quantum computing and pharmaceutical researchers. Quantum computing specialists can provide the necessary expertise in algorithm design, hardware optimization, and error mitigation. Pharmaceutical researchers, on the other hand, possess in-depth knowledge of drug discovery processes, biological systems, and relevant datasets. By combining their expertise, these collaborations can lead to the development of more effective and targeted quantum algorithms tailored to specific drug discovery challenges.
Joint research projects, workshops, and training programs can facilitate this collaboration and accelerate progress in the field. For instance, a collaboration could involve quantum computing experts developing algorithms for molecular dynamics simulations, while pharmaceutical researchers provide the necessary biological data and expertise to validate and interpret the results.
Potential Future Applications of Quantum Computing in Drug Discovery
The potential applications of quantum computing in drug discovery extend far beyond the areas already discussed. The following list highlights some promising future avenues:
- Personalized medicine: Quantum algorithms could enable the development of personalized treatments based on an individual’s genetic makeup and disease profile.
- Predicting drug efficacy and toxicity: Quantum simulations could accurately predict the efficacy and toxicity of drug candidates before expensive and time-consuming clinical trials.
- Accelerated drug repurposing: Quantum computing could help identify existing drugs with potential for treating new diseases, significantly reducing development time and costs.
- Discovery of novel drug targets: Quantum machine learning algorithms could identify novel drug targets by analyzing vast biological datasets more efficiently than classical methods.
- Development of advanced drug delivery systems: Quantum simulations could optimize drug delivery systems to improve efficacy and reduce side effects.
Case Studies and Examples
While quantum computing is still in its early stages, several promising applications in drug discovery are emerging. These examples showcase the potential of quantum algorithms to tackle complex problems that are intractable for classical computers, leading to faster and more efficient drug development. The following case studies highlight specific instances where quantum approaches are making a difference.
Quantum Simulation of Molecular Properties for Cancer Drug Design
Quantum computers excel at simulating the behavior of molecules, a crucial step in drug discovery. Accurately predicting the properties of molecules, such as their binding affinity to a target protein, is essential for designing effective drugs. One area where this is particularly impactful is cancer research. Classical methods struggle to simulate large, complex molecules involved in cancer pathways.
Quantum computing holds immense promise for revolutionizing drug discovery by simulating molecular interactions with unprecedented accuracy. However, harnessing this power requires incredibly fast data transfer, which is why advancements in Low-latency communication are crucial. Without near-instantaneous communication, the potential of quantum computers in this field would be significantly hampered, slowing down the process of developing life-saving medications.
Quantum simulations, however, can model these interactions with greater accuracy, potentially leading to the identification of novel cancer drugs with improved efficacy and reduced side effects. For example, researchers are exploring the use of quantum algorithms like Variational Quantum Eigensolver (VQE) to simulate the interaction between a potential cancer drug molecule and a specific cancer-related protein. By accurately predicting the binding energy, researchers can assess the drug’s potential effectiveness and optimize its design for stronger binding and improved therapeutic outcomes.
The increased accuracy provided by quantum simulation allows for a more targeted and efficient drug design process, reducing the time and resources required for traditional trial-and-error approaches.
Quantum Machine Learning for Identifying Antibiotic Drug Targets
The rise of antibiotic resistance is a significant global health concern. Identifying new drug targets for combating resistant bacteria is a challenging task. Quantum machine learning (QML) algorithms offer a potential solution. Traditional machine learning methods often struggle with the high dimensionality and complexity of biological data related to bacterial resistance mechanisms. QML algorithms, however, can leverage the power of quantum computers to analyze large datasets and identify subtle patterns indicative of potential drug targets.
A hypothetical example involves using a quantum support vector machine (Q-SVM) to analyze genomic data from antibiotic-resistant bacteria. The Q-SVM could identify specific genes or proteins associated with resistance, which could then be targeted by novel antibiotics. The improved accuracy and efficiency of Q-SVM compared to classical SVM could accelerate the identification of new drug targets, potentially leading to the development of more effective antibiotics to combat drug-resistant infections.
This faster identification of targets could significantly reduce the time it takes to develop new antibiotics, combating the growing threat of antibiotic resistance.
Closing Summary: Quantum Computing In Drug Discovery
In conclusion, quantum computing holds immense promise for transforming drug discovery. While still in its early stages, the potential benefits are undeniable. The ability to simulate complex molecular interactions, design novel drugs with enhanced efficacy and reduced side effects, and optimize drug delivery systems offers a transformative path forward. Overcoming the current technological hurdles through continued research and collaboration will unlock the full potential of this revolutionary technology, leading to faster development of life-saving medications and ultimately, a healthier future for all.
Commonly Asked Questions
What are the main limitations of classical computing in drug discovery?
Classical computers struggle with the complexity of simulating molecular interactions, especially for large molecules. This limits their ability to accurately predict drug efficacy and design novel drugs efficiently.
How much will quantum computing cost the pharmaceutical industry?
The cost is currently high due to the specialized hardware and expertise required. However, costs are expected to decrease as the technology matures and becomes more widely available.
When will quantum computers be widely used in drug discovery?
Widespread adoption is still some years away, but significant progress is being made. We can expect to see increasing applications in the next decade as quantum computing technology advances.
Are there ethical concerns surrounding the use of quantum computing in drug discovery?
Ethical considerations, such as equitable access to new drugs developed using quantum computing and potential misuse of the technology, need careful consideration and proactive mitigation strategies.
What types of diseases are most likely to benefit from quantum computing-aided drug discovery?
Diseases involving complex molecular interactions, such as cancer, neurodegenerative disorders, and infectious diseases, are prime candidates to benefit from quantum computing’s enhanced simulation and modeling capabilities.