Quantum Computing Applications Exploring the Future
Quantum computing applications are poised to revolutionize numerous fields. This powerful technology leverages the principles of quantum mechanics to perform calculations beyond the capabilities of even the most advanced classical computers. Imagine simulating complex molecules with unprecedented accuracy, optimizing global supply chains in real-time, or developing unbreakable encryption methods. This exploration delves into the exciting potential and current realities of this transformative technology.
From accelerating drug discovery and materials science to revolutionizing finance and cryptography, quantum computing promises to solve problems currently intractable for classical computers. Its unique ability to handle vast amounts of data and perform complex calculations opens doors to breakthroughs across various sectors, impacting everything from our daily lives to the global economy. However, this nascent technology also presents significant challenges, including the need for further technological advancements and careful consideration of ethical implications.
Introduction to Quantum Computing Applications
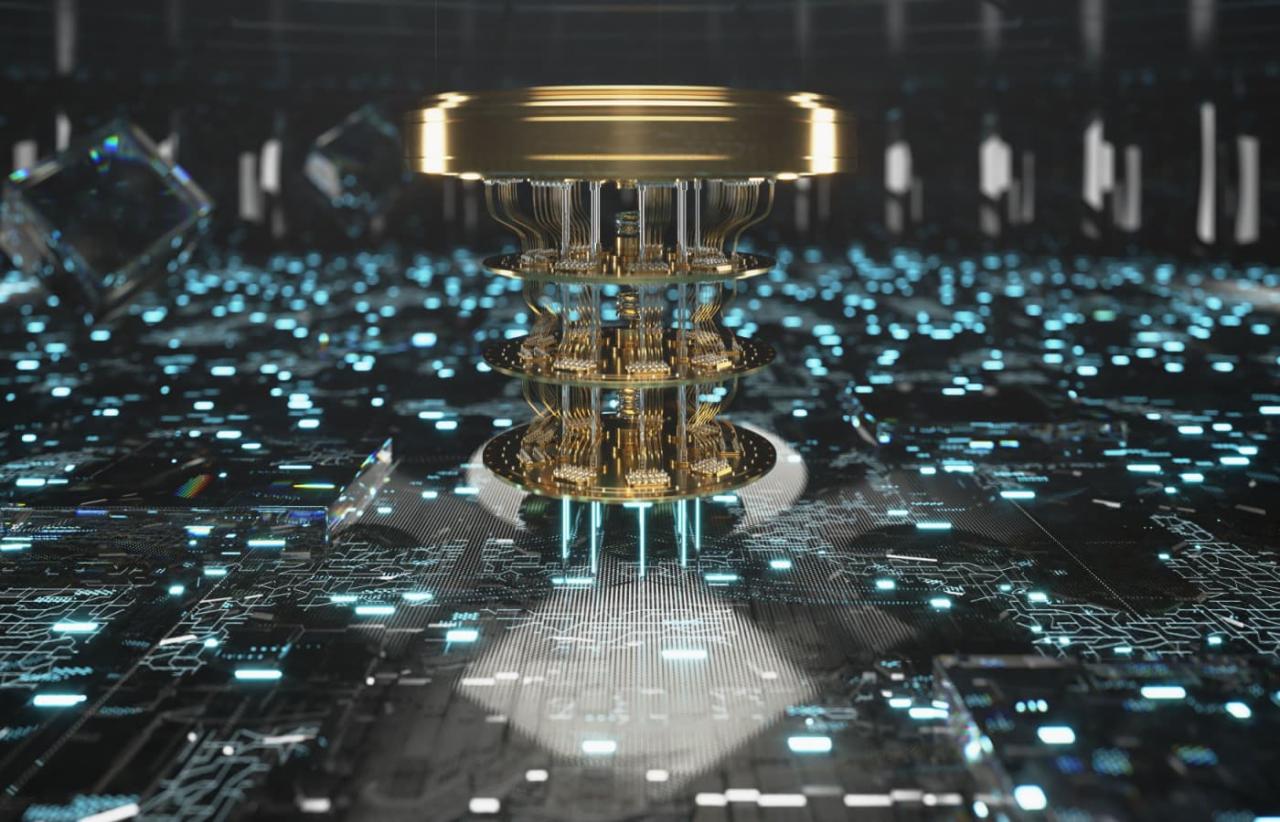
Source: neilsahota.com
Quantum computing represents a paradigm shift in computation, leveraging the bizarre principles of quantum mechanics to solve problems intractable for even the most powerful classical computers. Unlike classical computers that store information as bits representing 0 or 1, quantum computers utilize qubits. Qubits, through superposition and entanglement, can exist in a combination of 0 and 1 simultaneously, exponentially increasing their computational power for specific tasks.
This fundamental difference unlocks the potential for revolutionary advancements across numerous fields.The advantages of quantum computing stem from its ability to perform computations in fundamentally different ways. Classical computers struggle with certain complex problems, such as simulating molecular interactions or breaking current encryption methods, due to the exponential growth in computational resources required. Quantum algorithms, however, can tackle these problems efficiently, offering solutions that are currently impossible to achieve with classical approaches.
This potential for speedup is particularly significant in areas like drug discovery, materials science, and cryptography.
Quantum Computing Development and Current State
Quantum computing’s history traces back to the theoretical foundations laid in the early 1980s. Richard Feynman and Yuri Manin independently proposed that quantum systems could simulate other quantum systems more efficiently than classical computers. The first experimental implementations of rudimentary quantum computers emerged in the late 1990s, using trapped ions or superconducting circuits. Progress has been rapid in recent years, with significant investment from both governments and private companies leading to the development of more powerful and stable quantum computers.
Currently, we are in the “noisy intermediate-scale quantum” (NISQ) era, where quantum computers are still relatively small and prone to errors, but capable of demonstrating quantum advantage in specific tasks. Companies like IBM, Google, and Rigetti are actively developing and providing access to their quantum computing platforms, paving the way for wider adoption and further research. For example, Google claimed “quantum supremacy” in 2019, demonstrating that their quantum computer performed a specific calculation faster than any known classical algorithm.
While this claim remains debated, it highlights the rapid advancements and potential of the field.
Quantum Computing in Drug Discovery and Materials Science
Quantum computing holds immense potential to revolutionize drug discovery and materials science, fields traditionally hampered by the computational complexity of simulating molecular interactions and material properties. Classical computers struggle to handle the vast number of variables involved in accurately modeling these systems, leading to lengthy development times and high costs. Quantum computers, leveraging the principles of superposition and entanglement, offer a path towards significantly faster and more accurate simulations.Quantum simulations can accelerate drug discovery by efficiently modeling molecular interactions.
Quantum computing applications are poised to revolutionize various fields, from medicine to materials science. The immense data processing power needed for these applications will heavily rely on advancements in communication infrastructure, which is where the exciting work on 6G research and development comes in. Ultimately, the speed and capacity of 6G will be crucial for realizing the full potential of quantum computing’s transformative capabilities.
This allows researchers to explore a much wider range of potential drug candidates and predict their efficacy and potential side effects with greater accuracy. By simulating the binding of drug molecules to target proteins, for instance, quantum computers can help identify promising drug candidates much earlier in the development process, saving time and resources. Furthermore, they can assist in optimizing drug design for improved efficacy and reduced toxicity.
Quantum Simulation of Molecular Interactions
Quantum algorithms, such as the Variational Quantum Eigensolver (VQE) and Quantum Approximate Optimization Algorithm (QAOA), are particularly well-suited for simulating the complex quantum mechanical behavior of molecules. These algorithms leverage the inherent quantum properties of qubits to represent the quantum states of molecules and efficiently calculate their energies and other properties. This allows for the exploration of potential drug-target interactions with unprecedented accuracy, potentially leading to the discovery of novel drugs and therapies.
For example, VQE can efficiently compute the ground state energy of a molecule, which is crucial for understanding its stability and reactivity. This ability to accurately predict molecular properties is a significant advantage over classical methods.
Designing New Materials with Enhanced Properties
The design of new materials with tailored properties, such as increased strength, conductivity, or reactivity, is another area where quantum computing shows significant promise. Quantum simulations can model the behavior of materials at the atomic level, allowing researchers to predict their properties and optimize their design for specific applications. For example, simulating the behavior of different crystal structures can lead to the discovery of new materials with superior properties for use in electronics, energy storage, or construction.
Quantum algorithms can efficiently explore a vast parameter space, identifying optimal material compositions and structures that would be computationally prohibitive to explore using classical methods.
Comparing the Efficiency of Quantum and Classical Methods for Simulating Molecular Dynamics
Classical methods, such as molecular dynamics simulations, often rely on approximations and simplifications to handle the complexity of molecular interactions. This can lead to inaccuracies and limitations in predicting the behavior of molecules. Quantum simulations, on the other hand, offer the potential for more accurate and detailed representations of molecular dynamics. While current quantum computers are still limited in their scale and capabilities, they are already showing promising results in outperforming classical methods for certain types of molecular simulations, particularly those involving strongly correlated electrons.
As quantum computers continue to improve, their advantage over classical methods is expected to become even more pronounced. The exponential speedup offered by some quantum algorithms, such as Shor’s algorithm for factoring large numbers, although not directly applicable to molecular dynamics, hints at the potential for transformative improvements in computational efficiency.
Computational Resource Comparison for Molecular Simulations
Molecule | Property | Classical Method | Quantum Method |
---|---|---|---|
Hydrogen Molecule (H2) | Ground State Energy | High-performance computing cluster, days to weeks of computation | Noisy Intermediate-Scale Quantum (NISQ) device, hours to days (depending on device capabilities) |
Lithium Hydride (LiH) | Bond Length | High-performance computing cluster, significant computational time | NISQ device, potentially faster than classical methods for certain parameter regimes |
Quantum Computing in Finance
The financial industry, known for its complex calculations and vast datasets, is ripe for disruption by quantum computing. Quantum algorithms offer the potential to significantly improve the speed and accuracy of various financial processes, leading to better decision-making and increased profitability. This section explores the transformative potential of quantum computing in several key areas of finance.
Optimizing Investment Portfolios
Quantum computing’s ability to handle massive datasets and complex calculations makes it ideally suited for portfolio optimization. Classical algorithms struggle with the computational complexity of optimizing portfolios with many assets, often resorting to simplifying assumptions that can lead to suboptimal results. Quantum algorithms, such as Quantum Approximate Optimization Algorithm (QAOA) and Variational Quantum Eigensolver (VQE), can explore a much larger solution space more efficiently, potentially leading to portfolios that achieve higher returns for a given level of risk.
For example, a quantum algorithm could simultaneously consider thousands of assets, their correlations, and various risk factors to construct a portfolio that maximizes expected returns while minimizing risk, outperforming traditional methods that often rely on simplifying assumptions or heuristics.
Quantum Computing in Risk Management and Fraud Detection
Risk management and fraud detection are crucial aspects of the financial industry. Quantum algorithms can enhance these processes by identifying subtle patterns and anomalies in vast transaction datasets that would be missed by classical methods. For instance, quantum machine learning algorithms can be trained on historical fraud data to identify complex relationships and predict future fraudulent activities with greater accuracy.
Similarly, in risk management, quantum algorithms can be used to model and assess complex financial risks, such as systemic risk in interconnected financial markets, providing more accurate and timely risk assessments. This improved accuracy could lead to more effective risk mitigation strategies and prevent significant financial losses.
Comparison of Quantum and Classical Algorithms for Financial Modeling, Quantum computing applications
While classical algorithms are currently the standard in financial modeling, quantum algorithms offer significant advantages in certain areas. For instance, Monte Carlo simulations, commonly used to price complex financial derivatives, can be significantly accelerated using quantum computers. Quantum algorithms can handle a much larger number of simulations in a shorter time, leading to more accurate pricing and hedging strategies.
However, it’s important to note that quantum computers are not yet mature enough to replace classical computers entirely. Currently, quantum algorithms are more effective for specific types of problems, and hybrid approaches, combining classical and quantum computation, are often the most practical solution. The relative performance depends heavily on the specific problem and the size of the input data.
For example, pricing a complex option using a Monte Carlo simulation on a quantum computer might show a speedup compared to a classical computer, while other tasks, such as basic accounting, might be more efficiently handled classically.
Hypothetical Scenario: Improving Financial Forecasting
Imagine a large investment bank using quantum computing to improve its macroeconomic forecasting. The bank’s classical models struggle to accurately predict economic downturns due to the complexity of interconnected global economic factors. By leveraging a quantum algorithm capable of analyzing massive datasets including global trade data, interest rates, inflation rates, and consumer sentiment indicators, the bank can create a significantly more accurate predictive model.
This model can identify subtle correlations and patterns that are missed by classical methods, leading to more accurate predictions of economic cycles. Consequently, the bank can make more informed investment decisions, better manage its risk exposure, and potentially avoid significant financial losses during economic downturns. For example, the bank could predict a recession six months earlier than traditional methods, allowing it to adjust its portfolio accordingly and minimize losses.
Quantum Computing in Cryptography
The advent of powerful quantum computers poses a significant threat to the security of many widely used encryption methods. Current cryptographic systems rely on mathematical problems that are computationally infeasible to solve with classical computers, but quantum algorithms could potentially crack these problems relatively quickly, undermining the confidentiality and integrity of sensitive data. This necessitates a shift towards post-quantum cryptography, preparing for a future where quantum computers are a reality.Quantum computers leverage the principles of quantum mechanics, specifically superposition and entanglement, to perform computations in a fundamentally different way than classical computers.
Shor’s algorithm, for example, is a quantum algorithm that can efficiently factor large numbers, a problem that underpins the security of widely used public-key cryptosystems like RSA. Similarly, Grover’s algorithm offers a quadratic speedup for searching unsorted databases, potentially weakening symmetric encryption methods. The implications for cybersecurity are substantial, ranging from compromised financial transactions and sensitive government data to widespread disruption of online services.
Quantum Computer’s Threat to Current Encryption
Quantum computers, with their unique capabilities, pose a direct threat to several widely used encryption methods. The RSA algorithm, for example, relies on the difficulty of factoring large numbers. A sufficiently powerful quantum computer running Shor’s algorithm could factor these numbers quickly, rendering RSA encryption vulnerable. Similarly, the Diffie-Hellman key exchange, a cornerstone of secure communication protocols, is also susceptible to quantum attacks.
The impact would be far-reaching, affecting everything from online banking and e-commerce to national security communications. The potential for widespread data breaches and disruption of critical infrastructure is a major concern driving the development of post-quantum cryptography.
Post-Quantum Cryptography: Building Resilience
Post-quantum cryptography (PQC) refers to cryptographic algorithms that are believed to be secure against both classical and quantum computers. These algorithms are based on mathematical problems that are considered hard even for quantum computers. Several promising approaches are being explored, including lattice-based cryptography, code-based cryptography, multivariate cryptography, hash-based cryptography, and isogeny-based cryptography. These approaches leverage different mathematical structures and complexities to provide security against quantum attacks.
Quantum computing applications are rapidly expanding, with potential uses in various fields like medicine and materials science. A key area driving this growth is the development of Quantum AI , which leverages quantum computing power to create more advanced artificial intelligence. This synergy promises to significantly accelerate the development and deployment of innovative quantum computing applications across numerous sectors.
The development of PQC is a complex and ongoing process, requiring rigorous mathematical analysis and extensive testing to ensure its robustness and practical implementation. Standardization efforts are underway to select and deploy the most secure and efficient PQC algorithms.
Comparison of Encryption Algorithms
The following table compares the security levels of different encryption algorithms against classical and quantum attacks. It’s important to note that the security levels are relative and depend on the specific implementation and key sizes.
Algorithm | Classical Security | Quantum Security |
---|---|---|
RSA | High (with sufficiently large key sizes) | Low (vulnerable to Shor’s algorithm) |
ECC (Elliptic Curve Cryptography) | High (with sufficiently large key sizes) | Low (vulnerable to Shor’s algorithm) |
AES (Advanced Encryption Standard) | High (with appropriate key sizes and modes of operation) | Moderate (vulnerable to Grover’s algorithm, but requires significantly more resources than breaking RSA) |
Lattice-based cryptography | High | High (believed to be resistant to known quantum algorithms) |
Current Research Efforts in Post-Quantum Cryptography
Significant research efforts are underway to develop and standardize post-quantum cryptographic algorithms. These efforts involve mathematicians, cryptographers, and computer scientists worldwide.The National Institute of Standards and Technology (NIST) has led a significant standardization process, evaluating and selecting candidate algorithms for standardization. This process involved rigorous analysis and public review to identify algorithms that are both secure and efficient.
Several promising candidates emerged from this process, spanning various cryptographic approaches. For example, CRYSTALS-Kyber (lattice-based) and CRYSTALS-Dilithium (lattice-based) were selected for standardization as key establishment and digital signature algorithms, respectively. These algorithms offer strong security against quantum attacks while being relatively efficient for practical implementation. Other research focuses on improving the efficiency and performance of PQC algorithms, exploring new mathematical foundations, and developing robust implementation techniques to ensure widespread adoption.
The ongoing research is crucial for securing our digital infrastructure in the age of quantum computing.
Quantum computing applications are poised to revolutionize various fields, impacting everything from medicine to materials science. The massive data processing demands of these advancements will require incredibly fast and reliable networks, which is where the ongoing 5G network expansion becomes crucial. This infrastructure will be essential for supporting the data transfer needs of future quantum computers and their complex algorithms.
Quantum Computing in Optimization Problems
Optimization problems, ubiquitous across various fields, involve finding the best solution from a vast number of possibilities. Classical computers struggle with these problems as the number of possibilities grows exponentially, a phenomenon known as the “combinatorial explosion.” Quantum computing offers a potential pathway to overcome this limitation, leveraging the principles of superposition and entanglement to explore multiple solutions simultaneously.
This allows for significantly faster solutions to certain classes of optimization problems compared to classical approaches.Quantum computing’s ability to tackle optimization problems stems from its unique capabilities. Specifically, quantum algorithms can efficiently search through a large solution space, identifying near-optimal or optimal solutions much faster than classical algorithms. This advantage is particularly pronounced for problems with complex, non-linear relationships between variables.
Types of Optimization Problems Suitable for Quantum Computing
Many types of optimization problems can benefit from quantum computing’s power. These include combinatorial optimization problems (like the traveling salesman problem), quadratic unconstrained binary optimization (QUBO) problems, and integer programming problems. These problems frequently appear in logistics, finance, and materials science. For instance, finding the most efficient route for a delivery fleet (traveling salesman problem), optimizing a portfolio for maximum return (portfolio optimization), or designing a new material with specific properties (materials design) all fall under the umbrella of optimization problems that could be accelerated using quantum algorithms.
Quantum Algorithms for Optimization Problems
Several quantum algorithms are specifically designed to address optimization problems. Quantum Approximate Optimization Algorithm (QAOA) and Variational Quantum Eigensolver (VQE) are prominent examples. These algorithms leverage quantum hardware to iteratively improve solutions, often finding near-optimal solutions in a reasonable timeframe. For example, in logistics, QAOA could be used to optimize delivery routes by considering factors like distance, traffic, and delivery time windows.
In supply chain management, VQE might be employed to optimize inventory levels, minimizing storage costs while ensuring sufficient stock to meet demand. Similarly, in traffic flow optimization, quantum algorithms could analyze traffic patterns and suggest optimal traffic light timings to minimize congestion.
Quantum Annealing for Optimization
Quantum annealing is a specialized type of quantum computing that’s particularly well-suited for solving optimization problems. It uses a quantum process to find the lowest energy state of a system, which corresponds to the optimal solution of the problem. This method is particularly effective for QUBO problems, which can be formulated to represent many optimization challenges. For instance, consider the problem of assigning tasks to workers in a factory to minimize overall completion time.
This can be formulated as a QUBO problem, where each bit represents whether a specific task is assigned to a specific worker. The energy function reflects the total completion time, and quantum annealing finds the configuration (assignment of tasks to workers) that minimizes this energy, thus minimizing the overall completion time.
Solving Optimization Problems Using a Quantum Algorithm: A Flowchart
The process of solving an optimization problem using a quantum algorithm can be visualized as follows:[Imagine a flowchart here. The flowchart would have these steps:
1. Problem Formulation
Define the optimization problem mathematically, often expressing it as a QUBO or another suitable form.
2. Algorithm Selection
Choose an appropriate quantum algorithm (e.g., QAOA, VQE, or quantum annealing) based on the problem structure and available hardware.
3. Encoding
Encode the problem into a format suitable for the chosen quantum algorithm. This often involves mapping the problem variables to qubits.
4. Quantum Computation
Execute the chosen algorithm on a quantum computer.
Quantum computing applications are rapidly expanding, impacting various fields. A particularly exciting area is the use of quantum algorithms in drug discovery, where the potential for breakthroughs is immense. Learn more about this at Quantum AI applications in drug discovery and development , which highlights how quantum AI accelerates the process. Ultimately, these advancements will further refine the capabilities of quantum computing applications across the board.
5. Measurement and Decoding
Measure the qubits and decode the results to obtain a solution to the original optimization problem.
6. Post-processing (Optional)
Refine the solution using classical algorithms if necessary.The flowchart would show these steps connected by arrows, indicating the flow of the process.]
Challenges and Future Directions of Quantum Computing Applications
Quantum computing holds immense promise, but its widespread adoption faces significant hurdles. Realizing its full potential requires overcoming technological limitations, addressing ethical concerns, and anticipating the societal impacts of this transformative technology. The next decade will likely witness significant advancements, shaping the future of various sectors.
Technological Hurdles to Widespread Adoption
Several technological challenges currently hinder the widespread adoption of quantum computing. These obstacles are intertwined and require concerted effort across multiple disciplines for their resolution. Key among these are qubit coherence, scalability, and error correction. Maintaining the delicate quantum states of qubits (the fundamental units of quantum information) for extended periods is crucial for complex computations. Current quantum computers struggle with decoherence, where qubits lose their quantum properties due to interactions with their environment.
Scaling up the number of qubits while maintaining their coherence and control presents another major challenge. Furthermore, robust error correction mechanisms are essential to mitigate the effects of noise and errors inherent in quantum systems. The development of fault-tolerant quantum computers is a significant research focus. For instance, the challenge of building a large-scale, fault-tolerant quantum computer is comparable to the challenge of building the first integrated circuit, requiring breakthroughs in materials science, engineering, and control systems.
Ethical Considerations in Quantum Computing Development and Application
The immense power of quantum computing necessitates careful consideration of its ethical implications. The potential for breaking existing encryption algorithms poses a significant threat to cybersecurity and data privacy. Quantum-resistant cryptography is actively being developed, but its deployment and widespread adoption require careful planning and coordination. Moreover, the concentration of quantum computing resources in the hands of a few powerful entities raises concerns about equitable access and potential misuse.
Algorithmic bias, a problem already present in classical machine learning, could be amplified in quantum machine learning algorithms, leading to unfair or discriminatory outcomes. Therefore, ethical guidelines and regulations are crucial to ensure responsible development and deployment of quantum technologies, preventing their misuse and promoting fairness and inclusivity.
Societal Impacts of Quantum Computing Across Sectors
Quantum computing’s impact will be far-reaching, transforming various sectors. In medicine, it could revolutionize drug discovery and development, enabling the design of new therapies and personalized medicine approaches. Materials science will benefit from the ability to simulate complex molecular interactions, leading to the discovery of novel materials with enhanced properties. Finance will see improvements in risk management, portfolio optimization, and fraud detection.
The development of quantum-resistant cryptography will be essential to secure sensitive data in the face of potential quantum attacks. However, the transition to a quantum-resistant infrastructure will require significant investment and coordination across industries. For example, the pharmaceutical industry could see a drastic reduction in drug development time and costs, while the financial sector could experience more efficient and secure transactions.
Anticipated Advancements in Quantum Computing Technology Within the Next Decade
The next decade is expected to witness significant advancements in quantum computing technology. Improvements in qubit coherence times, scalability, and error correction are anticipated, leading to the development of more powerful and stable quantum computers. Hybrid quantum-classical algorithms will likely become more prevalent, combining the strengths of both classical and quantum approaches. New quantum algorithms and applications will emerge, expanding the range of problems that can be addressed using quantum computing.
Furthermore, we can expect to see increased investment in quantum computing infrastructure, including the development of quantum cloud computing platforms. For example, companies like Google and IBM are already making their quantum computers accessible through cloud platforms, allowing researchers and developers to experiment with quantum algorithms without needing to own and maintain their own quantum hardware. This trend is likely to accelerate, making quantum computing more accessible to a wider range of users and applications.
Closing Summary
The potential of quantum computing applications is vast and transformative. While significant hurdles remain in terms of hardware development and algorithm design, the progress made so far is undeniably exciting. As quantum computers become more powerful and accessible, we can expect to see groundbreaking advancements across diverse sectors, reshaping industries and ultimately, improving the human experience. The journey into the quantum realm is just beginning, and the possibilities are truly limitless.
Query Resolution: Quantum Computing Applications
What is the difference between a qubit and a bit?
A bit, used in classical computers, represents either a 0 or a 1. A qubit, used in quantum computers, can represent a 0, a 1, or a superposition of both simultaneously, allowing for vastly more complex calculations.
When will quantum computers be widely available?
Widespread availability of powerful, fault-tolerant quantum computers is still some years away. While smaller, specialized quantum computers exist, building large-scale, error-corrected machines is a significant technological challenge.
What are the ethical concerns surrounding quantum computing?
Ethical concerns include the potential for misuse in cryptography (breaking existing encryption) and the need for responsible development to prevent exacerbating existing inequalities through unequal access to this powerful technology.
Are quantum computers going to replace classical computers?
Not entirely. Quantum computers excel at specific types of problems, but classical computers will likely remain essential for many everyday tasks. It’s more of a collaboration than a replacement.
How much does a quantum computer cost?
Current quantum computers are extremely expensive, costing millions or even tens of millions of dollars to build and operate. The cost is expected to decrease as the technology matures.