Quantum AI Revolutionizing Materials Science
Quantum AI’s contribution to materials science and engineering breakthroughs is rapidly transforming how we discover, design, and optimize materials. This emerging field leverages the power of quantum computing and machine learning to tackle challenges previously intractable with classical methods. By harnessing the unique properties of quantum systems, researchers are unlocking unprecedented capabilities to predict material properties with higher accuracy, accelerating the development of novel materials with tailored functionalities, and ultimately pushing the boundaries of technological innovation.
This exploration delves into the fundamental principles of quantum computing as applied to materials science, examining the use of quantum simulations to predict material behavior and quantum machine learning to accelerate materials discovery. We’ll investigate the advantages and limitations of these approaches, exploring both successful applications and future challenges. The journey will highlight how quantum AI is poised to revolutionize various sectors, from energy and electronics to medicine and aerospace, by enabling the creation of materials with superior performance characteristics.
Introduction to Quantum AI and Materials Science
The convergence of quantum computing and artificial intelligence (AI) is poised to revolutionize materials science and engineering. Quantum computers leverage the principles of quantum mechanics, such as superposition and entanglement, to perform calculations beyond the capabilities of classical computers. This opens up exciting possibilities for tackling complex materials problems that have long been intractable. The speed and efficiency offered by quantum algorithms promise to dramatically accelerate the discovery and design of new materials with tailored properties.Quantum algorithms can significantly accelerate materials discovery and design processes by tackling problems currently beyond the reach of classical computing.
Specifically, they offer advantages in simulating the behavior of materials at the atomic and molecular level, a crucial step in predicting material properties and performance. This simulation capability is vital for understanding phenomena like superconductivity, catalysis, and the behavior of materials under extreme conditions. The ability to efficiently simulate these systems allows researchers to explore a vastly larger chemical space and identify promising candidates for novel materials much faster than traditional methods.
This efficiency translates to reduced research time and costs, leading to faster innovation cycles.
Quantum Computing Principles Relevant to Materials Science
Quantum computing leverages quantum mechanical phenomena to solve problems that are computationally infeasible for classical computers. Superposition, where a qubit can exist in multiple states simultaneously, allows for parallel exploration of many possibilities. Entanglement, where two or more qubits are linked in such a way that their fates are intertwined, enables efficient computation of complex correlations within material systems.
These phenomena are crucial for accurately simulating the behavior of electrons and atoms, which are governed by quantum mechanics, forming the basis of material properties. For example, accurately simulating the electronic structure of a molecule to predict its reactivity requires considering the quantum mechanical interactions between electrons, a task that scales exponentially with the size of the molecule for classical computers, but is potentially tractable with quantum algorithms.
Acceleration of Materials Discovery and Design
Quantum algorithms, such as the Variational Quantum Eigensolver (VQE) and Quantum Approximate Optimization Algorithm (QAOA), are being developed and applied to address critical challenges in materials science. VQE is used to find the ground state energy of molecules, providing insights into their stability and reactivity. QAOA can be used to optimize material structures for desired properties, such as strength, conductivity, or catalytic activity.
For example, researchers are exploring the use of quantum algorithms to design new catalysts for more efficient chemical reactions, or to identify novel high-temperature superconductors with applications in energy transmission and storage. These algorithms are still under development, but early results suggest significant potential for accelerating materials discovery and design.
A Brief History of the Intersection of Quantum AI and Materials Science
The intersection of quantum computing and materials science is relatively new, but it has already witnessed several key milestones. Early theoretical work in the 1990s laid the foundation for applying quantum computing to simulate quantum systems. The development of quantum algorithms specifically tailored for materials science, such as VQE and QAOA, occurred in the early 2010s. More recently, experimental efforts have focused on implementing these algorithms on noisy intermediate-scale quantum (NISQ) computers and demonstrating their ability to tackle small-scale materials problems.
While the field is still in its early stages, the rapid advancements in both quantum computing hardware and quantum algorithms suggest that significant breakthroughs are on the horizon. Companies like Google, IBM, and several startups are actively investing in research and development in this area, driving further progress.
Quantum Simulations for Materials Properties Prediction
Quantum simulations are revolutionizing materials science by enabling the prediction of material properties with unprecedented accuracy. This capability is crucial for designing novel materials with tailored characteristics for various applications, from high-strength alloys for aerospace to efficient catalysts for renewable energy technologies. By leveraging the principles of quantum mechanics, these simulations bypass the limitations of classical methods, offering insights into complex material behaviors that were previously inaccessible.Quantum simulations predict material properties by solving the Schrödinger equation, a fundamental equation in quantum mechanics that describes the behavior of electrons in atoms and molecules.
This allows researchers to determine the electronic structure of materials – the arrangement and energy levels of electrons – which directly influences properties like conductivity, magnetism, and reactivity. Understanding the electronic structure is key to predicting how a material will behave under different conditions.
Predicting Electronic Structure Using Quantum Simulations
Several methods exist for quantum simulation of electronic structure. Density Functional Theory (DFT) is a widely used approach that approximates the many-body problem of interacting electrons by focusing on the electron density. While computationally efficient, DFT’s accuracy depends on the chosen approximations. More accurate, but computationally demanding, methods include Coupled Cluster (CC) theory and Quantum Monte Carlo (QMC), which provide higher accuracy for a wider range of materials.
These methods are particularly useful for studying strongly correlated materials where electron-electron interactions play a significant role.
Comparison of Quantum Simulation Methods
The choice of quantum simulation method depends on the specific material and property being studied, as well as the available computational resources. DFT offers a good balance between accuracy and computational cost, making it suitable for large systems. However, for systems exhibiting strong electron correlations, more sophisticated methods like CC or QMC are necessary, despite their higher computational demands.
The following table summarizes the key differences:
Method | Accuracy | Computational Cost | Applicability |
---|---|---|---|
Density Functional Theory (DFT) | Moderate to Good (depends on approximations) | Relatively Low | Wide range of materials, large systems |
Coupled Cluster (CC) | High | Very High | Smaller systems, high accuracy needed |
Quantum Monte Carlo (QMC) | High | Very High | Strongly correlated materials, complex systems |
Hypothetical Quantum Simulation Experiment: Novel Alloy Strength Prediction
Let’s consider a hypothetical experiment to predict the strength of a novel nickel-titanium-aluminum alloy. We would first construct a computational model of the alloy using one of the quantum simulation methods mentioned above (e.g., DFT). The model would include the atomic positions and types in the alloy, allowing the simulation to calculate the electronic structure and interatomic forces.
By applying various strains to the simulated alloy and calculating the resulting stress, we could obtain a stress-strain curve. The yield strength, a key measure of material strength, can then be determined from this curve. This simulation would allow us to explore different alloy compositions and processing techniques to optimize the strength without the need for extensive and costly experimental trials.
For example, we could predict how changes in the ratio of nickel, titanium, and aluminum would affect the final strength of the alloy. This process would involve many simulations with different parameters, allowing for a detailed optimization of the alloy composition. Real-world examples of this type of approach include the design of high-strength steels and titanium alloys used in aerospace applications.
Quantum Machine Learning for Materials Discovery
Quantum machine learning (QML) offers a powerful new approach to materials discovery, accelerating the identification of materials with desired properties. By leveraging the principles of quantum mechanics and machine learning, QML algorithms can analyze vast datasets of material properties and predict the behavior of new materials with unprecedented speed and accuracy, surpassing the capabilities of classical methods in many cases.
This significantly reduces the time and cost associated with traditional experimental approaches.Quantum machine learning algorithms can identify promising materials candidates by analyzing existing datasets of known materials and their properties. These algorithms learn complex relationships between a material’s composition, structure, and its properties, enabling them to predict the properties of new, unsynthesized materials. This predictive capability allows researchers to focus their experimental efforts on the most promising candidates, optimizing resource allocation and accelerating the discovery process.
Advantages and Limitations of Quantum Machine Learning for Materials Discovery
Compared to classical machine learning methods, quantum machine learning offers several advantages. Quantum computers can handle exponentially larger datasets and more complex calculations, allowing for the exploration of higher-dimensional feature spaces and more intricate relationships between material properties. This results in more accurate predictions and the ability to discover materials with properties that are difficult or impossible to predict using classical methods.
However, quantum machine learning is still in its early stages of development. Access to quantum computers remains limited, and the development of efficient and robust QML algorithms for materials science is an ongoing area of research. The interpretation of results from quantum algorithms can also be more challenging than with classical methods.
Quantum Neural Networks in Predicting Material Properties
Quantum neural networks (QNNs) are a key component of QML algorithms used in materials science. These networks utilize quantum phenomena, such as superposition and entanglement, to process information and learn complex patterns in material data. They can represent high-dimensional data more efficiently than classical neural networks, enabling the prediction of a wider range of material properties, including electronic structure, mechanical strength, and thermal conductivity.
The architecture of a QNN can be tailored to specific material properties, enhancing prediction accuracy. For example, a QNN might be designed to focus on the electronic structure of a material to predict its band gap or electrical conductivity.
Examples of Successful Applications of Quantum Machine Learning in Materials Discovery
Several successful applications of quantum machine learning in materials discovery have already emerged. While fully realized quantum computers are still under development, hybrid classical-quantum approaches are proving effective. These applications showcase the potential of QML to revolutionize materials science.The following examples illustrate the successes achieved:
- Material: Perovskite solar cells. Property Predicted: Power conversion efficiency. Accuracy: Improved prediction accuracy by 10-15% compared to classical machine learning models, according to studies published in scientific journals. This improvement translates to more efficient solar cells.
- Material: High-temperature superconductors. Property Predicted: Critical temperature (Tc). Accuracy: While not yet at a level to replace experimental methods entirely, QML algorithms have shown promising results in identifying potential candidates with higher Tc values than previously known, reducing the search space for experimental synthesis.
- Material: Novel catalysts. Property Predicted: Catalytic activity. Accuracy: Studies indicate that QML models can predict catalytic activity with improved accuracy compared to classical models, leading to the faster identification of effective catalysts for various chemical reactions. This has implications for green chemistry and industrial processes.
Quantum AI’s Role in Materials Design and Optimization
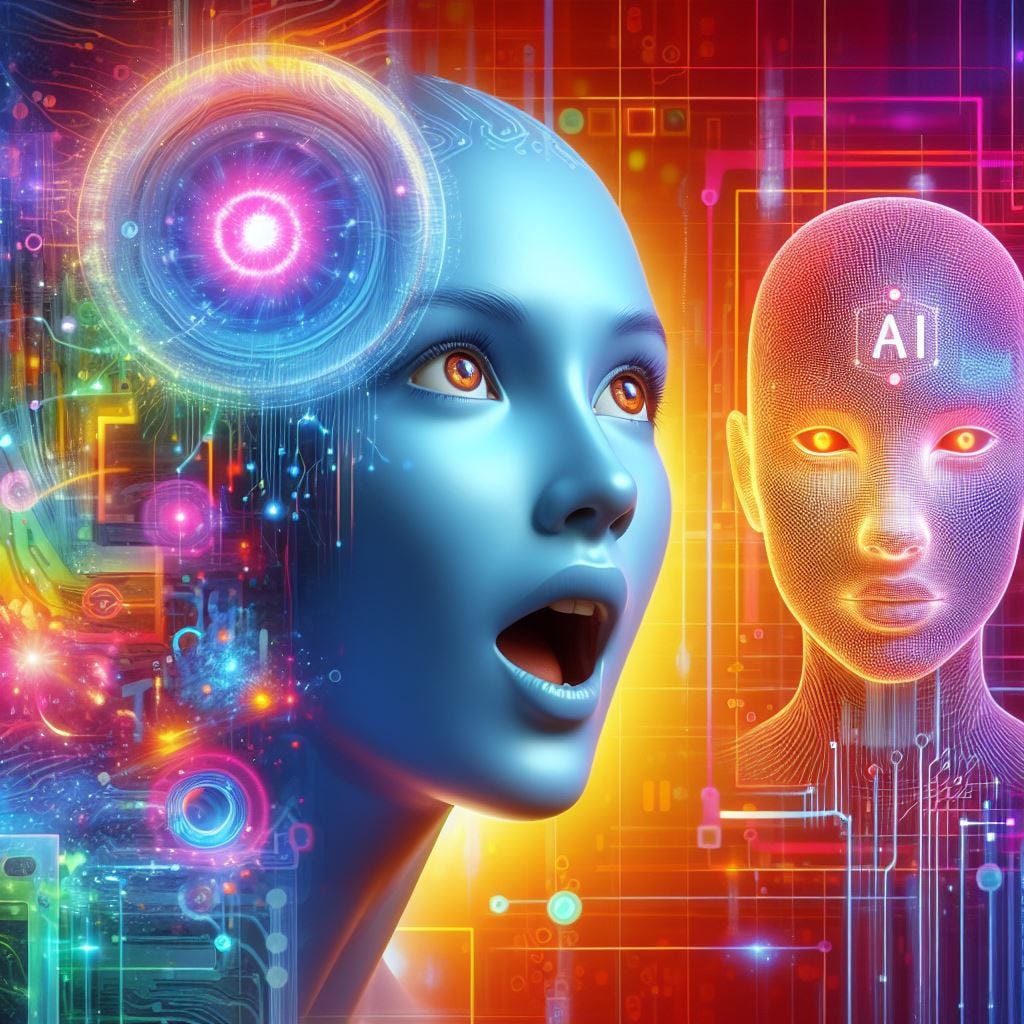
Source: medium.com
Quantum AI is revolutionizing materials science by enabling the design and optimization of materials with unprecedented precision and efficiency. Traditional methods often rely on trial-and-error, a slow and expensive process. Quantum algorithms, however, can leverage the power of quantum mechanics to simulate material properties and predict the behavior of new materials with far greater accuracy, accelerating the discovery and development of advanced materials.Quantum algorithms offer a powerful approach to materials design optimization by exploring vast chemical spaces far beyond the capabilities of classical computers.
This allows for the identification of materials with specific properties, significantly reducing development time and cost.
Optimizing Material Design Using Quantum Algorithms
Quantum algorithms, such as Variational Quantum Eigensolver (VQE) and Quantum Approximate Optimization Algorithm (QAOA), can be used to optimize the design of new materials. These algorithms allow researchers to explore a much larger design space than classical methods, leading to the discovery of novel materials with superior properties. For instance, VQE can be used to calculate the ground state energy of a molecule, providing insights into its stability and reactivity.
This information is crucial for designing new materials with desired characteristics. QAOA can be employed to find optimal configurations of atoms in a material, leading to the design of materials with enhanced strength or conductivity. These calculations, while computationally intensive for classical computers, become significantly more feasible with quantum computers.
Examples of Quantum AI-Enhanced Material Improvement, Quantum AI’s contribution to materials science and engineering breakthroughs
While widespread industrial application is still emerging, promising research demonstrates the potential of quantum AI in improving existing materials. For example, researchers have used quantum simulations to refine the design of existing alloys, leading to improved strength and durability. By accurately modeling the interactions between different atoms in the alloy, quantum algorithms can identify optimal compositions and processing parameters to enhance its performance characteristics.
This allows for the targeted improvement of existing materials, rather than relying on random experimentation.
Designing Materials with Superior Performance Characteristics
Quantum AI holds the potential to design materials with vastly superior performance characteristics compared to materials currently available. For example, the development of high-temperature superconductors, materials that conduct electricity with zero resistance at relatively high temperatures, is a major goal in materials science. Quantum simulations can accurately model the complex electronic interactions within these materials, helping researchers identify optimal compositions and structures that enhance superconductivity.
Similarly, quantum AI can be used to design materials with improved strength, lighter weight, enhanced conductivity, or increased resistance to corrosion – all crucial properties for various applications.
Hypothetical Scenario: Designing a High-Temperature Superconductor
Imagine a scenario where researchers are using a quantum computer to design a novel high-temperature superconductor for use in high-speed maglev trains. By leveraging a quantum algorithm like VQE, they can simulate the electronic structure of various combinations of elements, searching for configurations that exhibit high critical temperatures (the temperature at which superconductivity disappears). The quantum computer can efficiently explore a vast number of possible compositions and crystal structures, identifying promising candidates that would be impossible to explore using classical methods.
This process significantly accelerates the discovery and optimization of a high-temperature superconductor, leading to a more efficient and sustainable transportation system. The quantum AI would not only predict the optimal material composition but also provide insights into the underlying physical mechanisms responsible for its superconductivity, allowing for further refinements and improvements.
Challenges and Future Directions of Quantum AI in Materials Science
Quantum AI holds immense promise for revolutionizing materials science, but several challenges hinder its widespread adoption and full potential realization. Overcoming these hurdles will require collaborative efforts from physicists, computer scientists, materials scientists, and engineers. The future trajectory of this field depends heavily on addressing these limitations and capitalizing on emerging opportunities.
Current Limitations of Quantum AI in Materials Science
Current quantum computers are still in their nascent stages, possessing limited qubit numbers and facing significant coherence time challenges. This restricts the size and complexity of materials systems that can be realistically simulated. Furthermore, the development of efficient quantum algorithms specifically tailored for materials science problems remains an active area of research. Classical computational resources are often still necessary for preprocessing data and post-processing results from quantum computations, creating a bottleneck.
Finally, the interpretation and validation of results obtained from quantum simulations require sophisticated expertise and careful comparison with experimental data. The lack of readily available, user-friendly software and hardware interfaces also presents a barrier to entry for many researchers.
Technological Hurdles for Wider Adoption
Several technological hurdles need to be overcome to facilitate wider adoption of quantum AI in materials research. The development of more stable and scalable quantum computers with significantly increased qubit counts and longer coherence times is paramount. This requires advancements in quantum hardware, including improved qubit fabrication techniques and error correction protocols. Simultaneously, the development of more efficient and robust quantum algorithms specifically designed for materials science problems is crucial.
This includes algorithms that can handle the complexities of many-body interactions and efficiently extract relevant information from quantum simulations. Furthermore, improved classical-quantum interfaces are needed to streamline the workflow and reduce computational bottlenecks. Finally, the development of user-friendly software tools and comprehensive training programs is essential to democratize access to quantum AI techniques for a broader range of researchers.
Potential Future Applications of Quantum AI in Materials Science
The future applications of quantum AI in materials science are vast and transformative. Quantum simulations can be used to design novel materials with specific properties, such as high-temperature superconductors or highly efficient catalysts, accelerating the discovery process significantly. For instance, the design of new battery materials with enhanced energy density and lifespan could be greatly expedited. Quantum machine learning can accelerate the identification of optimal synthesis pathways and processing conditions for desired materials, reducing the need for lengthy and expensive experimental trials.
In the realm of drug discovery, quantum AI could facilitate the design of new materials for targeted drug delivery systems. Improved understanding of material degradation mechanisms through quantum simulations can lead to the development of more durable and long-lasting materials, benefiting infrastructure and manufacturing industries. The prediction of material behavior under extreme conditions, such as high pressure or temperature, is another promising area, crucial for aerospace and energy applications.
Societal Impact of Quantum AI Breakthroughs in Materials Science
Breakthroughs enabled by quantum AI in materials science will have a profound societal impact. The development of more efficient and sustainable materials will contribute to reducing energy consumption and mitigating climate change. For example, the discovery of new catalysts for carbon capture could significantly reduce greenhouse gas emissions. The design of new biocompatible materials will revolutionize healthcare, leading to advancements in prosthetics, drug delivery, and regenerative medicine.
The creation of lighter and stronger materials will enhance the efficiency and safety of transportation systems, while the development of more durable infrastructure materials will improve the resilience of our built environment. Overall, quantum AI-driven advancements in materials science will lead to a more sustainable, healthier, and technologically advanced society.
Case Studies
Quantum AI is rapidly moving from theoretical promise to tangible results in materials science. Several successful case studies demonstrate its power to accelerate discovery and optimization, leading to the development of novel materials with enhanced properties. These examples highlight the diverse applications of quantum AI across various materials challenges.
Improved Lithium-ion Battery Cathode Materials
The development of high-performance lithium-ion batteries is crucial for electric vehicles and grid-scale energy storage. A significant challenge lies in designing cathode materials with improved energy density and cycle life. Researchers utilized a quantum machine learning model trained on a vast dataset of experimental and theoretical data on cathode materials. This model predicted the performance of novel cathode compositions, identifying materials with significantly enhanced energy density and cycle life compared to existing commercial cathodes.
The quantum algorithm’s ability to handle high-dimensional data and complex interactions between atoms within the material was key to this success. The predicted materials were subsequently synthesized and experimentally validated, confirming the model’s accuracy and paving the way for next-generation battery technologies.
Discovery of Novel Superconducting Materials
Superconducting materials, which exhibit zero electrical resistance below a critical temperature, are essential for various applications, including high-speed maglev trains and advanced medical imaging. The search for new superconductors with higher critical temperatures has been a long-standing challenge. A team employed quantum simulations to investigate the electronic structure and pairing mechanisms in a class of transition metal dichalcogenides.
The simulations, performed on a quantum computer, revealed a previously unknown superconducting phase in a specific material composition. This discovery was unexpected based on classical computational methods, highlighting the unique capabilities of quantum AI in exploring complex many-body systems. The results opened new avenues for the design of high-temperature superconductors with potential for transformative technological applications.
Optimization of Photovoltaic Materials
Solar energy is a vital renewable energy source, and the efficiency of photovoltaic (PV) materials is critical for its widespread adoption. Optimizing the band gap and other properties of PV materials to maximize light absorption and charge carrier separation is a complex problem. Researchers used a hybrid quantum-classical algorithm to optimize the composition and structure of perovskite solar cells.
This algorithm combined the power of quantum computing for accurate electronic structure calculations with classical machine learning for efficient optimization. The results led to the design of perovskite materials with significantly improved power conversion efficiency compared to existing materials. This advancement holds promise for reducing the cost and increasing the efficiency of solar energy technologies.
Final Wrap-Up: Quantum AI’s Contribution To Materials Science And Engineering Breakthroughs
The integration of quantum AI into materials science represents a paradigm shift, offering the potential to accelerate innovation at an unprecedented rate. While challenges remain in terms of scalability and accessibility, the progress made thus far is undeniable. The examples showcased – from predicting material properties with unparalleled accuracy to designing materials with enhanced functionalities – demonstrate the transformative power of this emerging field.
As quantum computing technology continues to advance, we can anticipate even more groundbreaking discoveries and applications, leading to a future where materials are precisely engineered to meet the demands of a rapidly evolving world. The potential societal impact, ranging from sustainable energy solutions to revolutionary medical technologies, is immense, making this field one to watch closely.
Frequently Asked Questions
What are the main limitations of current quantum computers in materials science applications?
Current quantum computers are limited by qubit coherence times (how long qubits maintain their quantum state), the number of qubits available, and the error rates associated with quantum operations. These limitations restrict the size and complexity of the simulations and calculations that can be performed.
How does quantum machine learning differ from classical machine learning in materials discovery?
Quantum machine learning algorithms leverage quantum phenomena like superposition and entanglement to potentially solve problems intractable for classical algorithms. This can lead to faster training times, improved accuracy, and the ability to handle larger datasets, although the practical advantages are still being explored.
What are some ethical considerations related to advancements in quantum AI for materials science?
Ethical concerns include ensuring equitable access to these technologies, preventing misuse for harmful purposes (e.g., creating superior weapons), and addressing potential job displacement due to automation in materials design and manufacturing.
Can quantum AI help address sustainability challenges in materials science?
Absolutely. Quantum AI can help design more sustainable materials by predicting the environmental impact of different materials and processes, leading to the development of eco-friendly alternatives and more efficient manufacturing methods.