AI Ethics and Bias A Critical Examination
AI ethics and bias represent a critical juncture in technological advancement. The increasing integration of artificial intelligence into various societal aspects necessitates a thorough understanding of its potential for perpetuating and amplifying existing biases. This exploration delves into the multifaceted nature of AI bias, examining its origins, impacts, and potential mitigation strategies, ultimately aiming to foster responsible AI development and deployment.
From algorithmic biases embedded within code to data biases reflecting societal inequalities, the consequences of unchecked AI bias are far-reaching. We will analyze how these biases disproportionately affect marginalized communities, leading to unfair or discriminatory outcomes in areas such as loan applications, criminal justice, and healthcare. The discussion will also cover ethical frameworks, mitigation techniques, and the future trajectory of responsible AI innovation.
Defining AI Bias
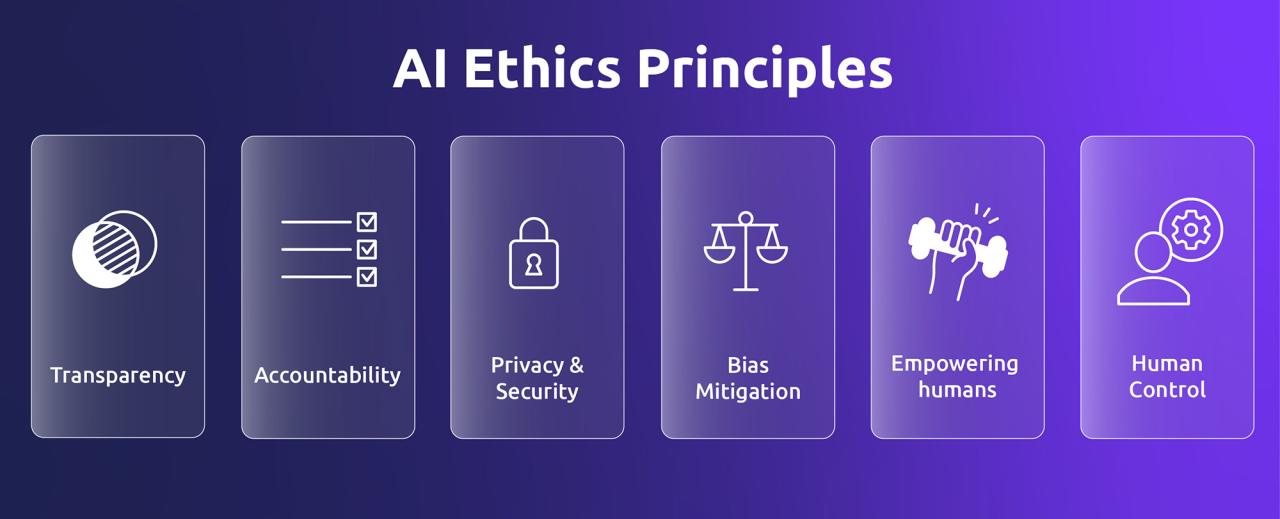
Source: diabolocom.com
AI bias refers to systematic and repeatable errors in a computer system that create unfair or discriminatory outcomes, often reflecting and amplifying existing societal biases. It’s crucial to understand that AI systems, despite their seemingly objective nature, are not immune to human prejudices. These biases can manifest in various forms, significantly impacting the fairness and equity of AI-driven decisions.AI bias is not a single entity but rather a multifaceted problem stemming from various sources.
Understanding its different forms and origins is essential to mitigating its harmful effects. The consequences of biased AI can range from minor inconveniences to severe injustices, depending on the context and the severity of the bias.
Algorithmic Bias
Algorithmic bias arises from the design and implementation of the algorithms themselves. This can involve flawed logic, biased assumptions embedded in the code, or the unintentional reinforcement of existing societal stereotypes through the algorithm’s decision-making process. For example, an algorithm designed to assess loan applications might inadvertently favor applicants from certain demographic groups due to the way features are weighted or thresholds are set, even if these factors are not explicitly included in the algorithm’s design.
This bias might not be immediately apparent, making it particularly insidious. Careful scrutiny of the algorithm’s logic and decision pathways is crucial to identify and rectify such biases.
Data Bias
Data bias, arguably the most prevalent form, occurs when the data used to train an AI system is not representative of the real-world population or contains inherent biases. This biased data can reflect historical inequalities, societal prejudices, or simply incomplete or skewed sampling methods. For instance, if a facial recognition system is trained primarily on images of individuals with lighter skin tones, it will likely perform poorly on individuals with darker skin tones, leading to inaccurate and potentially discriminatory outcomes.
The quality and diversity of the training data are paramount to ensuring fairness and accuracy.
Societal Bias
Societal bias represents the broader societal prejudices and inequalities that are reflected in the data and algorithms. These biases are often deeply ingrained and can be difficult to detect and address. For example, an AI system used in criminal justice might inadvertently perpetuate existing racial disparities in sentencing if the training data reflects historical biases in arrest rates or convictions.
Addressing societal bias requires a multifaceted approach that goes beyond simply adjusting algorithms or datasets; it requires confronting the underlying societal inequalities that fuel these biases.
Comparison of Bias Types
The different types of AI bias are interconnected and often reinforce each other. Algorithmic bias can amplify data bias, and both can perpetuate societal bias. While algorithmic bias focuses on the flaws within the algorithm’s logic, data bias highlights the skewed representation in the training data. Societal bias, on the other hand, represents the larger societal context that influences both the data and the algorithms.
Understanding these interconnections is crucial for developing effective strategies to mitigate AI bias.
Hypothetical Scenario: Biased Hiring Algorithm
Imagine a company uses an AI system to screen job applications. The system is trained on historical hiring data, which reflects a past where a disproportionate number of men were hired for technical roles. Consequently, the AI learns to associate certain s and phrases in resumes (e.g., “competitive sports,” “military experience”) more strongly with male applicants. As a result, the AI system consistently ranks male applicants higher, even if female applicants possess equivalent or superior qualifications.
This leads to a discriminatory outcome, perpetuating gender inequality in the workplace, despite the absence of any explicit gender-based criteria in the algorithm itself. This illustrates how seemingly neutral algorithms can reinforce and amplify existing biases.
Impact of AI Bias on Different Groups
AI bias, stemming from flawed data or algorithms, doesn’t affect everyone equally. Marginalized and vulnerable populations disproportionately experience the negative consequences of biased AI systems, leading to unfair or discriminatory outcomes in various aspects of their lives. Understanding this unequal impact is crucial for mitigating harm and ensuring equitable access to AI-driven technologies.
AI bias manifests in various ways, often reflecting and amplifying existing societal prejudices. These biases can be subtle or overt, leading to significant disparities in areas like loan applications, criminal justice, hiring processes, and even healthcare. The lack of diversity in the datasets used to train AI models often contributes to this problem, resulting in systems that perform poorly or unfairly for certain demographic groups.
Examples of AI Bias Against Specific Demographic Groups
Numerous examples illustrate how AI systems can perpetuate and exacerbate societal biases. Facial recognition technology, for instance, has been shown to exhibit higher error rates for individuals with darker skin tones, leading to misidentification and potential miscarriages of justice. Similarly, recruitment tools trained on historical data reflecting gender imbalances in certain industries may inadvertently discriminate against female applicants. In the healthcare sector, algorithms used for risk assessment or treatment recommendations have been found to produce biased results based on race or socioeconomic status.
These instances highlight the urgent need for greater transparency and accountability in the development and deployment of AI systems.
Case Studies Illustrating Real-World Consequences
The COMPAS (Correctional Offender Management Profiling for Alternative Sanctions) system, used in the US criminal justice system to predict recidivism, provides a stark example of biased AI in action. Studies revealed that COMPAS was more likely to incorrectly flag Black defendants as high-risk compared to white defendants, leading to potentially harsher sentencing and a perpetuation of racial disparities within the justice system.
Another example involves loan applications, where AI-powered systems trained on biased data have historically denied loans to individuals from low-income neighborhoods or specific racial groups, exacerbating existing economic inequalities. These real-world consequences underscore the importance of addressing AI bias to prevent further societal harm.
Table of AI Bias Effects on Different Demographic Groups
Demographic Group | Type of Bias | System Affected | Consequence |
---|---|---|---|
Black Individuals | Racial Bias | Facial Recognition Software | Higher rates of misidentification, leading to potential wrongful arrests or accusations. |
Women | Gender Bias | Recruitment AI | Lower likelihood of being shortlisted for job applications, perpetuating gender inequality in the workplace. |
Low-Income Individuals | Socioeconomic Bias | Loan Approval Algorithms | Reduced access to credit, hindering financial stability and opportunity. |
Elderly Individuals | Age Bias | Healthcare Risk Assessment Tools | Inadequate or inappropriate healthcare recommendations based on ageist assumptions. |
Mitigation Strategies for AI Bias
Mitigating bias in AI systems is crucial for ensuring fairness, accountability, and trustworthiness. This requires a multifaceted approach, implemented throughout the entire AI lifecycle, from data collection to deployment and monitoring. Effective bias mitigation involves careful consideration of data representation, algorithmic design, and ongoing evaluation.
Addressing AI bias necessitates a proactive and iterative process. It’s not a one-time fix but rather an ongoing commitment to improving fairness and equity. Strategies must be tailored to the specific context and type of bias identified, requiring a combination of technical and societal solutions.
Data Preprocessing Techniques
Data preprocessing plays a vital role in mitigating bias. Techniques employed at this stage aim to identify and correct imbalances or distortions in the data that could lead to biased outcomes. This involves various methods that can improve the quality and representativeness of the dataset.
Several methods are commonly used. These include data augmentation, which involves creating synthetic data to balance underrepresented groups. Data cleaning focuses on identifying and removing erroneous or irrelevant data points. Re-weighting techniques adjust the importance of different data points to account for class imbalances. Finally, data transformation methods can alter the data’s representation to reduce the impact of biased features.
Algorithmic Adjustments
Algorithmic fairness is a critical aspect of bias mitigation. Modifying algorithms to explicitly address fairness considerations is vital for ensuring equitable outcomes. This involves carefully designing the algorithm to minimize discriminatory effects and promote fair decision-making.
Several algorithmic adjustments can be made. Fairness-aware algorithms are designed with fairness constraints built-in. These constraints aim to balance accuracy with fairness considerations. Another technique is adversarial debiasing, where a separate model is trained to detect and counteract biases in the main model. Regularization techniques can also help to prevent overfitting and reduce the influence of biased features.
Ensemble methods can combine multiple models, each trained on different subsets of the data, to mitigate bias.
Bias Detection and Mitigation Methods
Numerous methods exist for detecting and mitigating bias in AI systems. These range from statistical analysis of datasets to specialized algorithms designed to identify and correct discriminatory patterns. The choice of method depends on the specific type of bias and the context of the application.
Statistical methods, such as analyzing group-specific performance metrics, can reveal disparities in how the AI system treats different groups. Explainable AI (XAI) techniques help to understand the decision-making process of the AI system, allowing for the identification of biased features or patterns. Adversarial attacks can be used to identify vulnerabilities in the AI system that might lead to biased outcomes.
Finally, ongoing monitoring and evaluation of the AI system’s performance are essential to detect and address emerging biases over time.
A Step-by-Step Process for Identifying and Addressing Bias in AI Development
A systematic approach is crucial for effectively identifying and addressing bias in the AI development lifecycle. This involves a series of steps, from initial data collection to ongoing monitoring and evaluation. A well-defined process ensures that bias is addressed proactively and systematically.
The following flowchart Artikels a typical process:
[Imagine a flowchart here. The flowchart would begin with “Data Collection and Preparation,” branching to “Data Analysis and Bias Detection” (including techniques like statistical analysis and visualization). This would then lead to “Bias Mitigation Strategies” (including data preprocessing, algorithmic adjustments, and fairness-aware model training). Next, it would proceed to “Model Evaluation and Testing,” focusing on fairness metrics.
Finally, it would end with “Deployment and Monitoring,” highlighting continuous evaluation and iterative improvements.]
Ethical Frameworks for AI Development
Developing and deploying artificial intelligence responsibly requires a robust ethical foundation. Numerous frameworks and guidelines exist, each offering a unique perspective on how to navigate the complex ethical challenges posed by AI. These frameworks aim to ensure that AI systems are developed and used in a way that benefits humanity while minimizing potential harms.Existing ethical frameworks provide a range of principles and guidelines for responsible AI development.
These frameworks often overlap and complement each other, offering a multifaceted approach to addressing ethical concerns. However, their implementation and interpretation can be complex and context-dependent, requiring careful consideration and adaptation to specific situations.
Existing Ethical Frameworks and Guidelines, AI ethics and bias
Several organizations and initiatives have published ethical guidelines for AI. These include the OECD Principles on AI, the EU’s AI Act, and various national strategies. These documents typically address key principles such as fairness, transparency, accountability, privacy, and human oversight. For instance, the OECD Principles emphasize the importance of human-centered AI, ensuring that AI systems are designed and used to benefit people and society as a whole.
The EU’s AI Act, on the other hand, focuses on risk-based regulation, categorizing AI systems based on their potential harm and implementing stricter requirements for high-risk applications. These frameworks provide a valuable starting point for organizations and developers seeking to build ethical AI systems.
Accountability and Transparency in Ethical AI
Accountability and transparency are crucial for ensuring ethical AI practices. Accountability mechanisms should be in place to identify and address instances of bias, harm, or malfunction in AI systems. This might involve clear lines of responsibility for developers, deployers, and users. Transparency involves making the workings of AI systems understandable and accessible to relevant stakeholders. This includes providing information about data sources, algorithms, and decision-making processes.
For example, a healthcare AI system diagnosing illnesses should be transparent about its reasoning process, allowing clinicians to understand and potentially override its decisions. Lack of transparency can erode trust and hinder efforts to identify and correct biases.
Comparison of Ethical Principles in AI
Fairness, accountability, transparency, and privacy are interconnected ethical principles in AI. Fairness ensures that AI systems do not discriminate against specific groups. Accountability involves establishing mechanisms to determine responsibility for AI’s actions and outcomes. Transparency requires openness about how AI systems function. Privacy protects individuals’ personal information.
While seemingly distinct, these principles often overlap. For example, a lack of transparency can hinder efforts to ensure fairness, while biased data can violate privacy. Striking a balance between these principles is a significant challenge in AI development. Consider a facial recognition system: fairness requires it to accurately identify individuals from diverse backgrounds; accountability demands that its developers are responsible for any inaccuracies; transparency necessitates understanding how the system works; and privacy mandates protecting the data used to train and operate the system.
Challenges of Implementing Ethical Guidelines in Rapidly Evolving AI
The rapid pace of AI technological advancements poses significant challenges to implementing ethical guidelines. New AI capabilities and applications emerge constantly, making it difficult to create and enforce universally applicable rules. The evolving nature of AI also necessitates ongoing evaluation and adaptation of ethical frameworks. For example, the emergence of generative AI models like large language models presents unique challenges related to intellectual property, misinformation, and the potential for malicious use, requiring new ethical considerations and guidelines.
Furthermore, the global nature of AI development necessitates international collaboration and harmonization of ethical standards to prevent regulatory fragmentation and ensure consistent ethical practices worldwide.
The Future of AI Ethics and Bias
The rapid advancement of artificial intelligence presents both immense opportunities and significant challenges. While AI promises to revolutionize various sectors, its inherent susceptibility to bias poses a considerable threat to fairness, equity, and societal well-being. Understanding and addressing the future of AI ethics and bias is crucial to harnessing AI’s potential while mitigating its potential harms. This section explores emerging trends, potential societal impacts, and preventative measures to ensure a more equitable and responsible future for AI.
Emerging Trends and Challenges in AI Ethics and Bias
The field of AI ethics and bias is constantly evolving, with new challenges emerging alongside technological advancements. One key trend is the increasing complexity of AI systems, making it harder to identify and mitigate bias. Explainable AI (XAI) is gaining traction, aiming to make AI decision-making more transparent and understandable, but it’s still in its early stages. Furthermore, the increasing use of AI in high-stakes domains, such as healthcare and criminal justice, amplifies the potential consequences of bias.
The rise of generative AI models, capable of creating realistic text, images, and videos, also presents new ethical concerns related to misinformation, deepfakes, and the potential for biased content generation. Finally, the global nature of AI development necessitates international cooperation and standardized ethical guidelines, which remain a significant challenge.
Potential Future Scenarios with Significant Societal Impacts
Several scenarios illustrate the potential societal impacts of unchecked AI bias. For instance, biased algorithms used in loan applications could disproportionately deny credit to minority groups, exacerbating existing economic inequalities. Similarly, biased facial recognition technology could lead to wrongful arrests and convictions, particularly affecting marginalized communities. In healthcare, biased AI systems could lead to misdiagnosis and unequal access to treatment.
The amplification of existing societal biases through AI could further polarize communities and deepen social divisions. Consider a scenario where an AI-powered hiring system, trained on historical data reflecting gender bias, consistently favors male candidates, perpetuating gender inequality in the workplace.
Potential Solutions and Preventative Measures
Addressing future AI bias requires a multi-pronged approach. This includes developing more robust methods for detecting and mitigating bias in datasets and algorithms. Investing in research on fairness-aware AI is crucial, focusing on developing algorithms that explicitly incorporate fairness constraints. Promoting diversity and inclusion in the AI development workforce is also essential, ensuring that diverse perspectives are considered throughout the development process.
Establishing clear ethical guidelines and regulations for AI development and deployment is necessary to hold developers accountable. Regular audits and independent evaluations of AI systems can help identify and address biases before they cause significant harm. Furthermore, promoting public awareness and education about AI bias is critical to fostering informed discussions and shaping responsible AI development.
Recommendations for Policymakers, Developers, and Researchers
To promote ethical AI development, policymakers should implement regulations that mandate bias audits and transparency in AI systems, particularly in high-stakes applications. They should also invest in research and development of fairness-aware AI technologies. Developers should prioritize the use of diverse and representative datasets, employ bias detection techniques throughout the development lifecycle, and incorporate explainability features into their AI systems.
Researchers should focus on developing robust methods for detecting and mitigating bias, exploring the social impacts of AI, and creating ethical frameworks for AI development. Collaboration between these three groups is essential to navigate the complex challenges of AI ethics and bias and ensure a more equitable and just future.
Case Study: Facial Recognition Technology
Facial recognition technology (FRT), while offering potential benefits in areas like security and law enforcement, presents significant ethical challenges, particularly concerning bias and discrimination. Its widespread adoption necessitates a careful examination of its potential for misuse and the resulting impact on fundamental rights and freedoms.The inherent biases within FRT systems stem from the datasets used to train them. These datasets often underrepresent certain demographic groups, leading to algorithms that perform less accurately on individuals from those underrepresented groups.
This inaccuracy can have serious consequences, ranging from minor inconveniences to significant injustices.
Bias and Discrimination in Facial Recognition Systems
Studies have repeatedly demonstrated that FRT systems exhibit higher error rates when identifying individuals with darker skin tones and women compared to lighter-skinned men. This disparity arises from the disproportionate representation of lighter-skinned men in the training data. For example, a 2018 study by the National Institute of Standards and Technology (NIST) found significant variations in accuracy across different demographic groups, highlighting the pervasiveness of this bias.
These inaccuracies can lead to misidentification, wrongful arrests, and other forms of discrimination. The lack of transparency in the algorithms and datasets further exacerbates the problem, making it difficult to identify and address the root causes of bias.
Potential for Misuse and Impact on Civil Liberties
The potential for misuse of FRT is considerable. Mass surveillance using FRT raises serious concerns about privacy and civil liberties. Governments and corporations could deploy this technology to monitor citizens without their knowledge or consent, potentially chilling freedom of expression and assembly. The lack of robust regulatory frameworks and oversight mechanisms increases the risk of abuse. Furthermore, the potential for biased algorithms to be used in discriminatory practices, such as targeted policing or profiling, is a significant ethical concern.
For instance, the use of FRT in predictive policing algorithms could exacerbate existing biases in law enforcement, leading to disproportionate targeting of minority communities.
Examples of Discriminatory Outcomes
Several real-world examples illustrate the discriminatory outcomes of biased FRT. Reports of wrongful arrests based on flawed facial recognition matches have surfaced, with individuals from marginalized communities disproportionately affected. In some instances, FRT has been used to misidentify individuals, leading to false accusations and unfair treatment. The lack of accountability and transparency in the deployment of these systems further compounds the problem, making it difficult to redress grievances and hold responsible parties accountable.
The use of FRT in border control and immigration enforcement has also raised concerns about its potential to exacerbate existing inequalities and discrimination against specific ethnic or racial groups.
Societal Consequences of Biased Facial Recognition Technology
Imagine a society depicted visually as a network of interconnected nodes representing individuals. Nodes representing individuals from marginalized communities are depicted as dimmer, smaller, and less connected than those representing dominant groups. These dimmer nodes represent the diminished opportunities and societal participation resulting from inaccurate and biased FRT systems. Lines connecting nodes represent interactions and relationships. The fewer connections and the weaker intensity of the lines linked to the dimmer nodes visually represent the isolation and marginalization experienced due to inaccurate identifications and discriminatory outcomes.
The overall network appears unbalanced and fragmented, highlighting the societal disruption caused by biased FRT. This imbalance negatively impacts social cohesion, trust in institutions, and the equitable distribution of resources and opportunities.
Ending Remarks: AI Ethics And Bias
In conclusion, navigating the complex landscape of AI ethics and bias requires a multi-pronged approach. Addressing algorithmic bias necessitates a concerted effort from developers, policymakers, and researchers to prioritize fairness, transparency, and accountability throughout the AI lifecycle. By fostering diverse and representative datasets, implementing robust bias detection methods, and promoting ethical guidelines, we can strive towards a future where AI serves as a force for good, benefitting all members of society equitably.
The ongoing dialogue and proactive measures are crucial in shaping a responsible and inclusive future for AI.
Common Queries
What is the difference between algorithmic bias and data bias?
Algorithmic bias refers to biases embedded within the code or algorithms themselves, while data bias reflects biases present in the data used to train the AI system. Data bias often originates from societal biases reflected in the data, while algorithmic bias can arise from flawed design choices.
How can I contribute to reducing AI bias?
You can contribute by advocating for diverse and representative datasets, supporting research on bias detection and mitigation, promoting ethical AI guidelines in your workplace or community, and engaging in informed discussions about the societal impact of AI.
What are some real-world examples of AI bias causing harm?
Examples include biased facial recognition systems misidentifying individuals from certain racial groups, loan applications unfairly denied based on biased algorithms, and hiring processes exhibiting gender or racial bias due to AI-powered recruitment tools.
Are there legal ramifications for deploying biased AI systems?
The legal landscape surrounding AI bias is still evolving, but there is increasing scrutiny and potential for legal action against companies deploying AI systems that cause demonstrable harm through bias and discrimination. Regulations are emerging in various jurisdictions to address this issue.