AI-driven Healthcare Revolutionizing Medicine
AI-driven healthcare is rapidly transforming the medical landscape, offering unprecedented opportunities to improve diagnostics, personalize treatments, and enhance patient care. From sophisticated algorithms analyzing medical images to AI-powered robotic surgery, the integration of artificial intelligence is reshaping how we approach healthcare delivery, promising more accurate diagnoses, more effective treatments, and ultimately, better health outcomes for patients worldwide. This exploration delves into the various applications of AI across the healthcare spectrum, examining its potential benefits and addressing associated challenges.
The transformative potential of AI extends beyond individual patient care, impacting hospital operations, resource management, and even healthcare access in underserved communities. This technology promises to streamline workflows, optimize resource allocation, and potentially reduce costs while simultaneously improving the quality and accessibility of care. However, ethical considerations, data privacy concerns, and the need for robust regulatory frameworks remain crucial aspects that require careful consideration as AI becomes increasingly integrated into healthcare systems.
AI Applications in Diagnostics
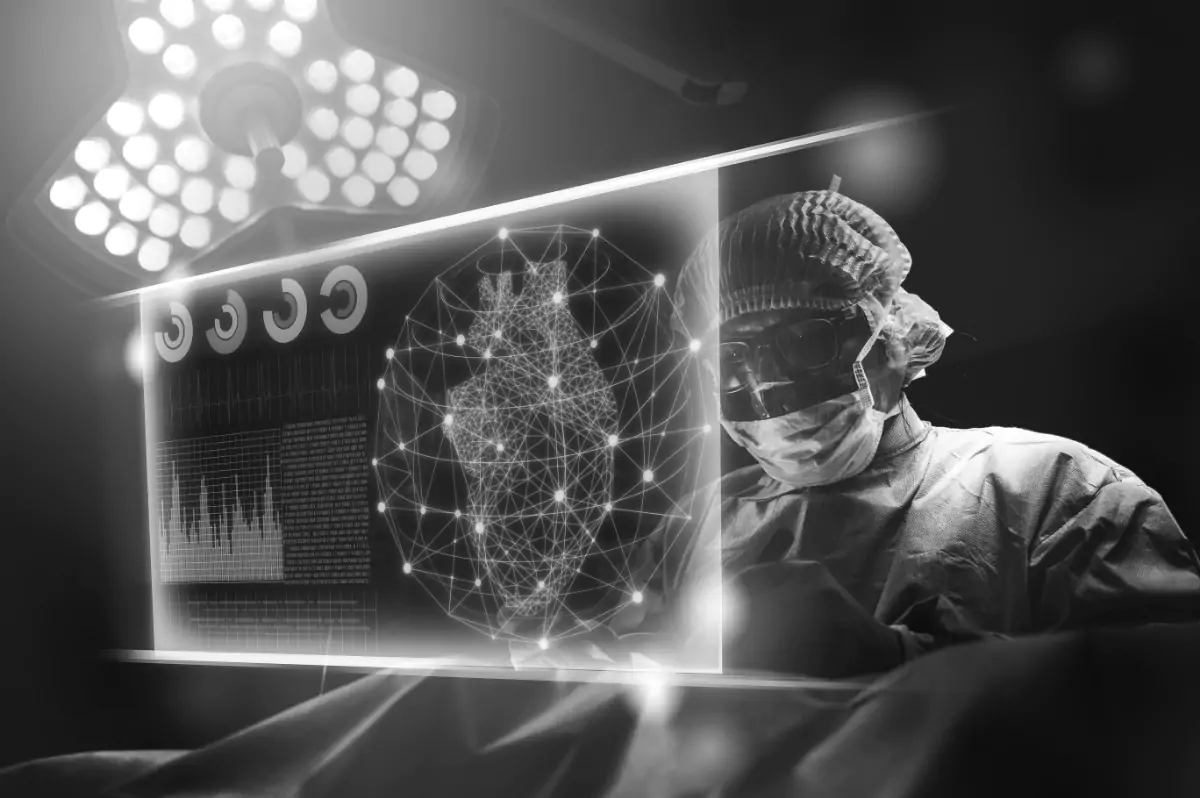
Source: kodytechnolab.com
Artificial intelligence is rapidly transforming medical diagnostics, offering the potential to improve accuracy, speed, and accessibility of healthcare. AI algorithms, trained on vast datasets of medical images and patient information, are proving invaluable in assisting clinicians in making more informed decisions. This enhanced diagnostic capability translates to earlier disease detection, improved treatment planning, and ultimately, better patient outcomes.
AI in Medical Image Analysis
AI algorithms are revolutionizing the analysis of medical images, such as X-rays, CT scans, and MRIs. These algorithms can identify subtle patterns and anomalies that might be missed by the human eye, leading to more accurate and timely diagnoses. Deep learning models, in particular, have shown remarkable success in tasks like image segmentation, object detection, and classification, enabling automated identification of tumors, fractures, and other abnormalities.
The integration of AI into diagnostic workflows is streamlining processes and improving efficiency across various medical specialties.
Accuracy, Efficiency, and Cost of AI-Based Lung Cancer Detection
The following table compares the accuracy, efficiency, and cost of AI-based diagnostic tools with traditional methods for detecting lung cancer. Note that the accuracy rates can vary depending on the specific AI algorithm, dataset used for training, and the characteristics of the patient population. These figures represent a general overview based on available research.
AI-driven healthcare is rapidly generating massive datasets, demanding efficient data processing and storage. To handle this explosive growth, scalable infrastructure is crucial, which is where solutions like Layer 2 scaling solutions become invaluable. These solutions significantly improve the speed and efficiency of AI algorithms, ultimately enhancing the accuracy and accessibility of AI-powered healthcare applications.
Method | Accuracy Rate | Time Efficiency | Cost |
---|---|---|---|
Traditional Radiologist Review | 80-85% | Variable, often lengthy | Moderate to High (depending on radiologist fees and facility costs) |
AI-Assisted Radiologist Review | 90-95% | Significantly faster | High (initial investment in AI software and ongoing maintenance, but potentially lower overall due to increased efficiency) |
AI-Only Diagnostic System (Still under development and limited clinical use) | 75-90% (accuracy varies widely based on the model and dataset) | Very fast | High (initial investment and ongoing maintenance) |
AI’s Role in Early Disease Detection and Risk Stratification, AI-driven healthcare
AI algorithms can analyze patient data, including medical images, genetic information, and lifestyle factors, to identify individuals at high risk of developing specific diseases. This allows for earlier interventions, such as lifestyle modifications or preventative screenings, potentially delaying or preventing disease onset. For example, in cardiology, AI can analyze electrocardiograms (ECGs) to detect arrhythmias and predict the risk of heart failure.
In oncology, AI can analyze genomic data to identify individuals at high risk of developing certain cancers. In radiology, AI can detect subtle changes in medical images that may indicate the early stages of a disease.
Ethical Considerations of AI-Based Diagnostic Tools
The use of AI in medical diagnostics raises several ethical considerations. A primary concern is the potential for bias in algorithms. If the training data used to develop an AI algorithm is not representative of the population it will be used on, the algorithm may produce inaccurate or discriminatory results. For instance, an algorithm trained primarily on data from one demographic group might perform poorly when used on patients from a different demographic group.
Ensuring fairness and mitigating bias in AI algorithms is crucial to avoid perpetuating existing health disparities. Transparency in algorithm development and rigorous testing are vital steps in addressing these ethical concerns. Furthermore, maintaining appropriate oversight and human review of AI-generated diagnoses is essential to ensure patient safety and responsible implementation of this technology.
AI in Personalized Medicine
The integration of artificial intelligence (AI) is revolutionizing healthcare, and personalized medicine stands to benefit significantly. AI’s ability to analyze vast datasets allows for the creation of tailored treatment plans, moving beyond a “one-size-fits-all” approach to healthcare. This shift promises more effective treatments, reduced side effects, and improved patient outcomes.
AI-Driven Tailoring of Treatment Plans
AI algorithms can analyze a patient’s unique genomic profile, lifestyle factors (diet, exercise, sleep), and medical history to predict treatment response and identify potential risks. This information enables the creation of customized treatment plans, including medication selection, dosage, and timing, specific to the individual’s needs. For example, AI could analyze a patient’s genetic makeup to determine their predisposition to certain drug side effects, allowing clinicians to choose alternative medications with a lower risk profile.
Similarly, AI could analyze lifestyle data to personalize preventative strategies, such as recommending specific dietary changes or exercise regimes based on an individual’s genetic predisposition to certain diseases.
A flowchart illustrating the process of personalized medicine using AI might look like this:
Flowchart: AI in Personalized Medicine
Start → Data Acquisition (Genomics, Lifestyle, Medical History) → Data Preprocessing and Cleaning → AI Model Training (Machine Learning Algorithms) → Prediction and Risk Assessment (Treatment Response, Side Effects) → Personalized Treatment Plan Generation (Medication, Dosage, Lifestyle Recommendations) → Treatment Monitoring and Adjustment (Feedback Loop) → Outcome Evaluation → End
Comparison of AI-Driven and Traditional Drug Discovery and Development
The development of new drugs traditionally involves a lengthy and expensive process. AI is accelerating this process by significantly improving efficiency and reducing costs.
Here’s a comparison:
- Traditional Methods: Relies heavily on trial-and-error experimentation, often involving lengthy pre-clinical and clinical trials. This process can take many years and cost billions of dollars.
- AI-Driven Methods: Leverages machine learning algorithms to analyze vast datasets of molecular structures, biological pathways, and clinical trial data to identify promising drug candidates. This approach can significantly reduce the time and cost associated with drug discovery and development.
- Traditional Methods: Limited ability to predict individual patient responses to drugs.
- AI-Driven Methods: Can predict individual patient responses to drugs based on genomic and other patient-specific data, leading to more effective and safer treatments.
- Traditional Methods: Target identification and validation often rely on laborious experimental techniques.
- AI-Driven Methods: AI can accelerate target identification and validation by analyzing large datasets to identify potential drug targets and predict their effectiveness.
Challenges and Opportunities in Implementing AI-Powered Personalized Medicine
While AI-powered personalized medicine offers tremendous potential, several challenges need to be addressed for successful implementation.
Challenges:
- Data Privacy and Security: Protecting sensitive patient data is paramount. Robust security measures and adherence to data privacy regulations are crucial.
- Data Bias and Fairness: AI algorithms can inherit biases present in the data they are trained on, leading to disparities in treatment outcomes. Careful data curation and algorithm design are necessary to mitigate this risk.
- Lack of Interoperability: The seamless exchange of patient data between different healthcare systems is essential for personalized medicine. Standardization and interoperability of healthcare data are crucial.
- Regulatory Hurdles: The regulatory landscape for AI-driven medical devices and therapies is still evolving. Clear guidelines and regulatory pathways are needed to facilitate the adoption of AI-powered personalized medicine.
Opportunities:
AI-driven healthcare is rapidly evolving, promising personalized medicine and improved efficiency. The secure and transparent management of patient data is crucial, and this raises questions about the best blockchain consensus mechanisms. Understanding the differences between Proof of stake vs proof of work is vital in this context, as it impacts the energy efficiency and security of such systems.
Ultimately, the choice of consensus mechanism will influence the future development and adoption of AI in healthcare.
- Improved Patient Outcomes: Personalized medicine can lead to more effective treatments, fewer side effects, and improved overall patient outcomes.
- Reduced Healthcare Costs: By preventing diseases and optimizing treatments, AI-powered personalized medicine can reduce healthcare costs in the long run.
- Enhanced Drug Discovery and Development: AI can accelerate the process of drug discovery and development, leading to the faster availability of new and effective therapies.
- Empowered Patients: Personalized medicine can empower patients by providing them with more control over their healthcare decisions.
AI-powered Robotic Surgery: AI-driven Healthcare
AI-powered robotic surgery represents a significant advancement in the field of minimally invasive procedures. By integrating artificial intelligence into surgical robots, surgeons can achieve enhanced precision, improved visualization, and greater control, ultimately leading to better patient outcomes. This technology is rapidly evolving, promising to revolutionize surgical practices across various specialties.
Capabilities of AI-Assisted Surgical Robots
AI-assisted surgical robots significantly enhance precision and enable minimally invasive procedures. These robots are equipped with advanced sensors, cameras, and AI algorithms that provide surgeons with real-time feedback and guidance during the operation. This results in smaller incisions, reduced trauma to surrounding tissues, less blood loss, shorter hospital stays, and faster recovery times for patients. The AI algorithms can also compensate for surgeon tremor, enhancing the stability and accuracy of surgical movements.
AI-driven healthcare offers incredible potential to revolutionize patient care, from diagnosis to treatment. However, realizing this potential requires careful consideration of the ethical implications, particularly concerning issues of algorithmic bias. Understanding these concerns is crucial; for more information, please refer to this helpful resource on AI ethics and bias. Ultimately, addressing these biases is vital for ensuring equitable and effective AI applications within the healthcare sector.
AI Algorithms in Surgical Planning and Execution
AI algorithms play a crucial role in both the planning and execution phases of robotic surgery. During the planning phase, AI can analyze medical images (CT scans, MRI scans) to create 3D models of the patient’s anatomy. This allows surgeons to virtually plan the procedure, simulating the surgical steps and identifying potential challenges before the actual operation. During the execution phase, AI algorithms provide real-time assistance, such as:
- Predictive analytics: AI can predict potential complications during the surgery and alert the surgeon.
- Haptic feedback: AI can enhance the sense of touch for the surgeon, providing more precise control of the surgical instruments.
- Image enhancement: AI can improve the quality of the surgical images, providing a clearer view of the surgical field.
- Automated suturing: Some robotic systems are being developed with AI-powered automated suturing capabilities.
Examples of AI Features in Robotic Surgery Systems
Several robotic surgery systems already incorporate AI features. For example, some systems use AI to automatically track the movement of organs during surgery, compensating for any shifts in position. Others use AI to identify and highlight critical anatomical structures, reducing the risk of accidental damage. The integration of machine learning allows for continuous improvement of the system’s performance over time, learning from previous surgeries to optimize future procedures.
Comparative Analysis of Robotic Surgery Systems
The following table provides a comparison of some prominent robotic surgery systems and their features:
Robot Name | Surgical Application | Advantages | Limitations |
---|---|---|---|
da Vinci Surgical System | General surgery, urology, gynecology, cardiac surgery | High precision, minimally invasive, 3D visualization | High cost, requires specialized training, limited dexterity in some applications |
Intuitive Surgical’s Ion System | Lung cancer surgery | Smaller incisions, reduced trauma, improved visualization | Relatively new technology, limited availability |
Verb Surgical System (under development) | Various surgical applications | Integration of AI and machine learning, improved precision and efficiency | Still under development, long-term effectiveness yet to be fully evaluated |
Risks and Benefits of Increased AI Use in Robotic Surgery
The increased use of AI in robotic surgery presents both benefits and risks. Benefits include improved precision, reduced invasiveness, and potentially faster recovery times. However, risks include the potential for AI errors, dependence on technology, and ethical concerns related to algorithmic bias and data privacy. Robust testing, validation, and regulatory oversight are crucial to mitigate these risks and ensure the safe and effective implementation of AI in robotic surgery.
AI and Healthcare Management
AI is rapidly transforming healthcare management, offering innovative solutions to optimize hospital operations, predict patient outcomes, and improve access to care. By analyzing vast amounts of data, AI algorithms can identify patterns and trends invisible to the human eye, leading to significant improvements in efficiency, cost-effectiveness, and patient safety. This section explores several key applications of AI in streamlining healthcare administration and enhancing patient care.AI Applications in Optimizing Hospital OperationsEfficient hospital operations are crucial for delivering high-quality patient care.
AI-driven healthcare is revolutionizing patient care through personalized treatments and efficient data management. However, the security of this sensitive patient data is paramount, which is why robust security measures are crucial. A key aspect of this is leveraging technologies like Blockchain security to ensure data integrity and privacy, ultimately enhancing the trustworthiness and effectiveness of AI in healthcare.
AI significantly contributes to optimizing various aspects of hospital management, leading to better resource utilization and improved patient flow.
- Patient Flow Optimization: AI algorithms can predict patient arrival times, estimate length of stay, and optimize bed allocation, minimizing wait times and improving patient throughput. For example, an AI system might analyze historical data on patient admissions, diagnostic tests, and treatment durations to predict the number of beds needed on a given day, preventing overcrowding and delays.
- Resource Allocation: AI can analyze real-time data on resource utilization (e.g., equipment, staff, supplies) to optimize allocation, reducing waste and ensuring resources are available when and where needed. This could involve dynamically assigning nurses based on patient acuity or predicting the demand for specific medical supplies.
- Staff Scheduling: AI-powered scheduling tools can create optimized staff schedules, considering factors such as skill sets, experience, and availability, minimizing overtime costs and ensuring adequate staffing levels across all departments. These systems can also adapt to unexpected absences or changes in patient volume.
Benefits of AI in Hospital ManagementThe integration of AI in hospital management offers numerous benefits:
- Improved Efficiency: Automation of tasks and optimized resource allocation lead to increased efficiency and reduced operational costs.
- Enhanced Patient Care: Reduced wait times, improved bed allocation, and better resource management contribute to a better patient experience and improved outcomes.
- Reduced Costs: Optimized resource utilization, minimized waste, and improved staff scheduling can significantly reduce hospital operating costs.
- Better Decision-Making: Data-driven insights from AI provide healthcare administrators with valuable information for strategic planning and decision-making.
AI in Predicting Hospital Readmissions and Preventing Adverse EventsPredictive analytics powered by AI play a crucial role in identifying patients at high risk of readmission or adverse events. By analyzing patient data such as medical history, vital signs, and lab results, AI algorithms can flag potential problems and allow for proactive interventions.
AI-Driven Prevention of Adverse Events
Consider a scenario where an elderly patient with a history of heart failure is admitted for pneumonia. An AI system, analyzing the patient’s electronic health record (EHR) and real-time vital signs, detects a subtle but significant increase in heart rate and blood pressure. This deviation from the patient’s baseline, coupled with the existing heart condition, triggers an alert for the medical team.
The alert prompts a thorough assessment, revealing early signs of cardiac decompensation. Prompt intervention, guided by the AI’s prediction, prevents a potentially fatal cardiac event.AI and Improving Healthcare Access and Affordability in Underserved CommunitiesAI offers promising solutions to address healthcare disparities in underserved communities. Telemedicine platforms powered by AI can provide remote access to specialists and diagnostic services, overcoming geographical barriers.
AI-driven tools can also assist in managing chronic conditions remotely, reducing the need for frequent hospital visits and lowering healthcare costs. Furthermore, AI can help optimize resource allocation in underserved areas, ensuring that limited resources are used effectively to maximize impact. For instance, an AI system could analyze demographic data and health needs to identify areas with the greatest need for mobile health clinics or telehealth services.
AI and Patient Monitoring
AI is revolutionizing patient monitoring, moving beyond traditional methods to provide continuous, real-time data and enabling proactive interventions. This shift towards continuous monitoring is facilitated by the integration of wearable sensors and sophisticated AI algorithms, leading to improved patient outcomes and more efficient healthcare delivery.
Wearable sensors, coupled with AI algorithms, allow for continuous patient monitoring and remote patient care. These devices collect physiological data, such as heart rate, blood pressure, oxygen saturation, and activity levels. AI algorithms then analyze this data, identifying patterns and anomalies that might indicate a developing health issue. This allows for early intervention, potentially preventing hospitalizations or adverse events. This proactive approach contrasts sharply with traditional methods, which typically rely on periodic check-ups and reactive responses to symptoms.
A Hypothetical Wearable Device and its AI Capabilities
Imagine a smart wristband, “HealthWatch,” designed for continuous health monitoring. HealthWatch incorporates a range of sensors: an optical heart rate sensor, a PPG sensor for blood oxygen saturation, an accelerometer to track movement and activity levels, and a galvanic skin response (GSR) sensor to measure stress levels. The device transmits this data wirelessly to a secure cloud platform.
AI algorithms on this platform analyze the data in real-time, looking for deviations from established baselines. For example, if the patient’s heart rate consistently exceeds a pre-defined threshold, or if their sleep patterns show significant disruption coupled with elevated stress levels, the system triggers an alert to the patient’s physician or designated caregiver. Furthermore, the AI can predict potential health risks, such as the likelihood of a heart attack or stroke, based on the collected data and the patient’s medical history.
This predictive capability allows for timely interventions, potentially saving lives. The device also incorporates features such as medication reminders and personalized health recommendations based on the AI’s analysis.
Comparison of AI-Powered Remote Patient Monitoring with Traditional Methods
The effectiveness of AI-powered remote patient monitoring significantly surpasses traditional methods in several key aspects:
Traditional methods often rely on infrequent clinic visits or scheduled check-ups, potentially missing crucial early warning signs of health deterioration. In contrast, AI-powered remote monitoring provides continuous data, enabling early detection of problems and timely interventions. This comparison highlights the transformative potential of AI in proactive healthcare.
- Data Frequency: AI-powered systems provide continuous data, while traditional methods rely on periodic measurements.
- Early Detection: AI can detect subtle changes indicating potential problems before they become critical, whereas traditional methods often rely on symptomatic presentation.
- Proactive Intervention: AI enables proactive interventions, preventing potential health crises, unlike traditional reactive approaches.
- Improved Patient Engagement: Continuous monitoring encourages patients to actively participate in their health management.
- Reduced Healthcare Costs: By preventing hospitalizations and complications, AI-powered monitoring can potentially reduce overall healthcare costs.
Privacy and Security Concerns in AI-Powered Patient Monitoring
The collection and analysis of sensitive patient data in AI-powered monitoring systems raise significant privacy and security concerns. Data breaches could lead to unauthorized access to personal health information, potentially causing significant harm to patients. Robust security measures, including data encryption, access control, and anonymization techniques, are crucial to mitigate these risks. Furthermore, ethical considerations surrounding data ownership, consent, and the potential for algorithmic bias must be carefully addressed.
Compliance with relevant data privacy regulations, such as HIPAA in the United States and GDPR in Europe, is paramount. Transparency regarding data usage and algorithmic decision-making is essential to build trust and ensure patient autonomy. The development and implementation of AI-powered patient monitoring systems must prioritize patient privacy and data security to ensure responsible and ethical use of this powerful technology.
Wrap-Up
In conclusion, AI-driven healthcare presents a paradigm shift in medical practice, promising a future where diagnoses are faster and more accurate, treatments are personalized to individual needs, and healthcare delivery is more efficient and accessible. While challenges remain, the potential benefits of AI in improving patient outcomes and transforming healthcare systems are undeniable. Continued research, development, and responsible implementation are crucial to harnessing the full potential of this transformative technology while mitigating potential risks and ensuring equitable access for all.
Essential Questionnaire
What are the biggest challenges in implementing AI in healthcare?
Major challenges include data privacy and security concerns, the need for robust regulatory frameworks, algorithmic bias, the high cost of implementation, and the integration of AI systems into existing healthcare infrastructure.
How does AI improve patient safety?
AI can improve patient safety through early disease detection, predictive modeling of adverse events, and improved precision in surgical procedures, reducing human error and improving overall outcomes.
Will AI replace doctors?
No, AI is intended to augment, not replace, healthcare professionals. AI tools assist doctors and other healthcare providers, enabling them to make more informed decisions and improve efficiency, ultimately leading to better patient care.
What is the role of human oversight in AI-driven healthcare?
Human oversight is crucial. Healthcare professionals are needed to interpret AI’s findings, make clinical judgments, and ensure ethical and responsible use of AI tools. Human expertise remains essential for effective healthcare delivery.
How is AI addressing healthcare disparities?
AI has the potential to improve healthcare access in underserved communities by providing remote diagnosis and monitoring capabilities, assisting in resource allocation, and developing more affordable and accessible treatment options.