Edge Computing and 5G A Powerful Synergy
Edge computing and 5G are revolutionizing how we process and access data. Imagine a world where self-driving cars navigate flawlessly, smart cities respond instantly to traffic congestion, and industrial robots work with unprecedented precision – all thanks to the lightning-fast speeds and reduced latency of 5G networks combined with the efficient processing power of edge computing. This powerful pairing is transforming industries, from manufacturing and healthcare to transportation and entertainment, by bringing computation closer to the data source, enabling real-time responses and unlocking unprecedented capabilities.
This synergy allows for applications previously impossible with cloud-based systems alone. The low latency of 5G provides the necessary speed for time-sensitive tasks, while edge computing handles the processing locally, minimizing delays and maximizing efficiency. This combination is particularly impactful in areas demanding immediate responses, such as autonomous driving, remote surgery, and real-time video analytics. The following sections will explore these applications in detail, delving into the architectural considerations and challenges of this transformative technology.
Introduction to Edge Computing and 5G Synergy
Edge computing and 5G represent a powerful technological synergy, transforming how we process and access data. By bringing computation closer to the data source, edge computing addresses the limitations of relying solely on centralized cloud infrastructure, while 5G’s high bandwidth and low latency provide the ideal network backbone for this decentralized approach. This combination unlocks new possibilities across various industries.
Fundamental Principles of Edge Computing
Edge computing shifts processing and data storage away from centralized cloud data centers to the network’s edge, closer to where data is generated. This reduces latency, improves bandwidth efficiency, and enhances data security by minimizing the distance data needs to travel. Key aspects include localized processing, reduced reliance on cloud connectivity, and improved real-time responsiveness. Think of it as bringing the power of the cloud to the devices and locations that need it most.
Key Characteristics of 5G Networks
G networks are characterized by significantly higher speeds, lower latency, and increased network capacity compared to previous generations. These improvements are driven by advancements in radio technologies, network architecture, and spectrum allocation. The lower latency is particularly crucial for applications requiring real-time responsiveness, such as autonomous vehicles and remote surgery. The increased bandwidth enables the transmission of large volumes of data, supporting applications like augmented reality and high-definition video streaming.
Synergistic Relationship Between Edge Computing and 5G
The synergy between edge computing and 5G is profound. 5G’s high bandwidth and low latency provide the necessary infrastructure to support the data-intensive requirements of edge computing applications. Conversely, edge computing leverages 5G’s capabilities to process data locally, reducing the reliance on cloud resources and improving response times. This mutual benefit leads to more efficient, responsive, and scalable systems.
Edge computing and 5G’s low latency are game-changers for data-intensive applications. This speed is crucial for processing the massive datasets involved in Quantum AI applications in drug discovery and development , allowing for faster simulations and more efficient drug design. Ultimately, the advancements in edge computing and 5G will accelerate breakthroughs in this exciting field.
For instance, a self-driving car relies on 5G for high-speed data transmission and edge computing for immediate processing of sensor data to avoid accidents.
Examples of Industries Where This Synergy is Particularly Impactful
The combination of edge computing and 5G is revolutionizing numerous industries. Here are a few examples:
Industry | Cloud Latency | Edge Latency | Bandwidth Difference |
---|---|---|---|
Autonomous Vehicles | 100-200ms | 10-20ms | Significant increase in bandwidth for sensor data |
Smart Manufacturing | 50-100ms | 5-10ms | Higher bandwidth for real-time machine control and data analytics |
Healthcare (Remote Surgery) | >100ms (unacceptable) | <20ms (critical) | High bandwidth for high-resolution video and data transmission |
Smart Cities (Traffic Management) | 50-100ms | 10-20ms | Increased bandwidth for real-time traffic monitoring and control |
Applications of Edge Computing in 5G Environments
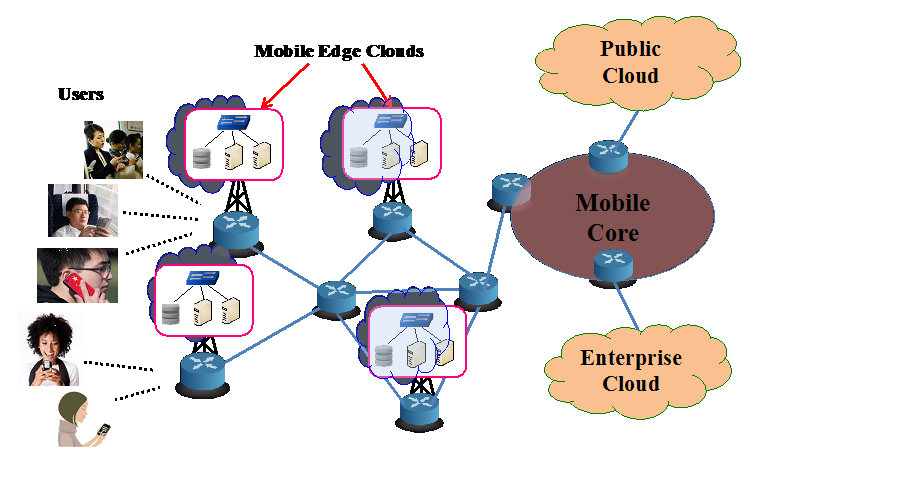
Source: ieee.org
The synergy between 5G’s high bandwidth and low latency and edge computing’s localized processing power unlocks a wide array of applications previously constrained by network limitations. This combination allows for real-time data analysis and faster response times, significantly improving efficiency and enabling entirely new functionalities across various sectors.Edge computing processes data closer to its source, reducing the reliance on cloud infrastructure and mitigating latency issues inherent in transmitting large amounts of data over long distances.
This proximity to data sources is particularly beneficial in applications demanding immediate responses, such as those found in smart cities, autonomous vehicles, and industrial automation.
Real-Time Video Analytics in Smart Cities
Real-time video analytics powered by edge computing transforms how smart cities manage traffic, monitor public safety, and optimize resource allocation. Edge devices, strategically placed throughout the city, process video streams from surveillance cameras, identifying events like traffic congestion, accidents, or suspicious activities in real-time. This immediate processing eliminates the delays associated with sending video data to a remote cloud server for analysis.
The results are then used to dynamically adjust traffic signals, dispatch emergency services, or enhance public safety measures. For example, a system might automatically detect a traffic jam and reroute traffic to alleviate congestion, or identify a potential security threat and alert law enforcement.
Edge Computing in Autonomous Vehicle Navigation with 5G Connectivity
Autonomous vehicles rely heavily on real-time data processing for navigation and obstacle avoidance. 5G’s high bandwidth and low latency provide the necessary communication infrastructure, while edge computing enables the rapid processing of sensor data crucial for safe and efficient driving. Data from various sensors (LiDAR, radar, cameras) is processed at the edge, allowing the vehicle to make immediate decisions based on its immediate surroundings.
This reduces reliance on cloud-based processing, which could introduce unacceptable delays that compromise safety. The edge computing system can identify obstacles, predict pedestrian movements, and adjust the vehicle’s path accordingly, all within milliseconds. A hypothetical scenario might involve a sudden pedestrian crossing; edge processing allows the vehicle to brake immediately, preventing an accident.
Enhanced Performance of IoT Devices within a 5G Network
The massive number of IoT devices generating data in a 5G environment presents a significant challenge for network bandwidth and data processing. Edge computing addresses this by offloading processing tasks from the devices themselves and the core network. This reduces the strain on the network and improves the overall responsiveness of the IoT devices. For instance, in a smart agriculture setting, sensors monitor soil conditions, temperature, and humidity.
Edge computing processes this data locally, identifying trends and triggering automated actions like irrigation or fertilizer application, without requiring constant communication with a distant cloud server. This approach enhances battery life, reduces network congestion, and ensures faster response times to changing environmental conditions.
Edge Computing in Industrial Automation
Edge computing plays a vital role in optimizing industrial automation processes, particularly in scenarios requiring real-time data processing and control. Consider a smart factory environment where numerous machines generate vast amounts of sensor data. Implementing edge computing at the factory floor enables real-time monitoring and control of these machines.Here’s a typical data flow and processing scenario:
- Data Acquisition: Sensors on various machines collect data on parameters like temperature, pressure, and vibration.
- Edge Processing: An edge server located within the factory processes this data in real-time, identifying anomalies or potential malfunctions.
- Real-time Control: Based on the processed data, the edge server adjusts machine parameters or triggers alerts to prevent failures or optimize performance.
- Data Aggregation: Aggregated data is then sent to a central cloud server for further analysis and long-term trend identification.
- Predictive Maintenance: The analysis of historical and real-time data allows for predictive maintenance, reducing downtime and optimizing maintenance schedules.
This approach enables proactive maintenance, improved production efficiency, and reduced operational costs. For example, early detection of a machine malfunction through edge processing allows for timely intervention, preventing costly production downtime.
Architectural Considerations for Edge Computing with 5G
Deploying edge computing effectively within a 5G network requires careful consideration of the architecture. The choice of architecture significantly impacts performance, scalability, and cost. Understanding the key components and addressing security concerns are crucial for successful implementation.
Comparison of Edge Computing Architectures
Fog computing and mobile edge computing (MEC) are two prominent edge computing architectures often used with 5G. While both aim to process data closer to the source, they differ in their scope and deployment. Fog computing encompasses a broader range of network edge locations, including data centers and enterprise networks, creating a distributed layer between the cloud and end devices.
MEC, on the other hand, focuses specifically on the radio access network (RAN) edge, placing computing resources closer to mobile devices for low-latency applications. The choice between them depends on the specific application requirements and the desired level of proximity to the data source. For example, a real-time video analytics application might benefit from the extremely low latency offered by MEC, while a less latency-sensitive application like remote sensor data aggregation might be more suitably deployed in a fog computing environment.
Key Components of a 5G-Enabled Edge Computing System
A typical 5G-enabled edge computing system comprises several interconnected components. These include the 5G RAN, edge data centers or MEC servers located within or near the RAN, the core network, the cloud, and the end devices (e.g., smartphones, IoT sensors). High-speed, low-latency 5G connectivity acts as the backbone, enabling seamless communication between these components. Sophisticated orchestration and management software are also essential for efficient resource allocation and service provisioning.
For instance, a smart city application might use sensors deployed throughout the city (end devices) to collect traffic data, which is then processed at nearby MEC servers (edge data centers) to optimize traffic flow in real-time. This processed data might then be sent to a central cloud server for long-term storage and analysis.
The Role of Network Slicing in Optimizing Edge Computing Deployments, Edge computing and 5G
Network slicing allows the creation of virtual networks within the 5G infrastructure, each tailored to specific application requirements. This is vital for edge computing because different applications have varying demands on bandwidth, latency, and security. For example, a slice dedicated to autonomous vehicles would prioritize low latency and high reliability, while a slice for video streaming might prioritize high bandwidth.
By creating dedicated slices, network resources are optimized for each application, ensuring optimal performance and preventing resource contention. This results in a more efficient and effective edge computing deployment, allowing multiple applications to coexist and function optimally without impacting one another.
Security Challenges and Solutions in Edge Computing with 5G
The distributed nature of edge computing introduces new security challenges. Protecting data and resources at multiple edge locations requires a multi-layered security approach.
Security Challenge | Mitigation Strategy | Implementation Details | Potential Impact |
---|---|---|---|
Data breaches at edge locations | Robust access control and encryption | Implementing strong authentication mechanisms, encrypting data both in transit and at rest, using intrusion detection systems. | Loss of sensitive data, reputational damage, financial losses |
Denial-of-service (DoS) attacks | Distributed denial-of-service (DDoS) mitigation techniques | Employing techniques such as rate limiting, traffic filtering, and using cloud-based DDoS protection services. | System unavailability, disruption of services |
Compromised edge devices | Secure device management and firmware updates | Regularly updating firmware, implementing secure boot processes, and using remote device management tools. | Data breaches, unauthorized access, malware propagation |
Lack of visibility and control across distributed edge locations | Centralized security management and monitoring | Implementing a centralized security information and event management (SIEM) system to monitor and manage security across all edge locations. | Delayed detection of security incidents, ineffective response to threats |
Challenges and Opportunities in Edge Computing and 5G Integration
The convergence of edge computing and 5G presents a powerful synergy, but realizing its full potential requires addressing significant challenges while capitalizing on exciting opportunities. Successfully navigating these complexities will be key to unlocking the transformative capabilities of this technological pairing. This section explores the hurdles and potential rewards associated with integrating edge computing and 5G.
Managing and Maintaining Distributed Edge Computing Resources
Managing a distributed network of edge computing resources introduces considerable complexity. Unlike centralized data centers, edge deployments are geographically dispersed, often involving diverse hardware and software configurations from multiple vendors. This heterogeneity necessitates robust management tools capable of monitoring performance, provisioning resources dynamically, and ensuring security across the entire network. Furthermore, the remote nature of many edge locations can complicate maintenance and troubleshooting, requiring innovative remote management solutions and potentially higher operational costs.
Effective resource allocation strategies, predictive maintenance, and automated responses to failures are crucial for maintaining system uptime and efficiency.
Ensuring Data Consistency and Integrity in a Distributed Environment
Maintaining data consistency and integrity across a distributed edge computing network is a significant challenge. Data replication and synchronization strategies are critical to ensuring that all edge nodes possess the most up-to-date information. However, the inherent latency and bandwidth limitations of communication links between edge nodes and the core network can impact the speed and reliability of data synchronization.
Edge computing and 5G’s low latency are crucial for handling the massive data streams generated by climate modeling. The speed and efficiency offered by these technologies are vital to support complex simulations, especially when considering Quantum AI’s potential to solve complex climate change modeling problems. Ultimately, the power of edge computing and 5G will be key to processing and acting upon the insights gained from these advanced simulations.
Robust mechanisms for conflict resolution and data validation are essential to prevent data corruption and ensure data accuracy. This often requires the implementation of sophisticated algorithms and protocols to handle potential inconsistencies and ensure data integrity in a dynamic environment.
Opportunities for Innovation in Edge Computing and 5G Services and Applications
The combination of 5G’s high bandwidth and low latency with the processing power of edge computing unlocks a wide range of innovative services and applications. The ability to process data closer to the source dramatically reduces latency, enabling real-time applications previously impossible with cloud-based architectures. This opens up opportunities for advancements in various sectors, leading to new business models and revenue streams.
Edge computing and 5G are revolutionizing data processing by bringing computation closer to the source. This rapid data processing is crucial, especially considering the massive computational demands of advanced AI algorithms. For a deeper dive into the computational limits and possibilities, check out this article on Understanding the quantum supremacy debate in the context of AI , which explores potential breakthroughs that could impact future edge deployments.
Ultimately, advancements in both areas are essential for creating truly responsive and intelligent systems.
For example, improved capabilities in areas such as augmented reality (AR) and virtual reality (VR) experiences, advanced robotics, and autonomous vehicles become readily available.
Addressing the Growing Demand for Low-Latency Applications
Advancements in 5G and edge computing are directly addressing the escalating demand for low-latency applications. The reduced distance data travels for processing translates to significantly faster response times, which is crucial for applications requiring immediate feedback.
Edge computing and 5G are poised to revolutionize data processing, bringing powerful computation closer to the source. However, even with this enhanced speed and efficiency, we still face hurdles in truly harnessing AI’s potential, as highlighted in this article exploring the limitations and challenges of current quantum AI algorithms: Exploring the limitations and challenges of current quantum AI algorithms.
Overcoming these quantum computing limitations will be key to unlocking the full potential of future edge applications powered by 5G.
- Autonomous Vehicles: Real-time processing of sensor data for collision avoidance and navigation.
- Remote Surgery: Enabling surgeons to perform complex procedures remotely with minimal latency.
- Industrial Automation: Real-time control of robots and machinery in manufacturing environments.
- Augmented Reality/Virtual Reality: Seamless and responsive interactive experiences without noticeable delays.
- Smart Cities: Real-time traffic management, environmental monitoring, and public safety applications.
Future Trends and Developments
The convergence of 5G and edge computing is poised for explosive growth, driven by advancements in both technologies and the increasing demand for real-time, low-latency applications. Looking ahead, we can anticipate significant developments shaping the future landscape of this powerful synergy.The evolution of these technologies will be intertwined, each pushing the other forward in a positive feedback loop. This section will explore the anticipated advancements in 5G technology, edge computing hardware and software, and the transformative role of AI and machine learning.
5G Technological Advancements
G is not a static technology; continuous improvements are expected. We’ll see the rollout of 5G-Advanced, focusing on enhanced capacity, improved network efficiency, and lower latency. This includes wider adoption of higher frequency bands (like mmWave) for increased bandwidth, along with more sophisticated network slicing to better allocate resources for specific applications. For example, dedicated slices for autonomous vehicles will prioritize low latency and high reliability, while slices for entertainment streaming will prioritize high bandwidth.
Furthermore, the integration of 6G technologies is likely to begin, with research and development already underway exploring even higher frequencies and more advanced antenna technologies. This will lay the groundwork for seamless integration with future edge computing deployments.
Advancements in Edge Computing Hardware and Software
Edge computing hardware will become more powerful, energy-efficient, and compact. We can expect to see the proliferation of specialized hardware accelerators, such as AI processors and FPGAs, optimized for specific tasks like video processing or machine learning inference. This will enable more complex computations to be performed at the edge, reducing the reliance on cloud data centers. Software advancements will focus on improved orchestration and management tools, allowing for easier deployment and scaling of edge applications.
Containerization and serverless computing will become increasingly prevalent, simplifying the development and deployment process. Furthermore, improved security protocols will be essential to address the inherent security challenges of distributing computing resources across geographically dispersed locations. For instance, imagine a smart city using a distributed network of edge devices to manage traffic flow; robust security is paramount to prevent disruptions or malicious attacks.
Impact of AI and Machine Learning on Edge Computing within 5G Networks
The integration of AI and machine learning (ML) will be transformative for edge computing in 5G environments. AI/ML algorithms can be deployed at the edge to process data locally, enabling real-time decision-making and reducing latency. This is crucial for applications such as autonomous driving, industrial automation, and real-time video analytics. For example, a network of smart cameras at a factory floor could use edge AI to detect defects in products immediately, without needing to send data to a distant cloud server.
Furthermore, AI/ML can be used to optimize network performance, predict network failures, and improve resource allocation within the 5G network itself. This will lead to more efficient and reliable networks. The increasing availability of powerful, low-power AI chips specifically designed for edge deployments will be a key enabler of this trend.
Hypothetical Future Edge Computing and 5G Infrastructure
Imagine a sprawling metropolis. The core infrastructure consists of a network of 5G base stations strategically positioned throughout the city, forming a high-bandwidth, low-latency network. These base stations are interconnected through a high-capacity fiber optic backbone. Distributed across the city are numerous edge data centers, smaller and more localized than traditional cloud data centers. These edge data centers house powerful servers equipped with AI accelerators and specialized hardware for specific applications.
Each edge data center is connected to multiple 5G base stations, forming a mesh-like network. Furthermore, smaller edge nodes are deployed closer to the source of data, such as smart cameras on street corners or sensors in industrial facilities. These nodes pre-process data before sending it to the larger edge data centers or the cloud, minimizing latency and bandwidth consumption.
The interaction between these elements is seamless and dynamic, with AI algorithms constantly monitoring and optimizing network performance and resource allocation based on real-time demand. For instance, during a major event, the system automatically adjusts resource allocation to prioritize applications related to crowd management and emergency response. This entire infrastructure is managed by a sophisticated orchestration system that ensures optimal performance and security.
Final Conclusion
The convergence of edge computing and 5G marks a significant leap forward in technological capabilities. By bringing processing power closer to the data source, we unlock a new era of real-time applications and unprecedented efficiency. While challenges remain in areas like security and resource management, the potential benefits – from smarter cities to more responsive industrial automation – are immense.
As 5G networks mature and edge computing technologies advance, we can anticipate even more innovative applications and a profound transformation across numerous industries, paving the way for a truly connected and intelligent future.
FAQ Overview: Edge Computing And 5G
What are the main security concerns with edge computing and 5G?
Increased attack surface due to distributed nature of edge devices, data breaches from compromised edge nodes, and potential for denial-of-service attacks are primary concerns. Robust security measures at each layer are crucial.
How does edge computing differ from cloud computing?
Cloud computing processes data in centralized data centers, while edge computing processes data closer to the source (e.g., on devices or at the network edge). Edge computing prioritizes low latency and reduced bandwidth usage.
What is network slicing in the context of 5G and edge computing?
Network slicing allows for the creation of virtual networks within a 5G network, optimizing resources for specific applications (e.g., dedicated slices for autonomous vehicles or industrial IoT). This improves efficiency and resource allocation for edge computing deployments.
What are some examples of new services enabled by this technology?
Examples include augmented reality applications with real-time responses, advanced remote monitoring and control systems, highly responsive smart home systems, and improved traffic management in smart cities.