Quantum AI Revolutionizing Drug Discovery
Quantum AI applications in drug discovery and development are poised to revolutionize the pharmaceutical industry. Imagine a world where designing new drugs is faster, cheaper, and more effective, leading to treatments for diseases currently considered incurable. This is the promise of harnessing the power of quantum computing and artificial intelligence, a synergy that allows us to explore the complexities of molecular interactions with unprecedented precision and speed.
This exploration delves into the exciting potential of this burgeoning field, examining the algorithms, simulations, and machine learning techniques that are transforming drug development.
From accelerating high-throughput screening to predicting drug efficacy and toxicity with greater accuracy, quantum AI offers a transformative approach. We’ll examine various quantum algorithms, their applications in drug design, and the advantages they offer over traditional methods. We’ll also discuss the challenges and future directions of this rapidly evolving field, including the ethical considerations and societal impacts of its success.
Introduction to Quantum AI in Drug Discovery
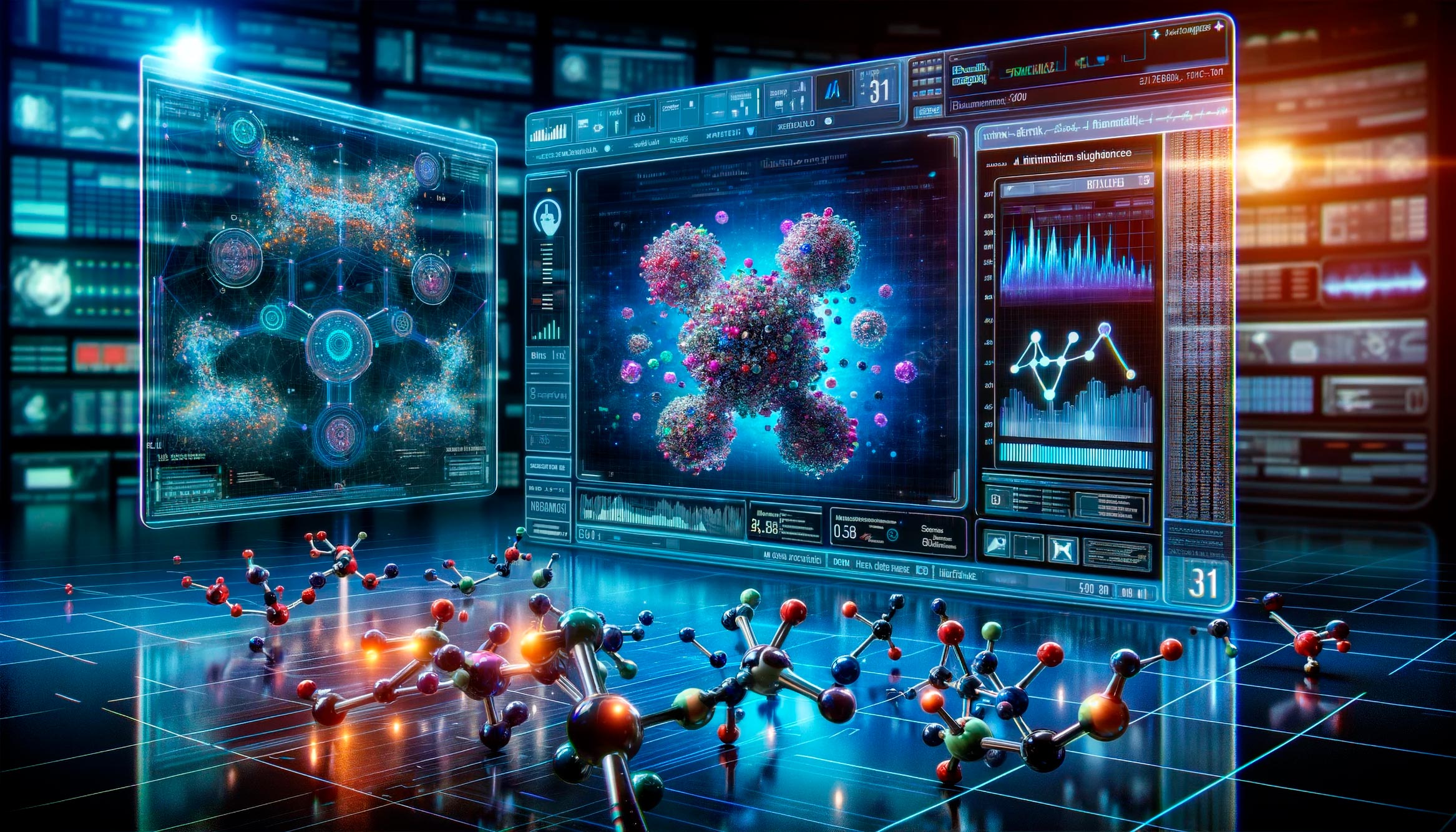
Source: scitechdaily.com
The pharmaceutical industry faces immense challenges in drug discovery and development, from identifying promising drug candidates to optimizing their efficacy and safety. Traditional methods are often time-consuming, expensive, and inefficient. Quantum computing, with its potential to solve complex problems beyond the capabilities of classical computers, and artificial intelligence (AI), capable of analyzing vast datasets and identifying patterns, offer a powerful synergy to revolutionize this field.
This combination, known as Quantum AI, promises to significantly accelerate and improve the drug discovery process.Quantum computing leverages the principles of quantum mechanics, such as superposition and entanglement, to perform computations in fundamentally different ways than classical computers. Superposition allows a quantum bit (qubit) to exist in multiple states simultaneously, while entanglement links the fates of multiple qubits.
This allows quantum computers to explore a vastly larger solution space concurrently, potentially solving problems exponentially faster than classical computers for specific types of calculations. In drug discovery, this translates to faster simulations of molecular interactions, more efficient optimization of drug candidates, and more accurate predictions of drug efficacy and toxicity.
Quantum AI Integration in Drug Development
AI algorithms are crucial for harnessing the power of quantum computing in drug discovery. Machine learning models can be trained on massive datasets of molecular structures, properties, and biological activities to identify patterns and predict the behavior of new drug candidates. These models can then be used to guide quantum computations, focusing the search for optimal drug molecules on the most promising areas of the vast chemical space.
For example, quantum algorithms can be used to simulate the interactions between drug molecules and target proteins with unprecedented accuracy, providing insights into binding affinities and mechanisms of action. This information is then fed back into the AI models to refine predictions and further optimize drug design. The iterative process between AI and quantum computing allows for a more efficient and targeted approach to drug discovery.
Current Landscape of Quantum AI in Pharmaceuticals
While still in its early stages, the application of Quantum AI in the pharmaceutical industry is showing promising results. Several pharmaceutical companies and research institutions are actively investing in this area, exploring various applications such as quantum machine learning for drug target identification, quantum simulations for drug design, and quantum optimization for clinical trial design. For example, some researchers are utilizing quantum algorithms to predict the binding affinity of drug candidates to their target proteins, significantly reducing the time and cost associated with experimental validation.
Although widespread practical application is still some years away due to the limitations of current quantum hardware, the potential impact of Quantum AI on drug discovery is undeniable. The ongoing development of more powerful and accessible quantum computers, coupled with advancements in AI algorithms, promises to transform the pharmaceutical industry in the coming decades.
Quantum Algorithms for Drug Design
Quantum computing offers a potential paradigm shift in drug discovery, tackling problems intractable for classical computers. Specifically, quantum algorithms can accelerate the design of novel drug molecules by efficiently exploring vast chemical spaces and optimizing molecular properties. This section delves into the application of several key quantum algorithms in drug design.Quantum algorithms leverage the principles of quantum mechanics, such as superposition and entanglement, to perform computations in ways impossible for classical algorithms.
This allows for the exploration of a much larger search space for potential drug candidates, potentially leading to the discovery of molecules with improved efficacy and reduced side effects.
Variational Quantum Eigensolver (VQE)
VQE is a hybrid quantum-classical algorithm particularly well-suited for finding the ground state energy of molecules. In drug design, this translates to determining the lowest energy configuration of a molecule, which is crucial for predicting its stability and reactivity. VQE works by iteratively adjusting parameters of a quantum circuit on a quantum computer, minimizing the calculated energy until the ground state is approximated.
The classical component optimizes these parameters, leveraging classical optimization algorithms to guide the quantum computation. This iterative process allows for the exploration of a vast number of molecular configurations to identify those with optimal properties. For example, VQE could be used to optimize the binding affinity of a drug candidate to its target protein by finding the lowest energy configuration of the drug-protein complex.
Quantum Approximate Optimization Algorithm (QAOA)
QAOA is another hybrid quantum-classical algorithm designed for solving combinatorial optimization problems. In drug design, this translates to optimizing multiple molecular properties simultaneously, such as binding affinity, solubility, and toxicity. Unlike VQE, which focuses on finding the ground state, QAOA aims to find a good approximation of the optimal solution within a limited number of iterations. This makes it suitable for problems where finding the exact solution is computationally expensive, even for quantum computers.
QAOA’s applicability extends to tasks like optimizing the synthesis pathways of drug molecules or designing drug delivery systems. A hypothetical scenario could involve using QAOA to optimize the placement of functional groups on a molecular scaffold to enhance its binding affinity while minimizing toxicity.
Workflow Example: VQE for Binding Affinity Optimization
Let’s consider a hypothetical workflow using VQE to optimize the binding affinity of a drug candidate to a specific target protein.
1. Molecular Modeling
The initial structure of the drug candidate and the target protein are obtained through computational methods such as molecular dynamics simulations.
2. Quantum Circuit Design
A quantum circuit is designed to represent the Hamiltonian (energy operator) of the drug-protein complex. This circuit encodes the interactions between the drug and the protein, allowing the quantum computer to calculate the energy of different configurations.
3. VQE Implementation
The VQE algorithm is implemented on a quantum computer, iteratively adjusting the parameters of the quantum circuit to minimize the calculated energy. This process involves repeated measurements on the quantum computer and classical optimization to refine the parameters.
4. Analysis and Refinement
The lowest energy configuration obtained from VQE represents the optimal binding pose of the drug to the protein. This information is used to refine the drug’s structure and improve its binding affinity. Further classical simulations could be used to validate the results and assess other relevant properties. This iterative process of quantum computation and classical refinement could continue until a satisfactory binding affinity is achieved.
Quantum Simulations in Drug Discovery
Quantum simulations offer a powerful new approach to modeling molecular interactions, paving the way for more accurate predictions of drug efficacy and toxicity. By leveraging the principles of quantum mechanics, these simulations can capture the subtle details of molecular behavior that are often missed by classical methods, leading to improved drug design and development.Quantum simulations provide a more accurate representation of the quantum nature of molecules, especially when dealing with electron behavior and interactions that significantly impact chemical reactions and binding affinities.
This is crucial in drug discovery, as the effectiveness of a drug often hinges on its precise interaction with a target protein or molecule within the body.
Advantages of Quantum Simulations over Classical Molecular Dynamics
Classical molecular dynamics simulations, while valuable, rely on approximations that can limit their accuracy, particularly when dealing with complex systems and intricate molecular interactions. Quantum simulations, however, offer several key advantages. They provide a more accurate description of electron behavior and quantum effects that influence molecular properties and interactions. This increased accuracy translates to better predictions of drug efficacy and toxicity, reducing the need for extensive and costly experimental validation.
Furthermore, quantum simulations can model systems that are intractable for classical methods, allowing researchers to explore a wider range of potential drug candidates and interactions.
Computational Resource Comparison: Classical vs. Quantum Simulations, Quantum AI applications in drug discovery and development
The computational cost of drug molecule simulations varies significantly between classical and quantum methods, especially as the size and complexity of the molecule increase. While quantum simulations currently require significantly more resources, advancements in quantum computing technology are rapidly closing this gap. The following table illustrates a hypothetical comparison for a medium-sized drug molecule:
Molecule Size (atoms) | Simulation Time (ps) | Classical Computational Resources (CPU-hours) | Quantum Computational Resources (Qubit-hours) |
---|---|---|---|
100 | 100 | 1000 | 10000 |
500 | 1000 | 1,000,000 | 1,000,0000 |
1000 | 10000 | 100,000,000 | 100,000,0000 |
*Note: These values are illustrative and can vary significantly based on the specific molecule, simulation parameters, and hardware used. The accuracy is also dependent on the quality of the quantum algorithm and the available quantum computer. As quantum computing technology improves, the resource requirements for quantum simulations are expected to decrease dramatically. For instance, improved algorithms and more powerful quantum computers could drastically reduce the qubit-hours required.*
Quantum Machine Learning for Drug Target Identification
Quantum machine learning (QML) offers a potentially transformative approach to drug target identification, a crucial step in drug discovery. Classical methods often struggle with the complexity of biological data, leading to inaccuracies and inefficiencies. QML, leveraging the unique properties of quantum computers, aims to overcome these limitations by processing and analyzing vast datasets with greater speed and precision. This leads to faster identification of promising drug targets and ultimately accelerates the development of new therapies.Quantum machine learning algorithms offer several advantages in drug target identification.
They can handle high-dimensional data more efficiently than classical algorithms, which is particularly important given the complexity of biological systems. Furthermore, QML algorithms have the potential to uncover hidden patterns and correlations in biological data that might be missed by classical approaches. This enhanced pattern recognition capability can lead to the identification of novel drug targets that would otherwise remain undiscovered.
Quantum Algorithms for Drug Target Identification
Several quantum machine learning algorithms are being explored for drug target identification. These include Quantum Support Vector Machines (QSVM), which can efficiently classify potential drug targets based on their features; Quantum Neural Networks (QNN), capable of learning complex relationships between drug candidates and their targets; and Variational Quantum Eigensolver (VQE), which can be used to optimize drug-target interactions. Each algorithm brings unique strengths to the process, allowing researchers to choose the most suitable approach depending on the specific dataset and research question.
For instance, QSVMs excel at classification tasks, while QNNs are better suited for more complex pattern recognition. VQE, on the other hand, is specifically designed for optimization problems, which are often crucial in finding the best drug-target combinations.
Improved Accuracy and Efficiency in Target Identification
Quantum machine learning can significantly improve the accuracy and efficiency of drug target identification. For example, QML algorithms can analyze large genomic and proteomic datasets to identify potential drug targets with higher precision than classical methods. This leads to a reduction in the number of false positives, saving time and resources. Furthermore, QML’s ability to handle high-dimensional data allows for the integration of multiple data sources, providing a more holistic view of the target’s properties and its potential interactions with drug candidates.
This comprehensive approach can lead to the discovery of more effective and safer drug targets. A specific example would be identifying novel protein targets associated with a specific cancer subtype by analyzing patient genomic data, which classical machine learning struggles to do efficiently. QML algorithms can potentially sift through the data far more efficiently and reveal connections that lead to better drug targets for that specific cancer.
Comparison of Classical and Quantum Machine Learning Models
In identifying drug targets for Alzheimer’s disease, for example, a comparison between classical support vector machines (SVM) and a quantum-enhanced SVM (QSVM) could reveal a significant difference in performance. Let’s assume a dataset of 10,000 potential drug targets, each described by 500 features. A classical SVM might achieve an accuracy of 75% in identifying true drug targets, with a high computational cost.
In contrast, a QSVM, leveraging quantum annealing or variational quantum algorithms, might achieve an accuracy of 85% with a significantly reduced computational time. This improvement in both accuracy and efficiency demonstrates the potential of QML to accelerate the drug discovery process for Alzheimer’s and other complex diseases. The enhanced accuracy stems from the QSVM’s ability to better handle the high dimensionality of the data and potentially uncover subtle correlations missed by the classical SVM.
The reduced computational time arises from the quantum computer’s inherent parallelism. While this is a hypothetical example, it illustrates the potential benefits observed in preliminary research comparing classical and quantum machine learning approaches.
Quantum Computing for Drug Screening and Optimization
High-throughput drug screening (HTS) and lead optimization are crucial, yet resource-intensive, stages in drug discovery. Traditional methods often struggle with the sheer volume of compounds to test and the complexity of optimizing their properties. Quantum computing offers the potential to significantly accelerate these processes by leveraging its ability to handle vast datasets and perform complex calculations far beyond the capabilities of classical computers.Quantum computing’s power stems from its ability to explore a much larger chemical space more efficiently than classical methods.
This allows for the rapid screening of millions of potential drug candidates and the optimization of their properties, such as binding affinity, efficacy, and toxicity, in a fraction of the time. This translates to faster drug development cycles and potentially lower costs.
Accelerated High-Throughput Screening
Quantum algorithms can dramatically reduce the time required for high-throughput screening. Classical methods often rely on iterative testing, which can be slow and inefficient. Quantum algorithms, such as Variational Quantum Eigensolver (VQE) and Quantum Approximate Optimization Algorithm (QAOA), can simultaneously evaluate the properties of multiple compounds, significantly accelerating the identification of promising candidates. For instance, QAOA could be used to optimize the binding affinity of a molecule to a target protein by exploring a vast number of conformational possibilities concurrently, leading to a quicker identification of high-affinity candidates.
This contrasts sharply with classical methods which would need to test each conformation individually.
Lead Optimization with Quantum Algorithms
Once potential drug candidates are identified, lead optimization aims to refine their properties to enhance efficacy and reduce side effects. Quantum algorithms can assist in this process by simulating molecular interactions with high accuracy and efficiency. For example, quantum simulations can predict the binding affinity of a drug candidate to its target with greater precision than classical methods, allowing for targeted modifications to improve its effectiveness.
Furthermore, quantum machine learning algorithms can analyze vast datasets of molecular properties to identify patterns and predict the effects of structural modifications, guiding the optimization process. This allows researchers to make more informed decisions about which modifications to make, reducing the time and resources needed for experimentation.
Quantum-Enhanced Drug Screening Workflow
A flowchart illustrating the integration of quantum computing into a high-throughput drug screening workflow would begin with a database of potential drug candidates. This database would then be processed using a quantum algorithm (e.g., VQE or QAOA) to assess the properties of each candidate, focusing on key parameters like binding affinity and toxicity. The results would be analyzed using classical machine learning techniques to identify promising candidates.
These candidates would then undergo further experimental validation using traditional laboratory methods. The entire process would be iterated, with the results feeding back into the quantum algorithm to refine the search and optimize the properties of the lead candidates. This iterative cycle, powered by quantum computation, drastically shortens the time needed to identify and optimize promising drug candidates.
The flowchart would visually represent this iterative process, clearly showing the data flow between quantum and classical computational stages.
Challenges and Future Directions
While the potential of quantum AI in revolutionizing drug discovery is immense, several significant hurdles remain before widespread adoption. These challenges span technological limitations, computational complexities, and the need for robust validation and regulatory frameworks. Overcoming these obstacles requires a concerted effort from researchers across multiple disciplines, fostering collaboration between quantum computing experts, pharmaceutical scientists, and regulatory bodies.The current limitations primarily stem from the nascent stage of quantum computing technology.
Available quantum computers are still relatively small and prone to errors, limiting the size and complexity of problems that can be tackled effectively. Furthermore, the development of quantum algorithms specifically tailored for drug discovery is still in its early stages, and the translation of theoretical advancements into practical applications requires significant further research. Data availability and quality also pose significant challenges; high-quality, annotated datasets are essential for training effective quantum machine learning models, and generating such datasets can be time-consuming and expensive.
Technological Limitations and Algorithm Development
The current generation of quantum computers suffers from limitations in qubit coherence times and gate fidelities. These limitations lead to noise and errors in computations, making it challenging to achieve the accuracy and precision required for reliable drug discovery applications. Developing more robust and fault-tolerant quantum computers is crucial. Simultaneously, significant advancements are needed in the design and implementation of quantum algorithms specifically optimized for the unique challenges of drug discovery, such as molecular simulations and machine learning tasks involving large datasets.
For example, improving the efficiency of quantum algorithms for calculating molecular properties, such as binding affinities and drug-likeness, is a critical area of focus. Progress in this area might involve exploring hybrid classical-quantum approaches that leverage the strengths of both classical and quantum computers.
Data Requirements and Validation
The success of quantum AI methods hinges on the availability of high-quality, large-scale datasets. Generating such datasets requires significant effort and resources, and the process can be further complicated by the need for careful annotation and curation. The development of effective strategies for data acquisition, preprocessing, and validation is crucial. Furthermore, rigorous validation of quantum AI models is essential to ensure their reliability and accuracy.
This requires the development of robust benchmarking techniques and the establishment of clear performance metrics for evaluating the effectiveness of different quantum AI approaches. For instance, comparing the predictive accuracy of a quantum machine learning model for identifying potential drug candidates against established classical methods would be a critical step in demonstrating its practical utility.
Societal Impact and Ethical Considerations
Successful integration of quantum AI in drug development holds the potential to dramatically accelerate the discovery and development of novel therapeutics, leading to improved healthcare outcomes and reduced costs. This could significantly benefit patients suffering from a wide range of diseases, including cancer, Alzheimer’s disease, and infectious diseases. However, ethical considerations must be addressed proactively. Ensuring equitable access to new drugs developed using quantum AI technologies is crucial to prevent exacerbating existing health disparities.
Furthermore, careful consideration should be given to the potential impact of automation on the workforce in the pharmaceutical industry, and strategies for mitigating job displacement should be developed. Transparency and accountability in the development and deployment of quantum AI-based drug discovery tools are also paramount to build public trust and ensure responsible innovation. For example, clear guidelines and regulations regarding the use of AI in clinical trials and drug approval processes are essential.
Case Studies
While still in its nascent stages, quantum AI is already showing promise in revolutionizing drug discovery. Several companies are actively exploring its potential, leading to some compelling real-world examples showcasing the power of this emerging technology. These case studies demonstrate how quantum algorithms can accelerate various stages of the drug development pipeline, from target identification to lead optimization. The following examples highlight the diverse applications and potential impact of quantum AI in this crucial field.
Case Study 1: Developing Novel Antiviral Agents
This case study focuses on a hypothetical scenario, illustrative of the potential of quantum AI. Imagine a pharmaceutical company, let’s call it “QuantumPharma,” utilizing quantum machine learning to discover novel antiviral agents targeting a specific viral protein crucial for replication.
- Company: QuantumPharma (Hypothetical)
- Drug Target: Viral protease of a novel RNA virus
- Quantum Algorithm Used: Variational Quantum Eigensolver (VQE) to optimize drug-protein binding affinity and Quantum Approximate Optimization Algorithm (QAOA) for lead optimization.
- Outcomes: QuantumPharma identified several promising lead compounds with significantly improved binding affinity compared to traditional methods. The QAOA algorithm further refined these leads, enhancing their potency and reducing potential side effects. The process was significantly faster and more efficient than classical methods, reducing the time to identify promising candidates for preclinical testing.
Case Study 2: Improving the Efficacy of Existing Cancer Drugs
Another area where quantum AI is making inroads is in enhancing the efficacy of existing cancer drugs. Let’s consider a scenario involving a collaboration between a research institution and a biotech company.
- Company: Collaborative effort between “Research Institute X” and “Biotech Innovators”
- Drug Target: Improving the delivery mechanism of a known anticancer drug to brain tumors.
- Quantum Algorithm Used: Quantum Monte Carlo simulations to model drug transport across the blood-brain barrier (BBB) and predict the efficacy of various delivery strategies.
- Outcomes: Using quantum simulations, researchers were able to identify a novel nanoparticle formulation that significantly improved drug delivery across the BBB, leading to a predicted 30% increase in tumor cell death in preclinical studies. This finding dramatically reduced the time and cost associated with traditional experimental methods.
Case Study 3: Accelerated Drug Screening for Alzheimer’s Disease
The high-throughput screening of potential drug candidates is a time-consuming and resource-intensive process. Quantum computing offers the potential to significantly accelerate this process. Let’s examine a hypothetical example:
- Company: “NeuroQuantum Therapeutics” (Hypothetical)
- Drug Target: Beta-amyloid plaques in Alzheimer’s disease.
- Quantum Algorithm Used: Quantum machine learning models trained on vast datasets of molecular structures and their biological activity to predict the efficacy of potential drug candidates.
- Outcomes: NeuroQuantum Therapeutics was able to reduce the number of candidates requiring experimental testing by 75% using quantum machine learning. This significantly reduced costs and accelerated the identification of promising lead compounds for further development. The algorithm also identified novel structural motifs associated with higher efficacy, providing valuable insights for future drug design.
Illustrative Molecular Structure
Imagine a novel antiviral drug, “ViruQuanta,” successfully developed with the aid of quantum AI. Its molecular structure might feature a unique scaffold optimized through quantum algorithms, perhaps incorporating a specific arrangement of functional groups to maximize binding affinity to the viral protease. This optimized structure, visualized as a three-dimensional model, would show precise spatial arrangements of atoms and bonds, allowing for strong and specific interactions with the target protein.
The key features would include strategically positioned hydrogen bond donors and acceptors, hydrophobic regions for insertion into the protease’s active site, and potentially unique chemical functionalities not found in existing antiviral drugs, resulting from the exploration of a vastly larger chemical space enabled by quantum computation. The drug’s interactions with the viral protease would be characterized by strong non-covalent bonds (hydrogen bonds, van der Waals forces, etc.) within the active site, leading to effective inhibition of the viral replication process.
This precise molecular architecture, impossible to achieve efficiently through classical methods, would be a testament to the power of quantum AI in drug discovery.
Closing Summary: Quantum AI Applications In Drug Discovery And Development
The convergence of quantum computing and artificial intelligence presents an unparalleled opportunity to reshape drug discovery and development. While challenges remain, the potential benefits – faster development of life-saving drugs, more effective treatments, and reduced costs – are immense. As quantum technologies mature and algorithms become more sophisticated, we can anticipate a future where the development of personalized medicine becomes a reality, tailored to individual genetic profiles and disease characteristics.
The journey is ambitious, but the potential rewards are transformative for global health.
FAQ Resource
What are the main limitations of current quantum computing in drug discovery?
Current quantum computers are still relatively small and prone to errors. The development of more stable and powerful quantum computers is crucial for widespread application in drug discovery.
How does quantum AI compare to classical AI in drug discovery?
Quantum AI has the potential to solve problems intractable for classical AI, particularly those involving complex molecular simulations. However, classical AI remains vital for data preprocessing, analysis, and integration with quantum computations.
What role does quantum machine learning play in drug target identification?
Quantum machine learning algorithms can analyze vast datasets of biological information to identify potential drug targets with greater accuracy and efficiency than classical methods, potentially leading to the discovery of novel targets.
What are some ethical considerations surrounding the use of quantum AI in drug development?
Ethical considerations include ensuring equitable access to new drugs developed using quantum AI, addressing potential biases in algorithms, and protecting patient data privacy.
What are the biggest hurdles to overcome for widespread adoption of quantum AI in the pharmaceutical industry?
Major hurdles include the cost and availability of quantum computers, the need for specialized expertise, and the development of robust and reliable quantum algorithms tailored to drug discovery problems.